Navigating the ethical landscape of artificial intelligence in radiography: a cross-sectional study of radiographers’ perspectives | BMC Medical Ethics
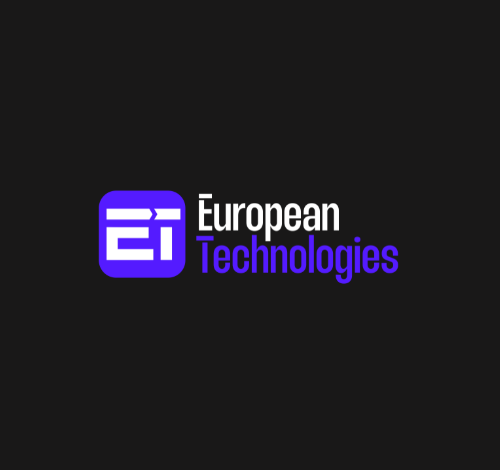
The aim of this study was to explore radiographers’ perspectives on the ethical implications of AI in their field and identify their key concerns and potential strategies for addressing them. The results presented in this paper provide a comprehensive overview of radiographers’ perspectives on the ethical landscape of AI in radiography. These findings highlight the varying levels of familiarity, trust, and preparedness among radiographers, thereby emphasizing the importance of addressing ethical considerations in the integration of AI technologies in radiographic practice.
The integration of AI into radiography represents a transformative shift in medical imaging, offering unprecedented opportunities for improved diagnostics and workflow efficiency. However, AI technologies have become increasingly prevalent; therefore, ethical considerations surrounding their implementation have become paramount. This cross-sectional study examined the perspectives of radiographers, who are crucial stakeholders in the use of AI in medical imaging and sheds light on the ethical landscape and provided insights for the responsible development and implementation of these technologies.
Understanding radiographers’ perspectives
The findings of this study revealed a nuanced understanding of AI among radiographers, reflecting varying degrees of familiarity and preparedness. Although some radiographers demonstrated a high level of awareness of and trust in AI, other radiographers were hesitant and expressed concern. These varying perspectives can be attributed to the differences in education, training, and exposure to AI technologies in professional environments. Similar studies [10, 11] reported comparable results, highlighting diverse perspectives among radiographers on the adoption of AI technologies. Some radiographers acknowledged the potential of AI to improve diagnostic accuracy and workflow efficiency. In contrast, others were apprehensive about possible job displacement and the need for further training.
Considerable debate exists regarding the potential impact of AI on the radiography sector [12]. A study by Hardy and Harvey [12] examined the potential impact of AI on the radiography profession by analyzing current workflow and identifying areas where AI automation such as protocol planning, image acquisition, and processing may be implemented. This study provided a comprehensive understanding of the practical use of AI in radiography. Furthermore, a study [9] conducted in Saudi Arabia examined radiographers’ perspectives on the use of AI in diagnostic imaging. This qualitative research focused on the specific challenges and concerns that radiographers face when incorporating AI into their profession. It also provided valuable recommendations for the development and advancement of radiography.
The integration of AI into the field of radiology has elicited considerable interest and concern within the radiographer community. The utilization of AI techniques has exhibited a remarkable ability to autonomously discern intricate patterns within imaging data, thereby facilitating the provision of quantitative evaluations pertaining to radiographic attributes [13].
The advent of AI in the field of radiology has elicited apprehension among radiographers, as highlighted by Abuzaid et al. [8]. Nevertheless, the lack of comprehensive data pertaining to the viewpoints of radiographers regarding the integration of AI within the realm of radiology is imperative to acknowledge, as expounded by Rainey et al. [14] in their recent study. Comprehending radiographers’ attitudes and perceptions regarding AI is of great importance because of their indispensable role in facilitating the effective integration of AI advancements within the field of radiology, as highlighted by Chen et al. [15]
As the frontline users of AI applications in radiography, radiographers have a pivotal role in shaping the ethical dimensions of radiography implementation. Their perspectives on patient privacy, data security, bias, and transparency offer valuable insights into the practical challenges faced during the integration of AI into daily practice.
Ethical considerations in AI-assisted radiography
The ethical considerations highlighted in this study align with broader discussions in the literature. Patient privacy and confidentiality have emerged as significant concerns, echoing the findings of studies more broadly focusing on AI in healthcare [16]. Ethical considerations regarding the use of AI in radiography have a vital role in ensuring patient safety, respecting privacy, and promoting equitable healthcare delivery. The ethical and professional implications of incorporating AI into radiology have been carefully scrutinized. Currie et al. [3] argue that the ethical application of AI in radiology should prioritize patient well-being, minimize harm, and ensure an equitable distribution of benefits and risks among stakeholders. A thorough comprehension of the ethical guidelines and principles regulating AI in healthcare, specifically in radiography, is essential [17].
The ethical considerations related to the integration of AI into radiography are multifaceted and encompass a range of crucial aspects. These include the preservation of patient data privacy, ensuring the confidentiality of sensitive information, and addressing complex issues of data ownership. Furthermore, the development and utilization of AI in healthcare must be approached within a strong ethical framework to ensure that its implementation aligns with established ethical principles and guidelines [18]. A comprehensive examination of AI precision, ethical quandaries, and predispositions, along with the conceivable effects of disparity in discriminatory practices and legal liabilities that may arise from the integration of AI technology within the domain of radiography, is necessary [3, 19]. Moreover, ethical concerns associated with the design and deployment of AI in the healthcare domain is important to emphasize. This highlights the necessity of incorporating ethical considerations into the developmental process of AI and advocating the establishment of comprehensive frameworks to facilitate such integration [20, 21].
Insights from radiographers regarding the ethical implications of AI in radiology are vital for the effective implementation of AI advancements in radiological procedures. Understanding and addressing these ethical problems are crucial to ensure the conscientious and ethical use of AI in radiology. Continued evaluation of the issue is crucial as the understanding of the effects and capabilities of AI grows and is crucial to ensure that AI tools adhere to revised ethical regulations and guidelines [22].
Bias and fairness in AI algorithms have been recognized as critical issues in various domains. This study’s findings emphasize their relevance in the context of radiography. Radiographers recognize the potential for bias in algorithmic decision-making and express the need for ongoing efforts to address and mitigate these biases. The issue of bias in algorithmic decision-making in radiography is a crucial subject that has received significant attention in recent research. Algorithmic decision-making is believed to be driven by the concept that algorithms, unlike humans, make decisions without considering the specific attributes of the person being evaluated [23]. Lee [24] suggested that the perception of fairness and trust in algorithmic decisions is influenced by different traits associated with human and algorithmic decision makers. The possibility that algorithmic clinical predictions contribute to health disparities is a concern, which highlights the importance of assessing algorithmic bias and fairness in healthcare decision-making and prediction [25].
Cognitive and systemic factors in radiology influence the occurrence of diagnostic errors. Cognitive biases such as anchoring, framing, and premature closure have been identified as particularly prone to errors in the interpretation of radiological findings [26]. Moreover, an analysis conducted by Pot et al. [27] sheds light on the existence of fair and unfair biases within the machine learning and radiology domains. This finding underscores the significance of actively addressing biases existing in datasets and in algorithms. Furthermore, acknowledging the pivotal contribution of the ACR Data Science Institute (Reston, VA, USA) to promoting health equity in the field of radiology is crucial. This acknowledgment highlights the profound clinical implications associated with the inadvertent bias that may arise from the use of AI visualization algorithms in radiological practice, as discussed by Allen and Dreyer [28,29,30].
This study also underscores concerns related to the transparency and explainability of AI systems. Radiographers expressed unease with the “black box” nature of these algorithms, emphasizing the importance of understanding how AI reaches its conclusions. This lack of transparency can affect radiographers’ trust in AI systems, which aligns with the findings of studies focusing on trust in AI in healthcare.
Implications for practice and policy
The insights gained from this study have practical implications for integrating artificial intelligence into radiography. Ethical guidelines and educational programs should be tailored to address the specific concerns and perspectives of radiographers. Transparent communication regarding the development, validation, and deployment of AI algorithms is essential for building trust among radiographers and for ensuring their active engagement and collaboration with AI technologies.
Moreover, this study’s findings underscored the need for ongoing professional development opportunities to enhance the preparedness of radiographers for the evolving AI landscape in radiography. As AI technologies continue to advance, continuous education and training programs will empower radiographers to effectively navigate ethical challenges and contribute to the responsible use of AI in healthcare.
Limitations
This study acknowledges certain limitations, including the reliance on self-reported data, potential selection bias in the convenience sample, and inherent subjectivity associated with qualitative data analysis. These limitations are discussed in the interpretation of the results.