4 Types of Data Analytics To Enhance Your Decisions
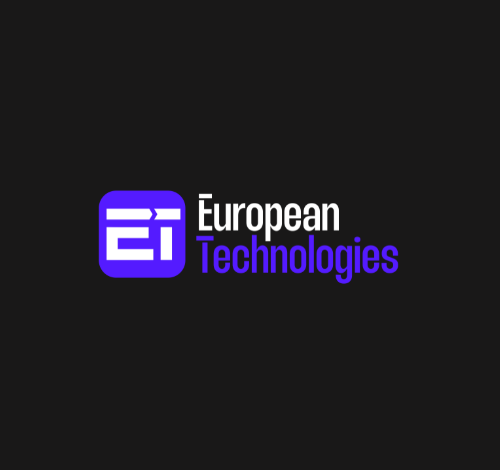
Many different types of data analytics can be employed to examine raw data, big data, or statistics to uncover valuable insights that businesses can use to inform their decision-making. Each type of data analytics offers a unique perspective on your data and provides distinct insights, patterns, correlations, and trends. The four main types of data analytics are descriptive, diagnostic, predictive, and prescriptive—understanding each can help you better analyze, interpret, and leverage your data to achieve your business goals.
Featured Partners: Data Analysis Software
Descriptive Analytics
Descriptive analytics involves summarizing historical data to understand changes that occurred. It provides an outline of observations in the past without delving deeper. This type of data analysis answers the question “What happened?” and lays the groundwork for further evaluation by establishing a baseline understanding of the data’s historical context.
Descriptive Analytics Use Cases
Descriptive analytics is used in numerous fields to summarize and understand data, including the following use cases.
Financial Reporting
Descriptive analytics in financial reporting entails organizing and summarizing historical financial data to provide a clear overview of a company’s performance. It covers key metrics like revenue, expenses, and profit margins, enabling stakeholders to understand past financial trends. Through visualizations and reports, descriptive analytics promotes informed decision-making by highlighting financial strengths, weaknesses, and areas for improvement.
Sales Reporting
Descriptive analytics can provide insights into patterns and trends from historical sales data. It lets you identify top-performing products, regions, or sales channels over time, gauge the effectiveness of promotions, and optimize inventory levels. This could lead to enhanced sales strategies and maximized revenue.
Website Traffic and Engagement Reports
Descriptive analytics can assess historical data on website visits, page views, and user engagement. It aids in determining popular content, peak traffic times, and user demographics. Using tools like Google Analytics, you can generate reports and visualizations to understand user behavior, optimize website content, and elevate overall user experience based on past performance.
Demand Trends
You can use descriptive analytics to understand and predict demand trends by analyzing customer behavior and preferences over time. This can improve business marketing strategies and product development.
Customer Segmentation
Descriptive analytics enables you to categorize customers based on shared characteristics, behaviors, or demographics for a more effective customer segmentation. By going over historical customer data, you can uncover segments with similar purchasing patterns. This information is valuable for tailoring marketing strategies, boosting customer engagement, and developing targeted products or services to meet the specific needs of different customer segments.
Descriptive Analytics Advantages
- Provides a clear picture of historical data in an easy-to-understand format
- Simplifies data interpretation, making it easier to communicate insights
- Helps identify outliers or anomalies in the data
- Enables quick comparisons between different groups or variables within a dataset
- Forms the foundation for more advanced analytics
Descriptive Analytics Disadvantages
- May oversimplify complex phenomena and has limited ability to explain causation
- Highly dependent on the quality of the sample; biased samples can lead to misleading conclusions
- Only offers insights based on currently available data, which might not give a full picture
- Cannot forecast future trends
- Does not provide actionable insights for the future
Descriptive Analytics Techniques
Common descriptive analytics techniques contribute to a comprehensive data analysis by simplifying its presentation, summarizing key aspects, and revealing patterns. Here are the most common:
- Data visualization—Presenting data using visual elements such as charts, graphs, and dashboards for easy understanding.
- Data aggregation—Combining data to present a high-level overview, often using methods like sum, average, count, or finding minimum/maximum values.
- Dispersion measures—Observing data variability using standard deviation, range, and interquartile range metrics.
- Summary statistics—Showing a concise overview of data through summary statistics like mean, median, mode, range, and standard deviation.
- Frequency distributions—Organizing data into histograms to track see distribution patterns.
Descriptive Analytics Tools
Like all types of data analytics, a wide range of tools is used in descriptive analytics. Some of the most common include spreadsheet software, data visualization tools, Business Intelligence (BI) tools, accounting software, and customer relationship management (CRM) systems.
How to Get Started with Descriptive Analytics
Getting started with descriptive analytics calls for collecting relevant data, data cleaning and preparation, selecting visualization tools, generating reports, seeking feedback, reviewing and refining analytics, and summarization.
Diagnostic Analytics
Diagnostic analytics delves deeper than descriptive analytics to reveal the underlying causes of observed phenomena. It aims to uncover relationships between variables and understand the factors contributing to specific outcomes by examining and scrutinizing historical data by answering the question, “Why did it happen?”
Diagnostic Analytics Use Cases
Here are some of the use cases where diagnostic analytics can be applied across different industries.
Employee Turnover Analysis
Diagnostic analytics can help Human resource (HR) departments to understand factors contributing to employee turnover. By taking a closer look at employee satisfaction surveys, performance data, and other HR metrics, your organization can identify areas for improvement.
Customer Churn Analysis
Analyzing customer churn using diagnostic analytics revolves around scrutinizing data to determine the factors contributing to customer attrition. This involves a thorough examination of customer behavior, feedback, and engagement metrics to unearth patterns and trends that may indicate reasons for customers discontinuing their engagement with a product or service.
Quality Control
You can apply diagnostic analytics to identify defects, irregularities, or patterns that may affect your product quality. This can include meticulously assessing sensor data, production metrics, inspection results, and customer complaints. Using diagnostic analytics for quality control helps you pinpoint the root causes of defects, allowing you to implement targeted corrective actions.
Identifying Outliers
Diagnostic analytics can help identify outliers in a dataset that may indicate errors in data collection, measurement, or exceptional cases that warrant further investigation.
Fraud Detection
You can use diagnostic analytics to detect unusual patterns that may indicate fraudulent activities in financial transactions. This could include scrutinizing financial data for irregularities or unexpected patterns in credit card usage or investment portfolios.
Diagnostic Analytics Advantages
- Gives insights into the factors impacting specific outcomes
- Supports informed decision-making based on a deeper understanding of events
- Facilitates the development of targeted solutions
- Reduces the likelihood of issues escalating into more significant challenges through early detection of anomalies or patterns that may lead to problems
- Improves strategic planning by promoting understanding the internal and external factors that influence business operations and goals
Diagnostic Analytics Disadvantages
- Requires in-depth analysis and expertise for accurate interpretation
- Can be resource-intensive, particularly when dealing with large datasets or complex algorithms
- Mainly focused on understanding past patterns, not predicting future ones
- Not practical for frequent use due to the depth of analysis and time required
Diagnostic Analytics Techniques
Diagnostic analytics techniques include root cause analysis (RCA), data profiling, regression analysis, comparative analysis, and error analysis. Each technique is designed for investigation and analysis, aiming to go beyond surface-level observations.
- Root cause analysis (RCA)—A systematic approach requiring a thorough investigation to discover the fundamental cause of issues.
- Data profiling—Examining data structure and content to identify anomalies or inconsistencies causing problems.
- Regression analysis—Assessing relationships between variables to detect patterns and dependencies, especially in understanding contributing factors.
- Comparative analysis—Contrasting different sets of data to pinpoint variations or discrepancies and potential issues.
- Error analysis—Investigating error logs and messages to understand the nature and frequency of errors, aiding in issue identification.
Diagnostic Analytics Tools
Tools used for diagnostic analytics include statistical analysis software, data drilling and data mining software, and software for anomaly detection.
How to Get Started with Diagnostic Analytics
The diagnostic analytics process entails defining the problem, gathering relevant data, formulating hypotheses, evaluating hypotheses, interpreting results, recommending solutions, implementing changes, and tracking outcomes.
Predictive Analytics
Predictive analytics involves forecasting future trends and outcomes using statistical algorithms, machine learning (ML), and data mining to analyze historical data. It goes beyond other types of big data analytics like descriptive and diagnostic analytics, focusing on anticipating what is likely to happen based on patterns identified in past data. Answering the question “What is likely to happen?”, this approach empowers organizations to find potential opportunities, assess risks, and make proactive decisions.
Predictive Analytics Use Cases
Predictive analytics gives organizations a competitive advantage and can be used in numerous scenarios, including the following examples.
Predicting Customer Churn
Businesses employ predictive analytics to identify customers who are likely to stop doing business with them. By predicting churn, your organization can proactively engage these customers with special offers or better service for retention.
Anticipating Consumer Trends
Predictive analytics helps brands anticipate consumer trends and product demands. This helps in optimizing inventory levels, managing supply chains, and increasing overall operational efficiency.
Sales and Demand Forecasting
Predictive analytics enables your business to forecast future sales and product demands by studying historical data, market trends, and various predictive modeling techniques. By leveraging ML algorithms and statistical models, businesses can anticipate fluctuations in sales and demand, identify peak seasons, optimize inventory management, and stay agile in response to changing market conditions.
Risk Assessment
Predictive analytics equips your organization to proactively address risks by anticipating market trends, recognizing operational vulnerabilities, and uncovering potential security threats. This necessitates examining past data to find patterns and indicators that may precede adverse events.
Predicting Employee Attrition
HR departments can employ predictive analytics to forecast employee attrition and potential employee turnover by reviewing historical workforce data and employee engagement metrics. This lets your organization take preventative action, such as targeted retention strategies and improved recruitment processes.
Healthcare Risk Classification
Healthcare organizations can use predictive analytics in singling out patients who are more likely to develop certain health conditions. This early identification allows healthcare professionals to act promptly and deliver preventive care that is specifically designed for those patients.
Predictive Analytics Advantages
- Enables informed decision-making based on data-driven forecasts and predictions
- Improves sales and marketing strategies by targeting specific demographics and personalizing campaigns for better customer engagement
- Streamlines planning and resource allocation through anticipating future demands and trends
- Mitigates risks by predicting potential challenges, allowing for proactive measures to minimize negative impacts
- Supports customer retention and satisfaction through predicting customer behavior and preferences
Predictive Analytics Disadvantages
- Heavily relies on the quality of input data; inaccurate, incomplete, or biased data can lead to unreliable predictions and skewed outcomes
- Integrating predictive models into existing systems and workflows can be complex, potentially leading to underutilized data
- Handling sensitive or personal data in predictive analytics raises privacy concerns and requires compliance with data protection regulations to avoid legal and ethical issues
- Predictive models might lose their precision over time due to shifts in market conditions and changes in consumer behaviors; regular updates are necessary to ensure accuracy
- Developing and maintaining predictive models can be resource-intensive, requiring skilled professionals, computational power, and ongoing updates to remain effective
Predictive Analytics Techniques
Some techniques typically employed in predictive analytics include time series analysis, machine learning, statistical modeling, regression analysis, decision trees, and neural networks.
- Time series analysis—Analyzing a sequence of data points collected over time to help understand and predict future points in the series.
- Machine learning (ML)—ML algorithms automatically learn patterns from data, improve over time, and predict outcomes.
- Statistical modeling—Creating mathematical models that represent relationships between variables in a dataset. These models use statistical techniques to estimate parameters and make predictions.
- Regression analysis—Models the relationship between a dependent variable and one or more independent variables to forecast numerical values.
- Decision trees—A visual representation of decision logic for predicting outcomes. These display decision-making processes in a tree-like structure for classification and regression tasks.
- Neural networks—A type of ML model structured like the human brain, with layers of interconnected nodes or “neurons.” They process data to recognize complex patterns and forecast results.
Predictive Analytics Tools
Tools used in predictive analytics can include ML libraries, data mining tools, data visualization tools, BI software, statistical modeling tools, and predictive analytics software.
How to Get Started with Predictive Analytics
Predictive analytics is an ongoing process that requires defining goals, data collection and cleaning, selecting prediction techniques, training and validating models, model deployment, making predictions, performance assessment, and continuous improvement.
Prescriptive Analytics
Prescriptive analytics is an advanced form of analytics that offers recommendations to achieve a specific result. It uses data, mathematical algorithms, and business rules to suggest the best course of action that your organization should take to refine decision-making processes.
This approach considers various scenarios, constraints, and potential outcomes, to guide decision-makers toward the most effective strategies. Essentially, prescriptive analytics answers the question “What should we do?” by giving actionable recommendations.
Prescriptive Analytics Use Cases
Prescriptive analytics can be used for a wide range of use cases across different sectors. Here are some of the most common.
Supply Chain Optimization
Prescriptive analytics enhances supply chain efficiency by using optimization algorithms and simulation tools. It scrutinizes extensive data on inventory levels, production capacities, transportation logistics, and market demand to formulate strategies for procurement, production, and distribution. This approach can lead to cost reductions, shorter lead times, and overall supply chain performance improvement.
Personalized Product Suggestions
In e-commerce, prescriptive analytics calls for analyzing customer behavior, purchase history, and preferences to come up with personalized product recommendations. By using ML algorithms, e-commerce platforms can suggest products tailored to individual users, increasing the likelihood of conversion and elevating customer satisfaction.
Proactive Fraud Prevention
Financial institutions use prescriptive analytics to spot and prevent fraudulent activities by evaluating transaction patterns, user behavior, and historical fraud data to develop models that detect anomalies and recommend immediate actions to mitigate potential fraud.
Dynamic Pricing Strategies Implementation
Prescriptive analytics can provide recommendations for dynamic pricing adjustments to support brands in making informed decisions to enhance competitiveness. By assessing factors such as demand, competitor pricing, and customer behavior, brands could potentially boost their revenue.
Data-Driven Treatment Plan Development
Prescriptive analytics allows healthcare institutions to offer personalized treatment recommendations by examining patient data, medical histories, and clinical research. This integration of optimization algorithms and decision support systems provides healthcare professionals with actionable insights for choosing treatments best suited to a patient’s unique conditions.
Marketing Campaign Enhancement
Prescriptive analytics generates recommendations for the most effective channels, timing, and content based on an examination of historical performance and customer segmentation to refine marketing campaign strategies.
Prescriptive Analytics Advantages
- Drives better decision-making with actionable recommendations
- Improves resource efficiency and reduces costs by recommending efficient resource allocation
- Enhances strategic planning
- Adapts strategies to changing conditions
- Increases revenue by suggesting best pricing strategies and targeted marketing approaches
Prescriptive Analytics Disadvantages
- Significantly dependent on accurate and comprehensive data; if the input data is incomplete, inaccurate, or outdated, it can lead to flawed recommendations
- Implementing recommended actions can be complex
- Raises ethical concerns related to privacy, particularly in areas like personalized marketing
- Deployment includes investment in technology, data infrastructure, and skilled personnel, which can have substantial costs
- Requires continuous monitoring and maintenance to guarantee relevance and accuracy
Prescriptive Analytics Techniques
Some of the techniques used in prescriptive analytics are optimization algorithms, simulation, Monte Carlo simulation, decision trees, and heuristic methods.
- Optimization algorithms—Helps find ideal solutions for complex problems with multiple variables and constraints.
- Simulation—Computer-based models of a situation and that simulate different actions to understand their potential impact.
- Monte Carlo simulation—A sub-type of simulation that involves generating repeated random samples to model the probability distribution of various outcomes and obtain numerical results.
- Decision trees—Graphical models that represent decisions and their possible consequences.
- Heuristic methods—The use of practical and intuitive approaches like educated guesses or rules of thumb to find good solutions in a reasonable amount of time.
Prescriptive Analytics Tools
Tools used for prescriptive analytics include optimization software, simulation tools, neural networks, prescriptive analytics platforms, supply chain optimization systems, advanced analytics platforms, BI tools, and AI/ML.
How to Get Started with Prescriptive Analytics
To perform effective prescriptive analytics, you must define objectives, collect and integrate relevant data, prepare data, select prescriptive analytics tools, build models, implement recommendations, monitor and assess performance, and constantly enhance predictive analytics models.
Bottom Line: Find the Right Type of Data Analytics to Transform Decision-Making
Data analytics can transform decision-making by delivering insights derived from analyzing large and complex datasets. Understanding the different types of big data analytics, what each is used for, and their strengths and weaknesses is critical in harnessing the full potential of data-driven decision-making.
Knowing the distinction between various types of data analytics allows you to fully leverage the array of data analytics tools and techniques. It can also assist you in strategically implementing the most appropriate approach for your specific business objectives, ensuring a sophisticated and informed application of data analytics resources
Keeping up with the latest in data analytics trends is essential in maintaining the accuracy of your data analysis. Read our article on data analytics trends and find out what’s new in data analytics.