Early automated detection system for skin cancer diagnosis using artificial intelligent techniques
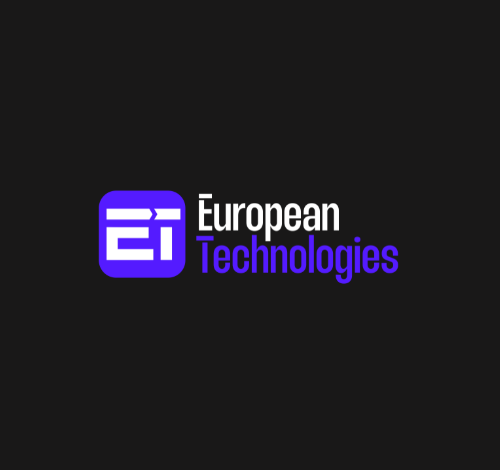
This paper proposed an intelligent system for skin cancer detection. The implemented system is developed to detect benign and malignant skin lesions. Multiple steps, including pre-processing, different methods for segmentation, features extraction/features selection, and different methods of classification are used for analyzing the automated dermoscopic images.
The dermoscopic images dataset used in this paper is from PH2 Dataset47. This dataset is publicly available47. PH2 Dataset is a widely used dataset in the field of dermatology and skin cancer detection. PH2 dataset contains dermoscopic images that were obtained at the Dermatology Service of Hospital Pedro Hispano (Matosinhos, Portugal) under the same conditions through Tuebinger Mole Analyzer system using 20× magnification. The dermoscopic images are 8-bit RGB color images with a resolution of 768 × 560 pixels47. PH2 Dataset is a widely used dataset in the field of dermatology and skin cancer detection. PH2 dataset contains three types of skin diseases, they are Atypical Nevi, Melanoma and Common Nevus. This dataset includes 200 dermoscopic images (80 common nevus, 80 atypical nevi, and 40 melanomas). The dermoscopic images were selected randomly for training and test, as 80% for training and 20% for test. GUI for this study was implemented. The system configuration used for the proposed models was Intel Core i5 with processor 1.80 GHz and 8 GB of RAM. The system was implemented using MATLAB program. Figure 1 shows the flowchart of the implemented proposed system.
-
1.
Pre-processing
Each image has several noises, therefore, the noise that appeared in the images should be eradicated for improving identification process. Pre-processing includes reading the image and applying sequence of hair removal filters using DullRazor algorithm48; negative the image; enhancing the contrast of image; gray scale transformation; converting it to grayscale; noise filtering by median filter; and finding the negative of image by finding maximum of this image. The proposed system is characterized by using series of filters to enhance the contrast of the image. These filters are median, Gaussian and lee filters. Negative of enhanced image is applied. Smooth images are obtained after pre-processing step.
-
2.
Segmentation
Segmentation is a technique to separate the objects from their background. It can adapt to the complex morphology of biological structures. In the step of segmentation, Adaptive snake and Region growing algorithms are used and modified.
In the segmentation process for skin cancer detection, AS and RG algorithms offer valuable advantages including efficiency, flexibility, incorporation of prior knowledge, adaptation to image variability, and exploitation of local homogeneity. The choice between these two algorithms is dependent on factors such as the complexity of the lesion, the quality of the input images, and computational resources available.
Segmentation using adaptive snake49 includes finding the size of interested image; creating a mask of zeros by the same size of the interested image; assigning one value for a specific region in the created mask to snake around lesion; resizing all images to be the same; and applying the mask on the interested image. The result will be the interesting lesion.
AS algorithm is deformable model that can adapt to the contours of skin lesions. This flexibility enables AS to accurately identify irregularly shaped lesions, which is common in skin cancer cases. In addition, AS often incorporate prior knowledge about the shape and appearance of skin lesions, including color, texture, and gradient information. It can help improve the accuracy of segmentation by guiding the snake towards relevant features. Their shape and position based on the characteristics of the image can be adjusted, such as intensity gradients and edges. This flexibility makes AS robust to variations in image quality, lighting conditions, and skin types.
Segmentation using region growing50 includes finding the size of interested image; specifying the lesion coordinates; determining the point form where the machine begin to grow by knowing X & Y Coordinates; applying opening and closing morphological methods.
RG starts from one or more seed points within the lesion and iteratively grows the region by adding adjacent pixels that satisfy certain criteria, such as similarity in intensity or color. This seed-based approach allows for the segmentation of lesions without requiring explicit boundary information. RG exploits the concept of local homogeneity within skin lesions, meaning that pixels within the lesion exhibit similar characteristics in terms of color, texture, or intensity. Therefore, even in the presence of noise or variations in illumination, RG algorithms can accurately segment lesions. In addition, it is computationally efficient and can produce segmentation results quickly, making them suitable for real-time or large-scale applications. Compared to other traditional image segmentation techniques, such as thresholding or clustering, RG offers unique characteristics and advantages including boundary sensitivity and it considers local pixel relationships and can capture fine-grained details and complex boundaries.
The evaluation of AS and RG algorithms is achieved using accuracy as evaluation metric. Accuracy measures the overall correctness of the segmentation results, considering both true positive and true negative pixels.
-
3.
Features extraction
The next important step is deriving the features from the affected region. The extracted feature is reflection of the affected area information51.
Gray Level Co-occurrence matrix (GLCM) is used for features extraction. GLCM is a statistical method to examine and characterize the texture of the image. It is a second-order statistical texture analysis method. It is also known as the gray-level spatial dependence matrix52,53,54,55.
GLCM Parameters include Autocorrelation, Contrast, Correlation, Cluster-Prominence, Cluster Shade, Dissimilarity, Energy, Entropy, Homogeneity, Maximum probability, Sum of squares, Sum average, Sum variance, Sum entropy, Difference variance, Difference entropy, Information measure of correlation1, Information measure of correlation, Inverse difference normalized (INN), Inverse difference moment normalized52,53,54,55.
ABCDE rules of dermatology are used for features extraction. ABCDE rules are the commonly approved approaches for skin lesions detection. The selected features are shape including asymmetry and border irregularity, color variance, diameter, area of object, and roundness39,56,57.
ABCDE rules for features extraction are as the following:
-
(1)
Shape
-
(a)
Asymmetry: The lesion asymmetry was evaluated by calculating the area with inner and outer of the lesion, as follows58,59:
$$ASI= \frac{\Delta AK}{AL}\times 100$$
(1)
where, ASI is asymmetry index. ΔAK is the area between the two halves of the lesion and AL is lesion area.
-
(b)
Border irregularity: The edge of a malignant lesion usually exhibits four factors of interest, density, fractal dimension, radial variability and the extent to which its contour exhibits small irregularities. Border Irregularity is evaluated as follows60:
$$I= \frac{ab}{2\pi (a2+b2)}\frac{p2}{\Delta A}$$
(2)
where, I denotes irregularity with a and b representing the lengths of major and minor axes of the lesions. P is the perimeter of the lesion and ΔA is the area of corresponding.
-
(a)
-
(2)
Color Variance
This feature helps in finding the variations in colors among several types of images by converting images from RGB to HSV56.
-
(3)
Area of Object
Lesion images can be classified by finding area of segmented lesion or area of interest. This can be done by converting image to black and white and segment lesion and find diameter from ‘regionprops’60.
-
(4)
Diameter
Lesion images can be classified by finding the diameter of segmented lesion or area of interest. This can be done by converting image to Black & White and segment lesion and find diameter from ‘regionprops’. Diameter is evaluated as follows46,51:
$$Diameter=\sqrt{4*Area \,\, of \,\,object/\pi }$$
(3)
-
(5)
Roundness
Roundness of lesion can be calculated after finding area of object to distinguish common lesions from diseased one.
Roundness is evaluated as follows60:
$$Roundness=\sqrt{4*\pi *Area \,\,of \,\,object/Perimeter^{2}}$$
(4)
-
4.
Classification
ANN and SVM algorithms are used for classification of skin cancer. Classification includes specifying all image’s features; entering input data and target data; applying validation and performance on it; and using train data and test data.
ANN is also known as Neural Networks. ANN are trained using a supervised learning approach. There are three types of computational nodes. There is input layer, nodes in it have a connection with the hidden layer. A typical ANN consists of multiple hidden layers. The number of input units connected to the hidden layer depends on the dataset. Output nodes produce the final output of the neural network after receiving the processed data from the hidden layers. The number of output nodes depends on the nature of the task that the network is designed for. ANN is particularly useful for skin cancer detection because ANN is excellent in learning complex representations from raw data. It can learn complex patterns and relationships from the input data, which is crucial for accurately classifying skin lesions.
SVM is used for classification, it is supervised learning model with associated learning algorithms that analyze data and recognize patterns, used for classification and regression analysis. SVM takes a set of input data and predicts, for each given input, which of two possible classes forms the output. SVM aims to find the hyperplane that best separates different classes in the input space. It works by mapping input data into a higher-dimensional feature space and finding the optimal separating hyperplane with the maximum margin between classes. SVM is effective in skin cancer detection because it can handle high-dimensional data and is robust to overfitting. SVM can also efficiently handle nonlinear relationships in the data by using kernel functions, allowing it to classify skin lesions accurately. SVM is widely used to classify digital dermoscope images61.
Both ANN and SVM offer advantages for skin cancer detection. By learning from a variety of training data, ANN can adjust to variations in imaging conditions, lesion characteristics, and patient demographics. In real clinical settings, this adaptability can enhance generalization performance. Compared to other ML models, SVM is less prone to overfitting, especially when using appropriate regularization techniques and kernel functions. This robustness is helpful in the detection of skin cancer, where the ability to generalize to new data is essential. SVM can perform well even with relatively small training datasets such as PH2 dataset, making it suitable for skin cancer detection where collecting large amounts of labeled data may be challenging.
In the classification process of skin cancer detection, both ANN and SVM algorithms are typically trained using labeled datasets containing various features extracted from images of skin lesions, such as color, texture, and shape information. Once trained, these algorithms can classify new skin lesion images into different categories, such as benign or malignant, based on the learned patterns and relationships in the data. The choice between ANN and SVM depends on factors such as the size and complexity of the dataset, computational resources available, and the desired performance metrics. Both algorithms have demonstrated promising results in skin cancer detection and are actively used in research and clinical applications.
The accuracy of skin cancer detection depends on the efficiency of classification.
-
5.
Evaluation
Evaluation metrics are very important in the development, validation, and deployment of skin cancer detection algorithms. They provide critical insights into algorithm performance, guiding improvements, supporting clinical decision-making, and ultimately contributing to better patient outcomes.
The efficiency of the proposed system is evaluated using the following metrics:
Accuracy: This measure records the correct and incorrect recognized samples of each class according to confusion matrix to evaluate the classification quality. A confusion matrix is a binary classification which is determined as TP: true positive, FP: false positive, FN: false negative, TN: the true negative amount(s). Accuracy is evaluated as follows62:
$$Accuracy= \frac{TP+TN}{TP+TN+FP+FN}$$
(5)
Sensitivity or Recall is called true positive rate and it measures the proportion of actual positives that are correctly identified as such (e.g., the percentage of sick people who are correctly identified as having the condition). Sensitivity is evaluated as follows32:
$$sensetivity= \frac{TP}{TP+FN}$$
(6)
Specificity is called true negative rate and it measures the proportion of actual negatives that are correctly identified as such (e.g., the percentage of healthy people who are correctly identified as not having the condition). Specificity is evaluated as follows63:
$$Specificity= \frac{TN}{FP+TN}$$
(7)
Precision is called positive predictive value. It is the fraction of relevant instances among the retrieved instances. Precision is evaluated as follows32,63:
$$Precision=\frac{ TP}{TP+FP}$$
(8)
Dice or F1 Score is a measure of a test’s accuracy. It considers both the precision and the recall of the test to compute the score. F1 score will be best value at 1 when the system achieves perfect precision and recall; and F1 will be worst at 0. F1 score is evaluated as follows23:
$$F1 score=Dice=2.\frac{precision.recall}{precision+recall}$$
(9)
The Jaccard index is known as Intersection over Union (IoU) and the Jaccard similarity coefficient. It is a statistic used for comparing the similarity and diversity of sample sets. The Jaccard coefficient measures similarity between finite sample sets. It is defined as the size of the intersection divided by the size of the union of the sample sets. Jaccard is evaluated as follows64:
$$Jaccard=\frac{F1}{2-F1}$$
(10)
Matthews correlation coefficient (MCC) is calculated as follows65:
$$MCC= \frac{\left(TP.TN\right)-(FP.FN)}{\sqrt{\left(TP+FP\right).\left(TP+FN\right).\left(TN+FP\right).(TN+FN)}}$$
(11)