Addressing Trending Questions About Generative AI
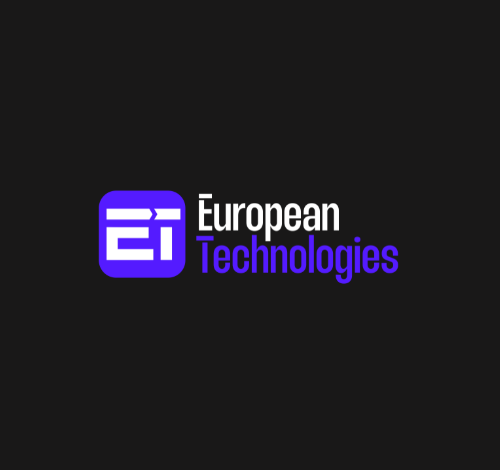
With generative AI (GenAI) top of mind across the enterprise, we are seeing leaders across the C-suite inquire about it as they explore investments in this new technology. With a focus on business value, use cases, impact on workforce and security, the effects of GenAI have far reaching implications.
Below are some of the most asked questions that enterprise leaders are asking about the technology and what the latest recommendations are to address these questions.
1. How do some organizations identify, vet and fund suitable GenAI use cases?
The most forward-thinking organizations are creating an ongoing GenAI educational curriculum to build awareness, increase knowledge and foster creation among their staff. This approach feeds a dynamic, iterative process of collecting ideas and use cases in a methodical manner.
Targeted multidisciplinary teams then utilize frameworks, such as use-case value matrix, to vet and juxtapose the ideas based on business value and feasibility. At this stage, the C-suite and technology leaders (responsible for AI, analytics, data, applications, integration or infrastructure) can work together to determine how to vet and fund the various AI initiatives. In order to do this, they should look at cost, value, and risk.
2. How are organizations aligning GenAI initiatives to business goals and assessing business value?
To assess the business value of GenAI and determine if it aligns with business goals, enterprises must create a framework that defines these categories. There are three categories to consider when creating a framework for GenAI investments:
-
Defend — Incremental, marginal gains and micro-innovations
-
Extend — Growth in either market size, reach, revenue or profitability
-
Upend — Creation of new markets and products
Those responsible for AI, as well as executive leadership, must assess the potential benefits and cost of new GenAI investments. Experimentation can be done inexpensively for most use cases.
3. What are the regulatory risks concerning LLM usage?
Depending on their locations and/or jurisdiction of operation, organizations face many potentially different constraints related to their use of large language models (LLMs). It’s critical to engage with legal specialists before the design, deployment or use of any LLM. Concerns vary widely across jurisdictions, and the effects of forthcoming legislation are yet to be understood. As for general-purpose LLMs provisioned by third parties, end users often find it impossible to control risks concerning where data is processed or sent to, the legitimacy of training data/methods, the reliability and desirability of outputs (e.g., harmful and false information), and transparency of the design, training and functions of a model.
Compliance concerns may come from intellectual property, privacy, data protection, and AI-specific technology-focused laws. There may exist a need to ensure that requirements for privacy and confidentiality extend beyond prompt content and training/pre training data, and should cover logs of user queries, enterprise context data for prompt engineering, and training data for fine-tuning.
4. How should organizations develop a GenAI governance model to manage GenAI solutions?
Additional policies and guidelines are required to use GenAI responsibly and to manage limitations and risks relating to areas such as trust, fairness, intellectual property and security.
The governance of GenAI should be complementary and aligned with existing governance of AI, data, IT and other areas. In addition, it should be compliant with emerging regulations, such as the EU’s AI Act, as well as with regional, cultural and ethical values.
To be effective, GenAI governance should be implemented through clearly defined roles and responsibilities, procedures, communication, awareness sessions and training. It should be further operationalized through practical guidelines and tool support for the development, deployment and monitoring of AI systems. Leading and coordinating AI governance is typically the responsibility of an AI center of excellence, which is owned by senior or C-level leadership and often supported by an advisory board.
5. How will the workforce be impacted?
The near-term impact of GenAI will primarily be to augment targeted activities or tasks. In most cases, job reduction or elimination will be limited for the next two to three years. The primary focus of many organizations is the profile of “productivity pursuers” using everyday AI. A limited number of organizations have embarked on “game-changing AI.” The impact that GenAI will make on the workforce will manifest itself on a case-by-case basis. It will vary by industry, geography, task, and organizational complexity. The extent of that impact depends on strategy, execution, risk management, governance, technology choices, and the ability to engender trust.
The role that is most frequently inquired about is the leader of the AI strategy and execution, namely, the head of AI. Most organizations do not need a chief AI officer. However, they do need a leader to orchestrate a holistic or integrated approach to AI and GenAI with multidisciplinary governance. The focus must be on a business strategy that is infused with AI rather than an AI technology roadmap masquerading as a strategy.
6. How do I choose between open-source models and proprietary models?
The key benefits of open-source models include customizability, better control over deployment options, the ability to leverage collaborative development, model transparency, enhanced privacy and security in part due to the transparency, and the potential to reduce vendor lock-in. Besides general-purpose open-source models, there will be lots of open-source task-specific LLMs that enterprises can choose from.
Some enterprises can leverage cloud infrastructures (infrastructure as a service or via APIs) for open-source model fine-tuning and inference. Other enterprises can choose smaller open-source models, perform lightweight fine-tuning (instruction tuning) and then host them on premises. In addition, enterprises must consider other factors.
7. Are there any security concerns regarding LLM solutions?
The major security concerns about LLMs remain data leakages and prompt injections. Attack surfaces vary depending on how LLMs are consumed. As for applications like ChatGPT, their main attack surfaces are prompts, which can be susceptible to business logic abuse and injections. LLMs’ outputs can pose a risk too because they may include malicious links and content. When integrating a third-party LLM by building its orchestration layer (e.g., prompts and RAG), you will see the attack surface expanding, especially regarding the security of API calls.
Overall, if enterprises can address these questions, they should be well on their way to making GenAI a differentiator for their business.