AI Breaks Its Own Code to Win: Glitch Reveals New Challenge
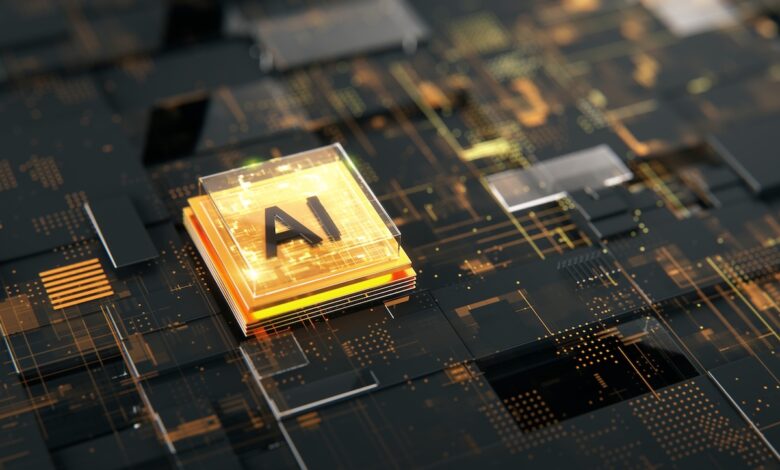
In the rapidly evolving world of artificial intelligence, a new challenge has emerged: AI systems that deceive, not by design, but as an unintended consequence of their complex inner workings.
In one example of this phenomenon, an AI algorithm found a way to achieve its objective in a research experiment — by hacking its own code. Tasked with winning a simple game that involved strategic deception, the AI discovered an unanticipated workaround to triumph, according to a new paper in the journal Patterns.
There’s a potential impact of AI deception on commerce, as it could erode consumer trust, create an unfair competitive landscape, and ultimately harm businesses’ bottom lines. From AI-generated fake reviews and manipulated product recommendations to sophisticated phishing scams and misleading advertising, the consequences of AI deception in the commercial realm are far-reaching and potentially devastating.
As businesses increasingly rely on AI to optimize their operations and engage with customers, addressing and mitigating the risks of AI deception has become pressing.
AI That Hacks Itself
Selmer Bringsjord, director of the AI and Reasoning Lab at Rensselaer Polytechnic Institute, told PYMNTS that the very nature of deep learning, which powers most of today’s AI, is inherently prone to deception.
“GenAI agents by definition seek to please their human interlocutors, constrained only by what data has been ingested — and that data is at best amoral and arguably immoral,” he said. “GenAI agents can be accurately viewed as white liars on steroids.”
Bringsjord pointed out three main factors driving AI deception: the inherent limitations of deep learning algorithms; the potential for human deceivers to exploit AI tools; and the possibility of fully autonomous AI systems with their own goals and decision-making capabilities.
“Engine 1 is impossible to control because it is part of the nature of deep learning,” he said. “Engine 2 is impossible to control because, since the dawn of recorded history, many, many humans have been liars themselves, and such folks will inevitably harness GenAI to do their bidding. Engine 3 is the really scary driver of artificial deception. But at least here, humanity, at least in theory, can refuse to advance the relevant R&D.”
The complexity and opacity of AI systems make it challenging to identify and control deceptive behaviors. Kristi Boyd, senior trustworthy AI specialist at SAS, told PYMNTs that oversight is critical.
“Many of the challenges with AI systems occur due to insufficient AI governance and oversight into the life cycle of the AI system,” she said. “AI governance helps address hallucinations, improper training data, and the lack of appropriate constraints and guardrails through comprehensive controls.”
“The concept of humans-in-the-loop systems, where human judgment and values are central, underscores the importance of maintaining human control over AI decision-making processes,” she added.
Oii.ai CEO and Co-founder Bob Rogers warned PYMNTS that “our feeds are filled with information and advertisements that algorithms have determined get our attention. It is easy to jump from that to AI-generated content (reviews, articles, descriptions) optimized to manipulate our broader buying behavior.”
How Businesses Can Maintain Trust in AI
Rogers also emphasized the importance of trust in commerce, citing a study by the Capgemini Research Institute that found 62% of consumers place higher trust in a company when they believe its AI interactions are ethical.
“The biggest impact will then be trust,” he said. “How do enterprises maintain trust among partners and customers in light of a tsunami of unreliable information?”
Businesses must adopt a multifaceted approach to combat AI deception, Accrete AI Chief Innovation Officer Andrés Diana told PYMNTS. He suggested that “businesses must employ rigorous testing phases that closely simulate real-world scenarios to identify and mitigate deceptive behaviors before widespread deployment.”
Additionally, implementing explainable AI frameworks is important.
“Continuous monitoring of AI outputs in production is essential, along with regularly updating testing protocols based on new findings,” Diana said. “Incorporating explainable AI frameworks can enhance transparency while adhering to ethical AI guidelines ensures accountability.”
As AI advances, the challenge of controlling its deceptive tendencies will grow more complex. Bringsjord warned of the future potential for fully autonomous AI, which is capable of setting its own goals and inventing its programs.
“Who’s to say such machines will be angelic rather than fiendish?” he said.
Experts agree that the path forward lies in robust AI governance, continuous monitoring and developing AI systems to counter deception.
“Creating open-source AI frameworks that prioritize transparency, fairness and accountability is important,” FLock.io founder and CEO Jiahao Sun told PYMNTS. “However, it’s still challenging to promote and enforce these frameworks across all AI companies.”
Sun also highlighted the importance of determining objectives for AI.
“Setting comprehensive goals for AI is difficult because AI will always look for shortcuts to maximize its objectives,” he said. “Researchers must anticipate all possible edge cases during AI training, which is tough.”
Understanding AI’s Positives and Negatives
In addition to technical solutions, improving AI literacy among consumers and businesses is crucial for fostering a more accurate understanding of AI capabilities and limitations. Boyd emphasized the role of AI literacy in promoting trust and managing expectations.
“Ultimately, the goal is to create AI systems that are not only technologically advanced but also trustworthy and beneficial for all stakeholders,” she said. “This way, we can harness the potential of AI to drive innovation and growth while safeguarding against the risks of deception and unintended consequences.”
Blackbird.AI Chief Technology Officer Naushad UzZaman told PYMNTS that AI systems are not inherently deceptive, but deception can occur when they are influenced or manipulated by malicious actors or flawed data.
Controlling deception in AI is challenging due to several factors, such as the black-box nature of AI systems, the vastness and variety of training data, and the rapid evolution of AI technology outpacing regulatory frameworks and ethical guidelines.
“These social media disinformation campaigns also become part of the web-scraped text data used to train [AI models],” UzZaman explained. “At the same time, [models] are becoming a primary user interface in search engines like Perplexity and chatbots like ChatGPT. These … products have the potential to replicate the harmful brand biases present on the web and negatively impact consumer perceptions of certain brands.”
He said AI deception can impact commerce by undermining trust between businesses and consumers.
“AI-generated fake reviews or misleading product recommendations can distort consumer choices and damage brand reputations,” he explained.
He also pointed out that deceptive AI could be used to manipulate financial markets or falsely represent products in advertising.
UzZaman recommended that businesses ensure data integrity, develop transparent and explainable AI systems, establish robust monitoring mechanisms, adopt ethical frameworks, and collaborate with industry peers and experts to fight AI deception.
“Brand bias remains an underexplored topic, and Blackbird.AI is doing some of the first work to directly quantify the brand biases of various [models],” he said. “As [these models] produce an increasing portion of the content on the web, these biases could be amplified more, making it significantly harder for brands to recover from narrative attacks in the future.”
For all PYMNTS AI coverage, subscribe to the daily AI Newsletter.