Next-Gen AI: OpenAI and Meta’s Leap Towards Reasoning Machines
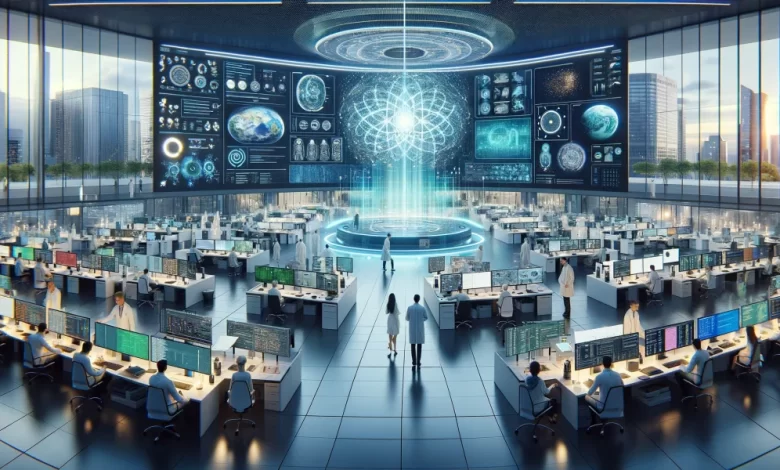
OpenAI and Meta, pioneers in the field of generative AI, are nearing the launch of their next generation of artificial intelligence (AI). This new wave of AI is set to enhance capabilities in reasoning and planning, marking significant advances towards the development of artificial general intelligence. This article explores these forthcoming innovations and the potential future they herald.
Paving the Way for Artificial General Intelligence
Over the past few years, OpenAI and Meta have made significant strides in advancing foundation AI models, essential building blocks for AI applications. This progress stems from a generative AI training strategy where models learn to predict missing words and pixels. While this method has enabled generative AI to deliver impressively fluent outputs, it falls short in providing deep contextual understanding or robust problem-solving skills that require common sense and strategic planning. Consequently, when tackling complex tasks or requiring nuanced understanding, these foundation AI models often fail to produce accurate responses. This limitation highlights the need for further advancements towards developing artificial general intelligence (AGI).
Furthermore, the quest for AGI seeks to develop AI systems that match the learning efficiency, adaptability, and application capabilities observed in humans and animals. True AGI would involve systems that can intuitively process minimal data, quickly adapt to new scenarios, and transfer knowledge across diverse situations— skills that stem from an innate understanding of the world’s complexities. For AGI to be effective, advanced reasoning and planning capabilities are essential, enabling it to execute interconnected tasks and foresee the outcomes of its actions. This progression in AI aims to address current shortcomings by cultivating a deeper, more contextual form of intelligence capable of managing the complexities of real-world challenges.
Toward a Robust Reasoning and Planning Model for AGI
Traditional methodologies for instilling reasoning and planning capabilities in AI, such as symbolic methods and reinforcement learning, encounter substantial difficulties. Symbolic methods necessitate the conversion of naturally expressed problems into structured, symbolic representations—a process that requires significant human expertise and is highly error-sensitive, where even slight inaccuracies can lead to major malfunctions. Reinforcement learning (RL), meanwhile, often requires extensive interactions with the environment to develop effective strategies, an approach that can be impractical or prohibitively costly when data acquisition is slow or expensive.
To overcome these obstacles, recent advancements have concentrated on enhancing foundational AI models with advanced reasoning and planning capabilities. This is typically achieved by incorporating examples of reasoning and planning tasks directly into the models’ input context during inference, utilizing a method known as in-context learning. Although this approach has shown potential, it generally performs well only in simple, straightforward scenarios and faces difficulties in transferring these capabilities across various domains—a fundamental requirement for achieving artificial general intelligence (AGI). These limitations underscore the need to develop foundational AI models that can address a wider array of complex and diverse real-world challenges, thereby advancing the pursuit of AGI.
Meta and OpenAI’s New Frontiers in Reasoning and Planning
Yann LeCun, Chief AI Scientist at Meta, has consistently emphasized that the limitations in generative AI’s capabilities for reasoning and planning are largely due to the simplistic nature of current training methodologies. He argues that these traditional methods primarily concentrate on predicting the next word or pixel, rather than developing strategic thinking and planning skills. LeCun underscores the necessity for more advanced training techniques that encourage AI to evaluate possible solutions, formulate action plans, and understand the implications of its choices. He has disclosed that Meta is actively working on these sophisticated strategies to enable AI systems to independently manage complex tasks, such as orchestrating every element of a journey from an office in Paris to another in New York, including the commute to the airport.
Meanwhile, OpenAI, renowned for its GPT series and ChatGPT, has been in the spotlight for its secretive project known as Q-star. While specifics are scarce, the project’s name hints at a possible combination of Q-learning and A-star algorithms, important tools in reinforcement learning and planning. This initiative aligns with OpenAI’s ongoing efforts to enhance the reasoning and planning capabilities of its GPT models. Recent reports from the Financial Times, based on discussions with executives from both Meta and OpenAI, highlight the joint commitment of these organizations to further develop AI models that perform well in these crucial cognitive domains.
Transformative Effects of Enhanced Reasoning in AI Systems
As OpenAI and Meta continue to enhance their foundational AI models with reasoning and planning capabilities, these developments are poised to greatly expand the potential of AI systems. Such advancements could lead to major breakthroughs in artificial intelligence, with the following potential improvements:
- Improved Problem Solving and Decision Making: AI systems enhanced with reasoning and planning capabilities are better equipped to handle complex tasks that necessitate an understanding of actions and their consequences over time. This could lead to progress in strategic gameplay, logistics planning, and autonomous decision-making systems that require a nuanced grasp of cause and effect.
- Increased Applicability Across Domains: By overcoming the constraints of domain-specific learning, these AI models could apply their reasoning and planning skills across various fields such as healthcare, finance, and urban planning. This versatility would allow AI to effectively address challenges in environments markedly different from the ones they were initially trained in.
- Reduced Dependence on Large Data Sets: Moving towards models that can reason and plan with minimal data reflects the human ability to quickly learn from few examples. This reduction in data needs lowers both the computational burden and the resource demands of training AI systems, while also boosting their speed in adapting to new tasks.
- Steps Toward Artificial General Intelligence (AGI): These foundational models for reasoning and planning bring us closer to achieving AGI, where machines might someday perform any intellectual task that a human can. This evolution in AI’s capabilities could lead to significant societal impacts, sparking new discussions on the ethical and practical considerations of intelligent machines in our lives.
The Bottom Line
OpenAI and Meta are at the forefront of developing the next generation of AI, focused on enhancing reasoning and planning capabilities. These improvements are key to moving closer to Artificial General Intelligence (AGI), aiming to equip AI systems to handle complex tasks that require an intricate understanding of the broader context and long-term consequences.
By refining these capabilities, AI can be applied more broadly across diverse fields such as healthcare, finance, and urban planning, reducing the dependency on large datasets and improving adaptability. This progress not only promises to expand the practical applications of AI but also brings us nearer to a future where AI might perform as capably as humans across all intellectual tasks, sparking important conversations about the integration of AI into everyday life.