Generative AI tools can enhance climate literacy but must be checked for biases and inaccuracies
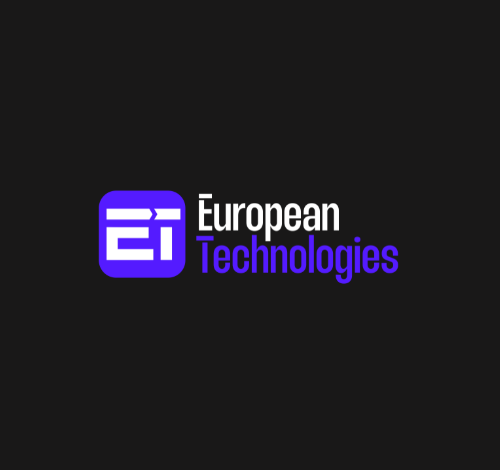
By focusing on ChatGPT as a case study, our exploratory study achieves one of the first steps toward informing users of generative AI tools’ potential strengths and weaknesses relevant to climate change literacy. By comparing three major hazards (floods, droughts, and cyclones) reported for each country by ChatGPT and comparing each to the validation data, we identified more accuracies than inaccuracies in ChatGPT’s responses—but not enough to conclude that the tool, when used in this way, is truly reliable. For example, ChatGPT tends to underestimate vulnerability to droughts, as ChatGPT reports droughts as a primary risk for considerably fewer countries than the trusted validation data do. This presents a false negative type error, which may potentially mislead ChatGPT’s users, who are currently formulating a sense of security and severity. For floods and cyclones, however, the opposite is true: most inaccuracies stem from false positives. Depending on the hazard, these trends in false positives/negatives present important biases and limitations that users should be aware of.
Despite the inaccuracies both types (false positive and false negative) clearly present, a considerable level of agreement is found between the ChatGPT responses and validation data for cyclones and floods. This is confirmed by the high accuracy scores across the 10 iterations of the GPT-4 model. However, the results also report a relatively lower level of agreement for droughts, as evidenced by lower accuracy scores than the other two hazard cases. Overall, our results suggest that, although the false positive bias should be kept in mind, ChatGPT may be used—with caution—as a starting point for users looking to gain climate literacy regarding some hazards, like floods and cyclones. However, considering droughts, more caution should be employed, as false negatives are arguably more dangerous in this context and overall accuracy is lower.
One should naturally ask what the origins of these inaccuracies might be. While identifying true causes is beyond the scope of this exploratory study, we suggest a few possible factors that may influence the performance of ChatGPT in this context. First, we must consider that this study was conducted entirely in English. As OpenAI has acknowledged, a bias toward English and perspectives aligning with Western cultures exists in the AI33. This bias may be relevant both to the responses generated by ChatGPT, which cater to Western, English-speaking users, as well as the AI’s processing of prompts—i.e., it may comprehend prompts from native English-speakers best. This situation is especially important to consider for regions within the Global South, where climate literacy is an important, yet poorly understood issue34. Perceptions of climate change risk vary widely across different cultures35, making even small semantic changes in ChatGPT responses potentially impactful. This language-related bias—in both ChatGPT functioning and user experience—introduces an additional variable to consider, the effects of which are not yet fully understood and may account for general variation in results, if this study were repeated in a non-English language. Additionally, regarding the lower accuracy for droughts (as compared to floods and cyclones), we must consider how such hazards are defined. The IPCC itself has acknowledged that drought is a relative term36, depending on many factors and contexts. Definitions in various sources other than the IPCC and related data sources may, therefore, vary more than other hazards like cyclones, which are more prominent and transparent in definitions (i.e., there is no debate over ongoing cyclones). This could partly explain the decreased accuracy in our validation of droughts, as opposed to floods and cyclones. Overall, this issue related to the definitions of hazards might contribute to the uncertainties of our analytical results, which future studies can examine through sensitivity analyses.
While not completely accurate compared to the validation data, GPT-4 offers a suggested pattern of consistency and reliability in its output regarding topic counts across 10 iterations. However, GPT-3.5 demonstrates unreliability as it produces errors when creating its responses, which we never encountered with GPT-4. Therefore, if possible, our results recommend that users employ GPT-4 rather than GPT-3.5. While it is unsurprising that GPT-4—the more advanced and costly version—performs better than the default version (GPT-3.5), this suggests potential ethical issues regarding tools available to users of different socioeconomic positions37,38,39. These potential ethical concerns can be especially relevant considering that those in developing economies have been some of the fastest populations to adopt applications of ChatGPT31.
We believe that a comprehensive examination of the capabilities of generative AI tools, such as ChatGPT and Bard, will likely grow in value, considering their quickly increasing role in climate literacy25,26 and their potential—yet debated—beneficial applications in the general education sector22,27,40 within countries where it is available. While providing insight into generative AI’s ability to summarize climate change-related hazards on a global, country-level scale, our study contains limitations that should not be overlooked. By utilizing the default parameters and API service that was initiated at each iteration, we provide data that, to the best of our knowledge, is minimally influenced by the user’s prompt history16,41. However, because of the black-box nature of AI models42, it must be noted that individual users may experience different outputs. Further, we recommend that future studies consult OpenAI’s documentation for relevant updates to either GPT version (since December 2023), as OpenAI regularly updates each model. Another variable to consider is that of user demand—might the performance of either version, particularly GPT-3.5, degrade with increased user demand at a given time? Next, we must also consider the limitations of the BERT NLP processing model with which we consolidated the ChatGPT responses into 50 themes. While the NLP model allows us to automate the consolidation process and reduce human error, and we employed the Davies-Bouldin Index, Silhouette, and Within-Cluster Sum of Squares scores (Supplementary Fig. 1), BERT is not perfect, and minor errors in clustering are possible, such as a group of temperature change topics including the more general topic of ‘arctic change.’ However, because BERT takes context into account, such an example may have related to temperature change in the original text. Regardless, this reminds us that BERT functions as a ‘black box’ model, which leaves us with unknowns that, for the time being, we simply accept. Keeping this in mind, we state that, within reasonable feasibility, the BERT model still offers improvements to this study’s approach in accuracy (eliminating human error) and efficiency (completing the same job manually would be nearly impossible, requiring contextual analysis of thousands of topics). Therefore, considering the high sample rate, we conclude that sparse random errors are acceptable for the scope of our study, especially in comparison to a manual approach. Thus, future studies are recommended to mitigate these uncertainties and limitations of the NLP model to provide a more robust theme consolidation result. Finally, the likely bias relating to the English language should be considered in additional cases43.
Further work should continue to comprehensively investigate the performance of the many additional emerging generative AI tools, such as Google’s Bard and ChatClimate (www.chatclimate.ai/)—a customized large language model developed by researchers26 for climate literacy-related use. Future studies are also recommended to quantify the limitations of these tools as precisely and comprehensively as possible. Potential geographic biases resulting from training datasets should also be examined more quantitatively16,44,45. One potential means to conduct further investigation into this issue would be to conduct a Delphi study46, which could offer insights before a wealth of established literature is available. Finally, developing educational recommendations for potential users of these AI tools is essential. More studies are being published, which indicate that prompt engineering and parameter-setting for GPT-4 are key for utilizing the tool effectively16. In light of this, we recommend further studies to examine the factors discussed here and develop best-practice guidelines. While most studies now focus on GPT-4 and its many additional capabilities, it is important to inform users of biases present in GPT-3.5, as many users, especially non-academic, will still use only the default version. This study puts forth an overview of country-level vulnerabilities to climate change-related hazards as told by both versions of ChatGPT as of December 2023.
In conclusion, climate change adaptation strategies will be dependent on the upcoming generations and their climate literacy—people’s understanding of climate change and willingness to be involved in mitigation and adaptation. This is a crucial point in the future of our planet, as projections show that waiting any longer to reduce climate emissions may result in a point of irreversible consequences47. Moreover, considering the growing importance of generative AI tools and their uptake by individuals worldwide, future studies on the combined topic of generative AI tools and climate literacy should commence with the ultimate goal of disseminating findings to enable informed, discerning use of ChatGPT and other increasingly popular generative AI platforms toward the pressing issue of climate change.