The Beijing dilemma: Dependencies in global artificial intelligence research
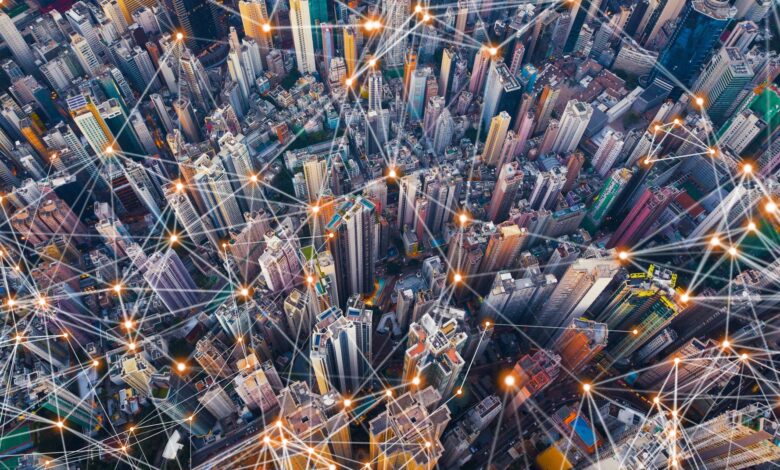
A common view of the relationship between the U.S. and China regarding artificial intelligence (AI) is of competition or even a battleground. However, artificial intelligence is a scientific field that thrives on collaboration and knowledge sharing. As a science, it relies on previous knowledge from all corners of the world. These views pose a dilemma. On the one hand, some developments in AI technologies must be kept secret for national security reasons. On the other, purposeful AI technologies in, for example, medical diagnosis or education may be slowed down because useful foreign knowledge is not accessed. In a recent paper, we take a step toward addressing this dilemma and describe AI knowledge dependencies worldwide (AlShebli et al. 2022). If dependencies are important, depriving the U.S. of AI knowledge created elsewhere can hamper social and economic development.
We document how AI researchers in given cities base their AI work on knowledge produced in other cities across the world (AlShebli et al. 2022). We study cities because they have driven innovation, and their inhabitants have consumed most of the wealth created by the new technologies used in production since the beginning of civilization (Montgomery 2008; Glaeser 2011; Dal Bó et al. 2022). As economic growth in the long run is determined by people searching for ideas, cities with large numbers of people, such as those in China and India, are likely to become future drivers of growth (Jones 2023).
Our work investigates inter-city dependencies on AI research by analyzing more than two million AI papers published over decades. We compare cities regarding their AI research, focusing on how many papers they produce, how many citations they receive, and how many AI researchers they house. We find that Beijing has been the most productive city since 2002, the most impactful since 2007, and the city with the largest AI workforce for more than two decades. Beijing is also the city that by far connects more AI knowledge and human capital through migration and collaborations between East and West.
While the public-good nature of AI research facilitates its widespread adoption, it also hinders the exclusion of firms and governments with opposite interests. For example, a discovery in AI in one country can spill over to a rival country because knowledge usually flows more easily than tangible goods. Still, eschewing AI knowledge production because it is a public good is not a solution for countries (like the U.S.) that are uncomfortable with the national security implications of such technologies. The reality is that even advanced countries must tap into knowledge and talent from wherever talent and knowledge are available if they are to lead the advance of the field. Research productivity across fields is already falling in the U.S. (Bloom et al. 2020), and nations with many people have the potential to grow knowledge. Authorities are aware of this. In May 2023, the U.S. National Artificial Intelligence R&D Strategic Plan made explicit the importance of the federal government establishing a principled and coordinated approach to international collaboration in AI research (National Science and Technology Council 2023). Enhanced collaboration may start with influential foreign cities in countries that espouse similar values, such as Seoul, London, Paris, Munich, and Sydney. Cooperating with nations that have opposite geopolitical interests is difficult at best. However, Beijing’s influence must not be overlooked. While collaborating in AI technologies leading to any military application must be prohibited, opening conversations to ensure safety and agreement on ethical standards could be allowed. As the director of the White House Office of Science and Technology Policy, Arati Prabhakar, acknowledged, a good place for collaboration with Beijing is to understand how to make “a technology that is safe and effective.” After all, there is increased demand for a better understanding of AI ethics and for oversight of AI research conducted abroad (Jobin, Lenca, and Vayena 2019).
Tracking Beijing’s influence on AI research
Our study examines the global influence of leading AI-research cities. We use the Microsoft Academic Graph (MAG) dataset retrieved on September 12, 2020. This dataset includes records of conference and journal papers and their attributes such as publication date, venue, and discipline as well as the authors’ names, affiliations, and the network of citations.1 Our study focuses on conference and journal papers published between 1990 and 2019 (inclusive) that fall under AI according to MAG. The last author is typically the principal investigator in computer science and “the head of the lab that hosted most of the research” (Venkatraman 2010), so we use the last author’s affiliation as the geographical location associated with the paper. We identified the city corresponding to the last author’s affiliation using the Google Map Geocoding API. We classified about 2.2 million AI papers to cities using this method. The three outcome measures we use to quantify each city’s scientific output are: impact, productivity, and number of AI scientists. Impact is the number of citations that papers from the city have accumulated within the first two years post-publication, productivity is the number of AI papers that the city has produced each year, and the number of AI scientists counts AI researchers affiliated with an institution in the city under consideration.
To assess the relative strength with which different cities influence the global AI landscape, we perform a geographical analysis inspired by the standard gravity models from the international trade literature (Anderson and Van Wincoop 2003; Santos Silva and Tenreyro 2009). We consider the three metrics just described—impact, productivity, and number of AI scientists, to describe the geographical evolution of AI research over time. For each metric and each year starting from 1990, we compute the center of mass, which is a weighted average of each city’s geographic location (latitude and longitude). The weights are each metric’s value in that city. A center of mass in this context concentrates the influences the world’s cities exert on one another into a single geographic point. Each of those three centers of mass has moved eastward since 1990. Beijing has exerted a significant pull, see Figure below.
How do Western cities depend on Eastern cities and vice versa?
To answer this question, we first count sources of paper references. Our analysis reveals home bias: All cities reference their work more frequently than others. Furthermore, focusing on the cities with the most citations globally from 2013 to 2017, which we label the top 20 cities, we find that the most cited Eastern cities (all world cities excluding American and European cities), including Beijing, have a relatively modest influence on top Western cities (American and European cities). Moreover, Beijing cites other cities the most, irrespective of whether they are in the East or West. In other words, Beijing, the largest producer of AI knowledge, is also the largest consumer, and its demand for AI knowledge spans Eastern and Western cities.
But Beijing’s sheer volume of papers requires creating a benchmark that accounts for Beijing’s size. To do so, we compare each city’s actual citations to a baseline model in which citations would occur randomly. If researchers cite randomly, the share of citations a city receives should correspond with its share of papers in the global pool. Researchers do not cite randomly, so deviations from the random baseline indicate a preference or aversion toward a specific city’s research. We find a marked inclination for Western cities to cite other Western cities with a frequency that surpasses what would be expected by chance. However, Beijing’s influence in the West is not commensurate to its size, as Western cities cite Beijing less frequently than expected. These findings reveal Western cities prefer to cite Western cities’ AI papers, while Eastern cities—Beijing in particular—favor both Eastern (notably, Singapore and Hong Kong) and Western cities’ AI papers. After accounting for size, the most preferred cities in the world are Mountain View, CA, Stanford, CA, and Redmond, WA.
Human capital flows between cities
Knowledge also diffuses through people, especially the part of knowledge that cannot be codified. To study this aspect, we identify AI scientists as those who: 1) have published at least three papers, and 2) at least half of their publications are in the field of AI, according to the Microsoft Academic Graph. For each pair of cities, we count the AI scientists who moved from one to the other at any point during the five years between 2015 and 2019. We also count those who stayed in the same city within that same time period. We find that the greatest migration of AI scientists occurs between Eastern cities. In addition, most AI scientists in any given city in 2015 remained in that city in 2019. However, Beijing attracted the most scientists from, and supplied the most scientists to, the other top 20 cities.
Inter-city teams can also create knowledge through collaborations. Collaborations between two cities in the five years between 2015 and 2019 are measured as the number of papers in which the last author is in one city and at least one coauthor is in another. We find that, just as with impact and migration, Eastern cities collaborate conspicuously. Western cities also collaborate but to a lesser extent. Collaboration is less frequent between the East and the West than within the East or within the West. Still, Beijing appears to be a bridge between Eastern cities and a few Western ones, especially Redmond, WA, Cambridge, MA, London, and Pittsburgh, PA.
The world of AI research seems to be bipolar in that citations and talent mostly flow within Eastern and within Western cities but hardly between them. This raises the question of whether Beijing is really a bridge between East and West. To answer this question, we propose a version of betweenness centrality. Betweenness centrality measures how many shortest paths from one city to another pass through a third city in the global network of cities. The measure we use counts the shortest paths of citations from East to West or West to East that pass through each city in the world. We analyze the citations network, the migration network, and the collaboration network. Beijing is the city that sees most of the shortest paths passing through it. In other words, Beijing is the largest bridge between East and West: Its betweenness is far greater than any other city, regardless of the network under consideration. Such a bridge is not too broad, but it is conspicuous.
In sum, our results suggest that size matters. Beijing influences other cities by its size—the number of researchers it has and the number of papers it produces. There is almost a one-to-one relationship between citations and the number of papers produced. When looking at the migration of scientists, the number of AI scientists at a destination is strongly and significantly associated with migration between cities. Likewise, the number of AI scientists at a source is also associated with migration between cities, suggesting that cities housing many AI researchers supply more scientists to the world, as one would expect.
Policy implications
One lesson from our paper is that size matters for AI research productivity and influence, so a simple policy should incentivize more local scientists, their communities, and employer organizations to engage in rigorous AI publication. More AI scientists working to advance the field are associated with more total citations, collaborations, and talent migration. As countries increasingly adopt industrial policies, AI research presents itself as a justifiable investment. AI knowledge creation is a public good emerging upstream on the value chain, potentially bolstering a broad range of technologies such as chip design and production. A key question is whether public funding should go to private firms or remain inside university and government-sponsored research labs. In this regard, the U.S. has a successful history of funding research through both universities and firms, especially the young and innovative (Mowery 2009). However, it is important to remember that a balance between industry and academic AI research is warranted. Industry’s natural advantages in acquiring the inputs needed for frontier AI research, such as large datasets, computing power, and talent, have increased its dominance in AI knowledge production (Ahmed, Wahed, and Thompson 2023). The prominence of Redmond, WA, the city home to Microsoft’s headquarters, as one of the leading locations in terms of AI impact in the world attests to this phenomenon.
Another lesson is that more research builds bridges. Cities in the West or the East other than Beijing (such as Seoul, Singapore, and Sydney) could also serve as bridges of citations, collaborations, and migrations. Many hubs have the potential to infuse diversity in terms of researchers’ expertise and interests. Large pools of scientists collaborating and migrating to or from different hubs increase scientists’ exposure to new ideas and the chances of disruptive discoveries. The literature on the agglomeration of economic activity offers clues on creating these hubs (e.g., Glaeser 2010; Balland 2020). Fostering global hubs for AI requires better salaries and perks and a critical mass of talented scholars and practitioners. Geographical hubs of AI other than the San Francisco Bay area and the Boston-Cambridge hub are developing in the U.S., such as in Redmond, WA, and Pittsburgh, PA.
While replicating the size of major Chinese cities and Beijing’s central role within the AI network is likely unfeasible from a policy perspective, acknowledging the importance of the sheer number of papers and scientists can guide Western research efforts. Further incentivizing training and retaining AI scientists across cities in the U.S., for example, aligns with the purpose of the CHIPs and Science Acts of forming national microelectronics training networks and fostering innovation, especially at the startup level. These initiatives are currently focused on microelectronics, but they could be extended to forming scientific AI networks that complement the advance of semiconductor design and manufacturing while accelerating breakthroughs in AI. Apart from the complementarities between semiconductors and AI, the wide applicability of AI knowledge can reduce the usual concern that laws promoting selected industries just “pick the winners’’ at the expense of all the rest—the usual criticism about industrial policy.
Our research suggests that the Western world, particularly the U.S., should find ways to contribute and benefit from the opportunities emerging in other countries. In May 2023, the U.S. National Artificial Intelligence R&D Strategic Plan added a new strategic pillar to the original eight that recognizes the importance of the federal government establishing a principled and coordinated approach to international collaboration (National Science and Technology Council 2023). The strategy aims at nations that share our values with respect to democracy and human rights. In our study, among the top 20 cities in terms of AI impact (number of citations), five cities are located in such countries: London, Munich, Paris, Seoul, and Sydney. We could consider Singapore, too. However, the other international cities among the top 20 are under China’s domain: Beijing, Shanghai, Nanjing, Wuhan, and Hong Kong. The case for cooperation with nations espousing different values is difficult, but their influence, particularly that of Beijing, must not be overlooked.
A starting point for these more difficult conversations is addressing the demand for a better understanding of AI ethics, standards, and socio-economic implications (Jobin, Lenca, and Vayena 2019). For example, Arati Prabhakar, the director of the White House Office of Science and Technology Policy, indicated that “steps have been taken to engage in that process” of collaboration because it seems that “the one place we can all really agree is we want to have a technology base that is safe and effective.” Opening discussions with antagonistic countries requires a delicate assessment of national security issues, but doing it right could afford global connection from a relatively safe position, increase public scrutiny of the technologies, and boost awareness of the potential benefits and harms of the discoveries that happen elsewhere in the world. Private American AI business executives and experts are already engaging—sometimes secretly—in conversations with their Chinese counterparts. Those conversations should take place within an official frame, spearheaded by high-level government officials who represent the interests of all the American people.