Can Generative AI Explain or Innovate?
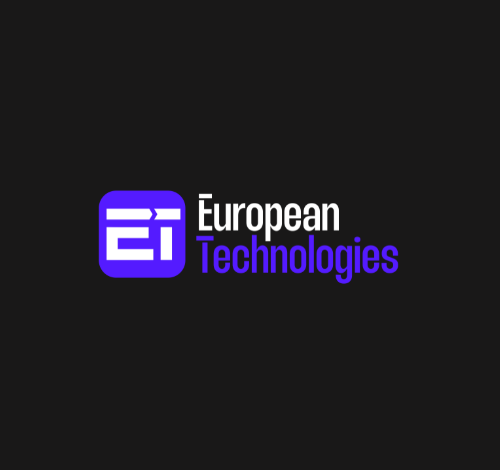
Generative AI burst onto the scene in November 2022 with the advent of ChatGPT, and many people, including me, have been amazed at its intelligence. For example, it appears to have human-level capability to generate and evaluate explanatory hypotheses. But these models have been challenged by distinguished skeptics, including Noam Chomsky and Alison Gopnik.
Is AI Incapable of Explanation?
In an opinion piece in the New York Times, the eminent linguist Noam Chomsky and his colleagues argue emphatically that ChatGPT and its ilk operate with a fundamentally flawed conception of language and knowledge. They claim that their reliance on machine learning and pattern recognition makes them incapable of explanation (Chomsky, Roberts, and Watumull 2023):
“Such programs are stuck in a prehuman or nonhuman phase of cognitive evolution. Their deepest flaw is the absence of the most critical capacity of any intelligence: to say not only what is the case, what was the case and what will be the case—that’s description and prediction—but also what is not the case and what could and could not be the case. Those are the ingredients of explanation, the mark of true intelligence.
“Here’s an example. Suppose you are holding an apple in your hand. Now you let the apple go. You observe the result and say, ‘The apple falls.’ That is a description. A prediction might have been the statement ‘The apple will fall if I open my hand.’ Both are valuable, and both can be correct. But an explanation is something more: It includes not only descriptions and predictions but also counterfactual conjectures like ‘Any such object would fall,’ plus the additional clause ‘because of the force of gravity’ or ‘because of the curvature of space-time’ or whatever. That is a causal explanation: ‘The apple would not have fallen but for the force of gravity.’ That is thinking.
“The crux of machine learning is description and prediction; it does not posit any causal mechanisms or physical laws.”
This argument seems to be based on general ideas about machine learning, not on examination of what ChatGPT actually does. My own interrogation shows that ChatGPT is highly sophisticated in its causal and counterfactual reasoning.
I asked ChatGPT 4 what happens when someone with an apple in hand opens the hand. The program responded with a 100-word paragraph that stated that the apple will fall because of the force of gravity in accord with Newton’s laws of motion. When asked what would have happened if the hand not been opened, ChatGPT responded that the apple would not have fallen because the force from the hand would balance the force of gravity.
Even more impressively, ChatGPT gave me a fine answer to the question of what would have happened if gravity did not exist and the hand is opened. It said that the apple would not fall because without gravity there would be no force pulling it downward. ChatGPT 3.5 gives similar but briefer answers. Accordingly, Chomsky’s claims about the limitations of ChatGPT are refuted by its performance on his own example. The responses of Google’s Gemini model are similar to that of ChatGPT.
I have found that ChatGPT can not only make reasonable judgments about the truth or falsity of counterfactual conditionals, but it is also surprisingly sophisticated about how to do so. It outlines several approaches to the difficult problem of assessing the truth of counterfactual conditionals, including possible world semantics favored by some philosophers, and causal modeling favored by some AI researchers.
If you do not believe that ChatGPT is excellent at counterfactual reasoning, I suggest that you query it, for example, about what would have happened if the United States had not dropped atomic bombs on Japan in 1945.
Can AI Innovate?
Alison Gopnik is a development psychologist famous for her research on sophisticated causal reasoning in children. She and her colleagues argue that the new AI models are excellent at imitation, but are incapable of the kind of innovation that small children can do (Yiu, Kosoy, and Gopnik, 2023; Kosoy et al. 2023).
The argument is based on the failure of the large language model LaMDA (produced by Google) to accomplish a well-known causal inference task. In this task, children are able to determine which objects are “blickets” on the basis of whether they set off a machine rather than on noncausal features of shape and color.
I asked ChatGPT to solve a version of the blicket detection problem based on Gopnik’s original 2000 experiment (Gopnik and Sobel, 2000). I replaced the term “blicket” by “gooble” so that ChatGPT could not simply look up the answer from published papers. ChatGPT inferred that setting off the machine was the key feature rather than shape or color, and got the right answer about which object was a gooble.
Moreover, when asked how it reached its conclusion, ChatGPT described sophisticated causal reasoning with hypotheses about what factors might set off the machine. When queried, it reported not using Bayesian probabilities because the relevant probabilities were not available. I suspect the same is true of children.
I believe that this analysis is too subtle to have been produced through reinforcement learning by humans rather than training from examples. So I see no reason to believe that ChatGPT is merely imitative rather than innovative, especially given the many examples of creative hypothesis formation that I have found.
I personally attribute the earlier failure of Gopnik and her colleagues to find child-level causal reasoning to their use of a now-obsolete model. Google has replaced LaMDA by Gemini, with many more parameters, and it also behaves like children on the blicket test. I predict that ChatGPT 4, Gemini, Claude 3, and Llama 3 can handle the many other causal reasoning tasks that Gopnik and her colleagues have studied in children.
In my view, ChatGPT’s understanding of causality is sophisticated, even though it lacks sensory and emotional experiences that are part of human appreciation of causes and effects. But it and other advanced AI models are already exhibiting explanation, understanding, and creativity.
References
Chomsky, N., Roberts, I., & Watumull, J. (2023, March 8, 2023). The False Promise of ChatGPT. New York Times.
Gopnik, A., & Sobel, D. M. (2000). Detecting blickets: How young children use information about novel causal powers in categorization and induction. Child Development, 71(5), 1205–1222.
Kosoy, E., Reagan, E. R., Lai, L., Gopnik, A., & Cobb, D. K. (2023). Comparing machines and children: Using developmental psychology experiments to assess the strengths and weaknesses of LaMDA responses. arXiv preprint arXiv:2305.11243.
Thagard, P. (2024) Can ChatGPT Make Explanatory Inferences? Benchmarks for Abductive Reasoning. https://arxiv.org/abs/2404.18982
Yiu, E., Kosoy, E., & Gopnik, A. (2023). Transmission versus truth, imitation versus innovation: What children can do that large language and language-and-vision models cannot (yet). Perspectives on Psychological Science, 17456916231201401.