How Generative AI Moonshots Can Reach Escape Velocity
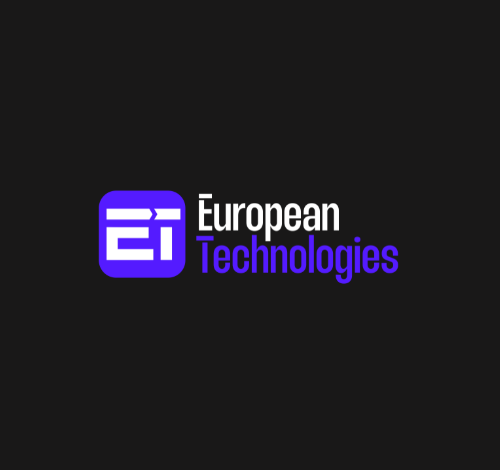
At a Glance
- Over 30% of Fortune Global 500 companies are investing in generative AI, but 85% of their announced initiatives are focused on incremental innovations, according to Bain analysis.
- That could soon shift; we see more companies ready to pursue AI moonshots.
- Leading companies are building confidence in their AI strategies through early wins and quickly identifying sustainable competitive advantages.
- They’re also balancing in-house development with partnerships and acquisitions, and they’re focusing early on getting the operating model right.
It’s not hyperbole to say that artificial intelligence’s current breakthrough moment is a once-in-a-generation opportunity for executives across industries to shift business boundaries and create enormous value. They recognize AI has the power to fundamentally change how consumers interact with the world and how work gets done. It’s early days, but things are moving fast. Now that the dust has settled from enterprises’ first wave of generative AI applications, many companies are ready to embark on bolder bets.
The question is, how can they give their AI moonshots the best chance to reach escape velocity?
Since large language models and generative AI broke through in late 2022, businesses across industries have invested enormous amounts of time and resources developing their initial use cases. Bain & Company analysis found that about 33% of Fortune Global 500 companies had publicly announced generative AI initiatives as of the end of February, so the actual number is certainly higher.
Meanwhile, in a down year for venture capital, global VC investments in generative AI start-ups surged from $5.1 billion in 2022 to $26.1 billion in 2023, according to Bain analysis of data from Crunchbase, PitchBook, and company websites. Although the vast majority of last year’s funding went toward companies building foundation models and developer tools, consumer and enterprise AI start-ups pulled in about $3 billion. They’re building applications spanning AI-powered drug discovery, language translation, content generation, and more. The upshot is that the landscape of generative AI application start-ups remains fragmented, with plenty of opportunity for disruption.
As with any new technology, most companies have invested first in use cases with a clearer, shorter path to investment returns. About 85% of the 235 generative AI initiatives announced by Fortune Global 500 companies through the end of February focused on incremental improvements to products, services, and efficiency, according to Bain’s analysis. Only 15% were pursuing more transformative changes such as expert task automation, AI agent-led customer interactions, and hyperpersonalization of offerings at scale (see Figure 1). None of the initiatives would be considered a moonshot or a fundamentally disruptive new business model.
So far, about 85% of large enterprises’ generative AI applications target more incremental improvements in products, services, and efficiency
It’s important to note that this analysis doesn’t include all the ways AI is being infused into everyday office software tools, and there’s some variation in the value of productivity use cases based on industry (what the cost structure looks like) and geography (relative cost of labor). Nevertheless, the initial bias toward incremental innovation is unmistakable.
But that could start to change in 2024. From the market signals we see, more companies are ready—whether operationally, financially, culturally, or all three—to go after more disruptive AI opportunities.
Balancing speed vs. risk
Speed will be even more critical to capturing a competitive advantage than with past technology advances. The pace of generative AI progress and the ability to rapidly develop a working proof-of-concept application are radically different from anything executives have experienced. But the speed of change also increases risk for new AI ventures: Customer expectations and regulations are evolving as quickly as the underlying technology, making it even more difficult to project how the market will play out. Finding the appropriate balance between speed and risk management has already begun to separate leaders from laggards.
Leading companies are setting a bold AI ambition that syncs with their firm’s overall business strategy and is guided by a deep understanding of what users and customers need. To hedge against risk, companies are positioning themselves to navigate through “two-way doors” that they can more readily back out of or pivot away from if results don’t materialize as anticipated.
What does that look like in practice? Based on our experience helping clients worldwide build businesses and develop generative AI products and services, four key actions can improve the odds of success for a company’s bold AI bets.
Four keys to bold AI bets
1. Use early wins to gain conviction. While many executives recognize that the opportunity is real, some might be hesitant to push their chips in with generative AI moonshots after feeling burned by other hyped technologies in recent years.
Emerging leaders start by setting a threshold of tolerable risk that protects the company without constraining its ability to experiment. To some extent, speed matters more than which use cases get pursued first. Starting with lower-risk opportunities (such as internal productivity use cases that require less-sensitive data) can generate near-term successes and incremental value, helping build internal confidence that the company can lean into AI for serious growth.
Getting a working version of the application into the hands of customers or end users as quickly as possible has helped accelerate progress for many of our clients in generative AI. As with other technology development, real user feedback enables companies to pinpoint what works and improve the product—or scrap it if it becomes clear that it won’t generate return on investment.
Early wins can help organizations strengthen their capabilities in AI, better understand how it could shape customer needs, create new ways to reach customers, and support organizational change management and employee buy-in. These stepping-stone use cases can also help fund bigger AI bets.
2. Quickly determine which proprietary assets will deliver sustained competitive advantage. Emerging leaders are identifying their key assets quickly, recognizing they need to capitalize before competitors develop a lead that’s hard to overcome.
It’s no secret that access to troves of high-quality data provides the best fuel for generative AI applications, which means large incumbents often have an edge. But it’s becoming increasingly clear that unlocking the most value from generative AI requires deeply understanding users and tuning the applications to truly meet their needs. Better insights into end users often result in more refined interactions with the AI, which raises the odds of customer adoption.
A frequent challenge for companies is that their proprietary data isn’t always easily accessible, or it’s not even clear where it resides. For example, sometimes companies have valuable knowledge and expertise that lives in staff members’ heads but hasn’t been systematically recorded or codified (e.g., sales tactics or fixes for common product maintenance issues). It may require a heavy upfront investment of time and resources to capture that knowledge in a database that AI can analyze, but it’s worthwhile if the end result is a distinctive data set that helps build a sustainable competitive edge.
3. Find the right balance between buying, building, and partnering. One of the most heated debates in every boardroom right now is when it makes sense to build a custom AI solution in-house and when it’s better to acquire or partner with someone else. No company has fully cracked the code, but one key consideration is whether the solution is built upon a proprietary asset that will provide true competitive advantage over the long term vs. capabilities that will become ubiquitous over time (e.g., data science).
It’s hard to imagine new AI growth businesses that aren’t supported at least in part by components developed in-house. But that doesn’t mean companies have to build everything from scratch either. For example, can they build solutions in ways that allow for relatively painless swapping of large language models, so companies can use the best available?
Emerging leaders are also laying the groundwork for acquisitions to add AI capabilities or expand their proprietary data sets.
4. Establish a winning operating model to incubate new ventures. One thing the emerging generative AI leaders have in common: sponsorship and sustained engagement from top executives. When executives are invested in the AI work and tracking outcomes—at some companies, the CEO and board chair join weekly steering committee meetings on AI for months at a time—it creates a sense of urgency and shared commitment across the company. It also helps remove internal hurdles to getting things done.
The specific makeup of the AI operating model will vary by company. But, in general, creating some degree of centralized resources and fast-paced decision-making processes can make a difference, especially for larger companies with multiple business units. It often helps keep different internal teams on the same page, fosters knowledge and experience sharing, ensures prioritization and optimal use of scarce talent and resources, and avoids inefficiencies and rework.
Establishing nimble governance early on allows companies to burst out of the gate; having these processes in place will continue to pay dividends over time. Leaders have adopted a stage-gate mindset and governance processes that focus on measurement of product adoption, customer sentiment, and business outcomes, with the expectation to pause, pivot, or accelerate work quickly based on learnings. Setting the right tempo for risk management and instituting lean approval processes are also critical elements of governance to get right. In the early days of generative AI experimentation, for expediency many companies rely on case-by-case approvals from risk and compliance departments. But that won’t be tenable over the long term as the company pursues a larger pipeline of AI products. The right operating model will allow the organization to both measure and balance risks and opportunities appropriately, and still move at pace.
The future is wide open
Generative AI has made enormous progress in a short time. But it’s still too early in the technology’s journey to fathom all the ways AI could transform industries and what its full potential will be. With the right strategy and operating model in place, executives who boldly invest in potential AI moonshots can position their companies for growth and leadership for years to come.