4 lessons healthcare can teach us about successful applications of AI
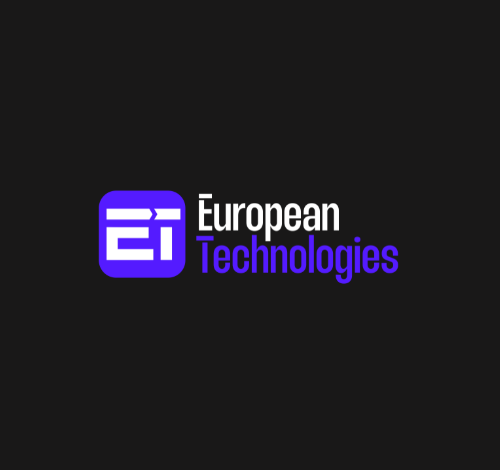
In order to get the most out of a chatbot and meet regulatory requirements, healthcare users must find solutions that enable them to shift noisy clinical data to a natural language interface that can answer questions automatically. At scale, and with full privacy, to boot. Since this cannot be achieved by simply applying LLM or RAG LLM solutions, it starts with a healthcare-specific data pre-processing pipeline. Other high-compliance industries like law and finance can take a page from healthcare’s book by preparing their data privately, at scale, on commodity hardware, using other models to query it.
Democratizing generative AI
AI is only as useful as the data scientists and IT professionals behind enterprise-grade use cases—until now. No-code solutions are emerging, specifically designed for the most common healthcare use cases. The most notable being, using LLMs to bootstrap task-specific models. Essentially, this enables domain experts to start with a set of prompts and provide feedback to improve accuracy beyond what prompt engineering can provide. The LLMs can then train small, fine-tuned models for that specific task.
This approach gets AI into the hands of domain experts, results in higher-accuracy models than what LLMs can deliver on their own, and can be run cheaply at scale. This is particularly useful for high-compliance enterprises, given no data sharing is required and zero-shot prompts and LLMs can be deployed behind an organization’s firewall. A full range of security controls, including role-based access, data versioning, and full audit trails, can be built in, and make it simple for even novice AI users to keep track of changes, as well as continue to improve models over time.
Addressing challenges and ethical considerations
Ensuring the reliability and explainability of AI-generated outputs is crucial to maintaining patient safety and trust in the healthcare system. Moreover, addressing inherent biases is essential for equitable access to AI-driven healthcare solutions for all patient populations. Collaborative efforts between clinicians, data scientists, ethicists, and regulatory bodies are necessary to establish guidelines for the responsible deployment of AI in healthcare and beyond.
It’s for these reasons The Coalition for Health AI (CHAI) was established. CHAI is a non-profit organization tasked with developing concrete guidelines and criteria for responsibly developing and deploying AI applications in healthcare. Working with the US government and healthcare community, CHAI creates a safe environment to deploy generative AI applications in healthcare, covering specific risks and best practices to consider when building products and systems that are fair, equitable, and unbiased. Groups like CHAI could be replicated in any industry to ensure the safe and effective use of AI.
Healthcare is on the bleeding edge of generative AI, defined by a new era of precision medicine, personalized treatments, and improvements that will lead to better outcomes and quality of life. But this didn’t happen overnight; the integration of generative AI in healthcare has been done thoughtfully, addressing technical challenges, ethical considerations, and regulatory frameworks along the way. Other industries can learn a great deal from healthcare’s commitment to AI-driven innovations that benefit patients and society as a whole.