The double-edged sword of data science
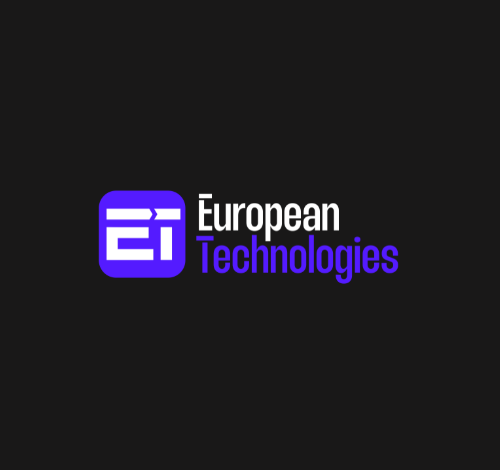
Although there is nothing new about using modelling in insurance, during the last 10 years this has increasingly involved data science.
The affordability and availability of more powerful computing, the development of new algorithms and an increase in the amount of data available have seen the usage of data science in insurance spread out from just the risk prediction element to also impact areas like marketing and claims.
For example, thanks to data science and artificial intelligence (AI), a consumer involved in a car accident can be guided by an app to take photos of their vehicle and receive a claims settlement within minutes.
But data science represents a double-edged sword.
Although it is supposed to minimise risk, data science also introduces new risks that have been growing at a faster rate than insurers’ understanding of the relevant governance and ethics.
Considering this, Pardeep Bassi, global proposition lead for data science at WTW, stressed the importance of achieving the right balance of governance and control for data science usage, while also still gaining value from its insights.
He said: “Insurers must compete, so it’s about how quickly they can understand the risk and adopt the governance and principles from [the] outset – it’s about having clearer definitions of roles and responsibilities.
“I’m not saying don’t move at speed, but make sure you understand the risks rather than go in blindly.
“Some insurers are more flexible in what they allow their data scientists to do, whereas others are more risk averse and want to fully understand the risks in a more controlled way. But around half occupy the middle ground and striking this balance makes them the most effective.”
Balance is also important when it comes to choosing the right data science algorithms to achieve a suitable relationship between accuracy and ease of interpretation.
Plus, insurers need to be able to explain to internal and external stakeholders – as well as to regulators – that they have the correct controls and ethical considerations.
Bassi highlighted that insurers which are more principles-based are setting themselves up well to deal with new regulations, such as the Artificial Intelligence Act, approved by the European Parliament in March 2024.
“The EU AI Act is a risk-based framework and therefore affects industries like insurance more,” he continued.
Relevant data and bias
While the focus for data science is often on algorithms and other tools, it is essential not to overlook whether the database due to be used is indeed relevant to the population being served.
For example, there’s no point in building models for young drivers using data from experienced drivers, or using data from rural areas for a model concerning London.
Max Ang, senior analyst in the insurance practice at Celent, noted that this is a particular issue with composite insurers because they have siloed systems.
Additionally, insurers need to be highly conscious of bias – they must pick the right definition of it and check for it before, during and after building data science models.
Tom Mansfield, data and analytics director at Axa Retail, said: “We have a pragmatic framework so we can assess individual use cases of AI and data science against a range of considerations, including diversity, non-discrimination, fairness and societal impact, privacy and data governance, transparency and accountability.”
But, even if insurers purport to be aware of these issues, there is a real risk of them being overlooked if they are moving in a hurry or if internal communications aren’t joined up.
Jamie Wilson, head of pricing and innovation at Hyperexponential, explained: “A model may deliberately exclude gender or race, but still use postcodes to charge people in inner city areas more than those in outer city areas. However, there could be a strong link between postcode and race, therefore creating inadvertent bias.
“Now [that] machine learning models can be built in a matter of minutes, there is less time to talk these things over than before, when building could take several months.”
Other preventative steps
Further steps that insurers can take to avoid these bias and relevancy problems include ensuring that data scientists work closely with others in the business, as well as subjecting models to soft launches.
Nutan Rajguru, head of analytics, claims and underwriting solutions at Verisk, told Insurance Times: “We do soft launches and let the claims handlers see the results to give us feedback, so that we can refine the model.
“We treat new models like a new member of staff and don’t let them loose until they know what they are doing.
“You also need regular reviews of models to make sure they are still up to date, appropriate and effective. Things can change in the marketplace – like inflation rates, Covid circumstances and the way that medical advances impact on personal injury claims.”
Firms that fail to take preventative steps don’t just risk failing to extract maximum value from data science. They also risk not being able to use the approach at all if people lose confidence in it.