Google builds an AI model that can predict future weather catastrophes
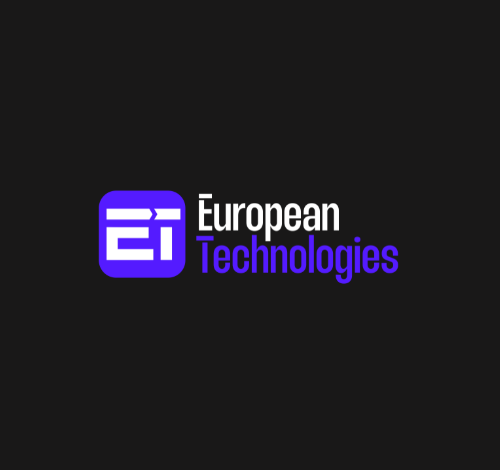
Google has released an artificial intelligence (AI) model that it claims can generate accurate weather forecasts at scale — while being cheaper than conventional physics-based forecasting.
The “Scalable Ensemble Envelope Diffusion Sampler” (SEEDS) model is designed similarly to popular large language models (LLMs) like ChatGPT and generative AI tools like Sora — which generates videos from text prompts.
SEEDS generates many ensembles — or multiple weather scenarios — much quicker and cheaper than traditional predicting models can. The team described its findings in a paper published March 29 in the journal Science Advances.
Weather is difficult to predict, with many variables that can lead to potentially devastating weather events — from hurricanes to heat waves. As climate change worsens and extreme weather events become more common, accurately predicting the weather can save lives by giving people time to prepare for the worst effects of natural disasters.
Physics-based predictions currently used by weather services collect various measurements and give a final prediction that averages many different modeled predictions — or an ensemble — based on all the variables. Instead of a single forecast, weather forecasting is based on a set of predictions per forecast cycle that provides a range of possible future states.
Related: ‘Jailbreaking’ AI services like ChatGPT and Claude 3 Opus is much easier than you think
This means most weather predictions are accurate enough for more common conditions like mild weather or warm summer days, but generating enough forecast models to find the likely outcome of an extreme weather event is out of the reach of most services.
Current predictions also use deterministic or probabilistic forecast models, in which random variables are introduced to the initial conditions. But this leads to a rapidly higher error rate — meaning that accurately predicting extreme weather and weather further in the future is hard to get right.
Unforeseen errors in the initial conditions can also vastly affect the prediction outcome as the variables grow exponentially over time and modeling enough forecasts to account for variables down to such minute detail is expensive. The Google scientists estimated that 10,000 predictions in a model are needed to forecast events that are only 1% likely to happen.
SEEDS produces prediction models from physical measurements collected by weather agencies. In particular, it looks at the relationships between the potential energy unit per mass of Earth’s gravity field in the mid-troposphere and sea level pressure — two common measures used in forecasting.
Traditional methods only feasibly produce ensembles of around 10 to 50 predictions. But by using AI, the current version of SEEDS can extrapolate up to 31 prediction ensembles based on just one or two “seeding forecasts” used as the input data.
The researchers tested the system by modeling the 2022 European heatwave using historical weather data recorded at the time. Just seven days before the heatwave, the U.S. operational ensemble prediction data gave no indication such an event was on the horizon, Google representatives said in the blog post of its research portal. They added that ensembles with less than 100 predictions — which is more than conventional would also have missed it.
The scientists described the computing costs associated with performing calculations with SEEDS as “negligible” compared with today’s methods. Google says the AI system also had a throughput of 256 ensembles for every three minutes of processing time in a sample Google Cloud architecture — which can be scaled easily by recruiting more accelerators.