How artificial intelligence can transform St. Louis traffic
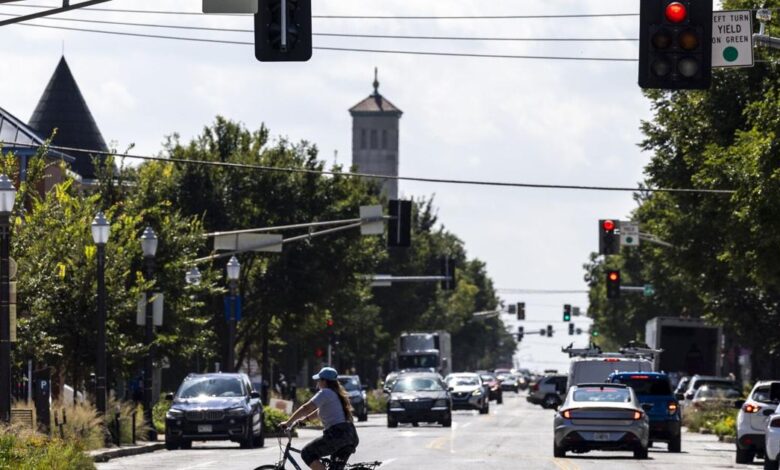
St. Louis has found a way to bring back red-light cameras, notwithstanding the earlier Missouri Supreme Court ruling holding them unconstitutional. This indicates the gravity of the problem with today’s traffic. But implementing red-light or speeding cameras is not enough. Artificial intelligence can help.
St. Louis officials have called reckless driving in the city an “urgent” problem after multiple vehicle crashes that have killed or seriously injured those in the cars and pedestrians.
AI excels in understanding massive historical data to remove or reduce uncertainties. When the traffic is more predictable, it becomes safer also as drivers and pedestrians can plan better.
Cameras used to detect speeding and running red lights are typically used in an unintelligent way. Some systems ticket people traveling above the speed limit, even briefly, but drivers who cut lanes dangerously go uncaught.
People are also reading…
A better option is for AI-enabled cameras to analyze driving patterns in real-time and ticket hazardous driving. AI-driven cameras can help identify patterns such as drivers going down wrong-way streets, speeding dangerously, running red lights and cutting lanes. Confronting those patterns could make Downtown St. Louis safer.
Coupled with the Internet of Things (IoT) technologies, AI can predict traffic jams, identify accident-prone areas and optimize signal timings. With the advent of connected vehicles and autonomous vehicles, the traffic infrastructure must be upgraded anyway to leverage the efficiencies. It makes sense to embrace changes now.
Initiatives like Google’s Project Green Light and INRIX’s Compass are already in this direction. The former analyzes traffic patterns with Google Maps data and recommends adjustments to traffic light timings, enabling shorter and more efficient commutes. INRIX’s Compass analyzes massive amounts of traffic information to identify the root causes of congestion and offer data-driven solutions.
Similar to how some security cameras detect people and vehicles, using edge computing, where the computation happens locally in the device, traffic cameras can identify drivers and vehicles involved in dangerous driving. By training the AI models in the camera with data on dangerous driving patterns and inadvertent or unavoidable maneuvers, false positives can be reduced.
Using advanced object detection techniques in AI, vehicle trajectories, traffic density, and accidents can be monitored in real-time. It should be the driving patterns that should be penalized, and not by blunt metrics like speed limits or turning right on red. AI can not only help vehicles but also bikes, bicycles and pedestrians — everyone using the roads.
AI can detect abnormalities such as a runaway object or person on a freeway and help with the investigation of accidents. For instance, AI can analyze the audio in the hours of the camera footage and pinpoint the exact timeframe of the accident in the video based on the sound of a crash.
Given that AI-enabled computer vision is near human-level performance, it is high time that the signaling was dynamically based on traffic movement rather than on induction loops, infrared sensors or the older technologies in practice today.
For instance, the signal often turns green even when the car across from the signal stops to make a right turn when a right turn on red is legal. Each minute counts in the rush hour and delays impact the driver’s health, driving behavior, timeliness, and therefore safety. The impact of driving in traffic has been studied in the past. Driving longer impacts metabolism and adversely affects health. Wait time at the intersection contributes to this problem.
There is substantial research into intelligent traffic signaling systems and using AI for traffic management. It is time that the research is translated into practice. Cities may be constrained by the availability of resources and skills in doing so, but the return on investment certainly justifies the prioritization.
Public health departments and sustainability advocates should push cities in the direction of saving lives and limbs by avoiding accidents through efficient traffic management and saving health.
To be sure, concerns remain with the use of AI, particularly regarding privacy. Availability of datasets to train the models, and technology limitations in uncertain weather and lighting are some other concerns.
Nevertheless, the AI juggernaut moves on and should take traffic management with it along the way. Let us facilitate a traffic flow that is smart, safer, and sustainable.
Pendyala teaches machine learning and other data science courses at San Jose State University and is a Public Voices Fellow of The OpEd Project.