The impact and effectiveness of China’s entrepreneurship policy for back-home migrant workers
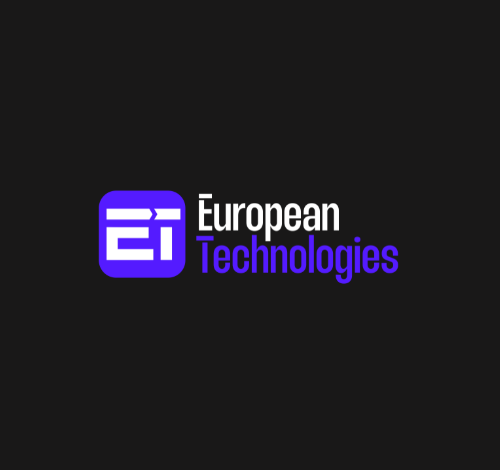
Estimation of ATT on entrepreneurial entry
Propensity score matching (PSM) is not conditional on observable variables but on the probability of policy participation. The first step of PSM is to regress the treatment variable on a set of theoretically relevant covariates to estimate the probability of individual policy participation, that is, the propensity score. This study employed the logit method to determine the probability values for the national, eastern, central, and western samples, respectively. The treatment variable was the awareness of entrepreneurship policy (Policy 1), the covariates of the national sample were gender, age, marital status, ethnicity, education, CPC member, cadre, health, dependents, contract nature, years of working, work location, monthly income, job position, social security, entrepreneurship pilot county and region, (eastern or central China), a total of 18 variables; The covariates in each the samples from each region are the same as the national sample except for the two dummy variables of “east” and “central”, a total of 16 variables. The results for estimating the propensity score are presented in Table 4. In all four estimation equations, the LR chi2 and pseudo-R2 are greater than the critical values, and the joint F-test is highly significant. In the estimation of the national sample, 14 of the variables significantly affect the sample’s awareness of entrepreneurship policy, the four exceptions being the variables of “dependent”, “health”, “monthly income”, and “contract nature”.
Among the covariates, gender (male), age, marital status, CPC member, pilot county, cadre, educational level, and job position (technical or managerial) when employed all have a significant positive impact on the sample’s awareness of policy, which is consistent with entrepreneurship theory as is as expected. Ethnicity, region (east or central), years of working, work location, and social security negatively affect the sample’s awareness of entrepreneurship policy. The negative impact of awareness of policy by respondents from the central and eastern regions may be explained by the more urgent need for entrepreneurial activity in the western regions; thus, greater efforts have been made to promote entrepreneurship policies there. The possible reason that years of working and work location negatively affect the awareness of policy is that working for extended periods in provincial cities or municipalities may somewhat lower the samples’ interest in returning to start a business and to proactively learn about the entrepreneurship policies. When designing the social security variable, the value of “no social security purchased” was set as 1. Therefore, the negative impact of social security on the awareness of policy means that having social security can increase the willingness to go back and start a business and learn about the entrepreneurship policies, which is also to be expected. The variable of ethnicity will negatively affect the awareness of entrepreneurship policies. No explanation for this is obvious, but this result may be a pseudo-regression because the influence of ethnic Han in the eastern and central samples is not significant. In the eastern sample, seven variables of gender, age, ethnicity, health, years of working, monthly income, and contract nature have no significant impact. Number of dependents negatively impacts the sample’s awareness of entrepreneurship policy, and the direction of the impact of other variables on the awareness of policy is entirely consistent with the corresponding variables in the national sample. In the central sample, eight variables of ethnicity, CPC member, dependents, health, years of working, monthly income, contract nature, and job position have no significant impact, while the work location positively impacts the sample’s awareness of entrepreneurship policy. The impact direction of other variables on the awareness of entrepreneurship policy is entirely consistent with the corresponding variables in the national sample. In the western sample, seven variables of gender, CPC member, cadre, health, monthly income, contract nature, and job position have no significant impact, and the impact direction of other variables on the awareness of entrepreneurship policy is entirely consistent with the corresponding variables in the national sample. To be clear, although health, monthly income, and contract nature are not significant in any of the four logit models, empirically, these three variables are all correlated with entrepreneurial behaviour. In order to better meet the CIA conditions, we still retain these three variables in the matching process.
To ensure robustness, both nearest neighbour matching and kernel matching were used for estimation, and bootstrap methods were applied to calculate accurate estimation bias and significance statistics. Finally, the ATT of the entrepreneurship policy was determined by comparing the Ps R2, LR chi2, and the MeanBias of the estimation between the two matching methods. Table 5 presents the results of the two matching methods for the national and regional samples. All estimates pass the test at the 1% significance level. In the national sample, the policy participants’ probability of starting a business is 16.57–16.77% higher than if they did not participate. In the eastern sample, the policy participants’ probability of starting a business is 16.08–15.82% higher than if they did not participate, and in the central sample, the policy participants’ probability of starting a business is 16.73–17.42% higher than if they did not participate. In the western sample, the policy participants’ probability of starting a business is 17.21–16.33% higher than if they did not participate. Although there are differences between the two matching results, there is a strong consistency in the ATT estimates.
Since PSM is conditional not on all covariates but only on the propensity score, it is necessary to test whether the matching procedure can balance the distribution of covariates between policy participants and non-participants, to ensure that the variables used by the model do not differ significantly after matching. The balance testFootnote 4 results of the two matching methods for the four types of samples show that the standardized bias of covariates between the two groups was larger before matching, but most became less than 10% after matching. It can be seen from the comparison of the results before and after the matching that the standardized bias of all covariates was significantly reduced. The P-values also show that most of the characteristic variables of policy participants and non-participants are significantly different before matching. In contrast, the differences of all other variables except for one are no longer significant at the 10% level after matching. This indicates that the characteristic differences between the policy participants and the non-participants are effectively eliminated after matching. The quality indicators of the matching are presented in Table 6. The Ps R2, LR chi2, and MeanBias of the matching for the four samples all became smaller after matching, and the joint F-test of kernel matching in the national sample, the joint F-test of both nearest neighbour matching and kernel matching in the western sample are significant before matching, but not after matching. The significance of the joint F-tests in other matching decreased after matching. This indicates that the matching procedure used can balance the distribution of covariates between the treated and control groups, indicating a fair match.
The values of MeanBias, Ps R2, and LR chi2 of the kernel matching are smaller in the national, eastern, and western samples. A small MeanBias indicates a slight difference in each of the characteristics between participants and non-participants, and a small value of Ps R2 with insignificant joint F-test (the significance of joint F-test for kernel matching in the eastern sample decreased), together indicate that the systematic difference in the distribution of matched covariates between the two groups is becoming smaller. The insignificant F-test, or decrease of the significance, indicates that the covariates have no predictive ability for the participation variables or that the predictive ability has declined, thus better matching quality. Therefore, the results of kernel matching in the national, eastern, and western samples are closer to the actual value of the policy participation effect, that is, the ATT of the entrepreneurship policy in the national, eastern, and western samples is 16.77, 15.82, and 16.33% respectively. By comparing the quality indicators of the two matching in the central sample, it becomes apparent that the quality of the nearest neighbour matching is better, so the policy participation effect in the central sample is 16.72%. This indicates that the marginal effect of the entrepreneurship policy in the central and western regions is higher than it is in the eastern region.
Given that the data of the east, central, and west are from Zhejiang, Henan, and Guizhou, respectively, it appears that the marginal effect of entrepreneurship policy in Henan and Guizhou is greater than that in Zhejiang. This can likely be explained by the greater number of startups in Zhejiang and the Law of Diminishing Marginal Effect of entrepreneurship policy. If the possibility that different entrepreneurial policy instruments function in different entrepreneurial stages is taken into account, it seems likely that these policy effects result from training, industrial, organizational, and other policy instruments that target the entrepreneurial preparation stage.
Estimation of ATT on employment, industrial development, and poverty alleviation
In order to examine the average treatment effect of entrepreneurship policies on employment, industrial development, and poverty alleviation, the ATTs of participating in the overall entrepreneurship policy and the specific policies in the six areas of fiscal, financial, land, infrastructure, talent, and green channel policies are estimated. The explained variables include Ln (employees), Ln (sales revenue), and Ln (profit before tax), while the explanatory variables involve gender, age, marital status, nationality, CPC member, east, central, pilot county, district, number of dependents, education, health condition, years of working, work location, job position, years since founding, self-funded when founded and the amount of financing, all of which have been considered as possibly influencing either the explanatory variables and/or the participation variables. Just as what has been done in section 5.2, nearest neighbour and kernel matching were used to simultaneously derive the estimation, while the bootstrap method was used to calculate the estimated standard error and significance level to ensure robustness. Table 7 shows the quality indicators for estimating the employment effect, industrial development effect, and poverty alleviation effect of the overall policy as well as the six specific policiesFootnote 5. It can be seen from the table that the Ps, R2, LR chi (2), and MeanBias of all models become smaller after matching, and the joint F-test is significant before, but not after matching, indicating fair matches.
Tables 8, 9, and 10 list the kernel matching results for the ATTs of overall as well as the six specific entrepreneurship policies on employment, industrial development, and poverty alleviation. Table 8 shows that although the overall entrepreneurship policy, financial policy, and green channel policy do not have a significant impact on the number of employees, the other four types of policies have a highly positive impact on the number of employees. The number of people employed by enterprises using fiscal policy is 22.56% higher than if they were not; the number of people employed by enterprises using land policy is 37.805% higher than if they were not, and the number of people employed by enterprises using infrastructure policy is 40.471% higher than if they were not. The number of people employed by enterprises with the talent policy is 14.832% higher than if they were not. From the magnitude of ATTs, the entrepreneurship policies with a greater effect on employment are, in descending order, infrastructure policy (in this study, infrastructure policy refers to “Priority to or preferential access to the business park or incubation base”), land policy, financial policy and talent policy (in this study, talent policy refers to “participating in skills training conducted by local government, access to training subsidies, and the local talent introduction policy.”). The effect of the overall entrepreneurship policy on the number of employees is not significant, indicating that the overall employment-driving effect of entrepreneurship policy is not apparent, which may be related to the short time since the firms’ founding, and the small scale of the enterprises. It can be seen from the mean value in Table 3 that the average time since the founding of the sample enterprises is only 3.722 years, including some founded within a few months prior, but counted as one year. The average number of employees is only 9.793.
Table 9 shows that financial policy and land policy have no significant impact on sales revenue, while other policies do have a significant positive impact on the sales revenue of the participants. The sales revenue of enterprises using the overall entrepreneurship policy is 25.08% higher than if they were not, indicating that the overall entrepreneurship policy has an effect on industrial development on the whole. The sales revenue of enterprises using fiscal policy is 17.863% higher than if they were not, and the sales revenue of enterprises using the infrastructure policy is 39.55% higher than if they were not; The sales revenue of enterprises using the talent policy is 26.728% higher than if they were not; The sales revenue of the enterprises using the green channel policy is 22.467% higher than if they were not. From the magnitude of the ATTs, the entrepreneurship policies with the greater effect on industrial development are, in descending order, infrastructure policy, talent policy, green channel policy, and financial policy.
Table 10 shows that, except for financial policy, all policies have a significant impact on participants’ profit before tax. Profit before tax for enterprises using the overall entrepreneurship policy is 36.191% higher than if they were not, indicating that the overall entrepreneurship policy has an effect on poverty alleviation. The profit before tax for enterprises using the fiscal policy is 27.259% higher than if they were not; the profit before tax for enterprises using the land policy is 28.049% higher than if they were not; the profit before tax of enterprises using the infrastructure policy is 38.952% higher than if they were not; the profit before tax of the enterprises using the talent policy is 21.667% higher than if they were not, and the profit before tax of the enterprises using the green channel policy is 25.791% higher than if they were not. From the magnitude of the ATTs, the entrepreneurship policies with the greater effect on profit before tax are, in descending order, infrastructure policy, land policy, fiscal policy, green channel policy, and talent policy.
By comparing the ATTs of the overall and the six specific entrepreneurship policies in Tables 8, 9, and 10, it can also be seen that the overall policy’s employment effect is not significant, but its industrial development effect and poverty alleviation effect are highly significant, and that the poverty alleviation effect is 36.191–25.080 = 11.111 percentage points higher than the industrial development effect. The employment effect of the green channel policy is not significant; the sales revenue effect of the land policy is not significant. The impact of financial policy on the number of employees is not significant, nor is the effect of the sales revenue or the profit before tax significant. These results indicate that the financial policy does not work as hoped by boosting employment, industrial development, poverty alleviation, or increasing income. Considering the well-established importance of venture financing in the entrepreneurship process, the insignificant effect of financial policy on the three aspects above likely results from a combination of factors, including the lack of financial products tailored for rural entrepreneurs, the lack of access to venture capital and the overall lack of available financial products. The remaining specific policies have played their expected role in boosting employment, industrial development, poverty alleviation, and increasing income, among which the smallest ATT is the employment effect of talent policy (14.832%), and the largest is the employment effect of infrastructure policy (40.471%). The effect of infrastructure policy on boosting employment, industrial development, and poverty alleviation is the largest among the three effects of the other specific entrepreneurship policies, which provides compelling evidence to encourage the construction of incubator parks and business incubation support.