A hybrid artificial intelligence approach for modeling the carbonation depth of sustainable concrete containing fly ash
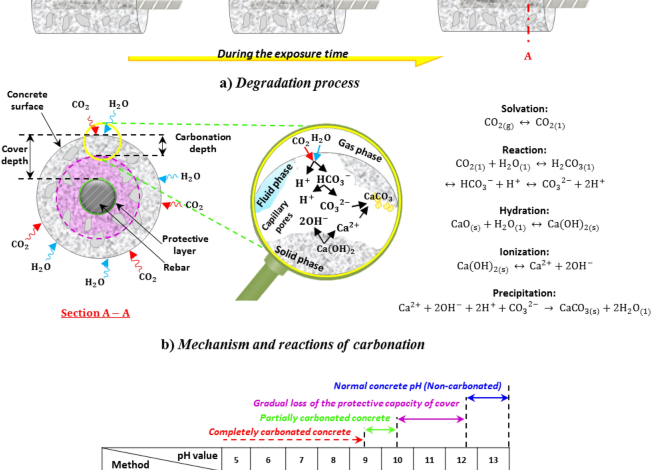
Bertolini, L., Elsener, B., Pedeferri, P., Redaelli, E. & Polder, R. B. Corrosion of Steel in Concrete: Prevention, Diagnosis, Repair (Wiley, 2013).
Czarnecki, L. & Woyciechowski, P. Modelling of concrete carbonation; Is it a process unlimited in time and restricted in space?. Bull. Pol. Acad. Sci. Tech. Sci. https://doi.org/10.1515/bpasts-2015-0006 (2015).
Hulimka, J. & Kałuża, M. Basic chemical tests of concrete during the assessment of structure suitability—Discussion on selected industrial structures. Appl. Sci. 10(1), 358 (2020).
Martys, N. S. & Ferraris, C. F. Capillary transport in mortars and concrete. Cem. Concr. Res. 27(5), 747–760 (1997).
Carević, V., Ignjatović, I. & Dragaš, J. Model for practical carbonation depth prediction for high volume fly ash concrete and recycled aggregate concrete. Constr. Build. Mater. 213, 194–208 (2019).
Castellote, M. & Andrade, C. Modelling the carbonation of cementitious matrixes by means of the unreacted-core model, UR-CORE. Cem. Concr. Res. 38(12), 1374–1384 (2008).
Cui, H., Tang, W., Liu, W., Dong, Z. & Xing, F. Experimental study on effects of CO2 concentrations on concrete carbonation and diffusion mechanisms. Constr. Build. Mater. 93, 522–527 (2015).
Leemann, A. & Moro, F. Carbonation of concrete: The role of CO2 concentration, relative humidity and CO2 buffer capacity. Mater. Struct. 50, 1–14 (2017).
Elsalamawy, M., Mohamed, A. R. & Kamal, E. M. The role of relative humidity and cement type on carbonation resistance of concrete. Alex. Eng. J. 58(4), 1257–1264 (2019).
Williams, P. J. et al. Microanalysis of alkali-activated fly ash–CH pastes. Cem. Concr. Res. 32(6), 963–972 (2002).
McCarthy, M. & Dhir, R. Development of high volume fly ash cements for use in concrete construction. Fuel 84(11), 1423–1432 (2005).
Atiş, C. D. Accelerated carbonation and testing of concrete made with fly ash. Constr. Build. Mater. 17(3), 147–152 (2003).
Khunthongkeaw, J., Tangtermsirikul, S. & Leelawat, T. A study on carbonation depth prediction for fly ash concrete. Constr. Build. Mater. 20(9), 744–753 (2006).
Thomas, M. & Matthews, J. Carbonation of fly ash concrete. Mag. Concr. Res. 44(160), 217–228 (1992).
Possan, E., Andrade, J., Dal Molin, D. & Ribeiro, J. L. D. Model to estimate concrete carbonation depth and service life prediction. In Hygrothermal Behaviour and Building Pathologies 67–97 (Springer, 2021).
Ta, V.-L., Bonnet, S., Kiesse, T. S. & Ventura, A. A new meta-model to calculate carbonation front depth within concrete structures. Constr. Build. Mater. 129, 172–181 (2016).
Liu, P., Yu, Z. & Chen, Y. Carbonation depth model and carbonated acceleration rate of concrete under different environment. Cem. Concr. Compos. 114, 103736 (2020).
Li, Q. et al. Splitting tensile strength prediction of Metakaolin concrete using machine learning techniques. Sci. Rep. 13(1), 20102 (2023).
Kazemi, R. Artificial intelligence techniques in advanced concrete technology: A comprehensive survey on 10 years research trend. Eng. Rep. 5, e12676 (2023).
Wu, F., Tang, F., Lu, R. & Cheng, M. Predicting compressive strength of RCFST columns under different loading scenarios using machine learning optimization. Sci. Rep. 13(1), 16571 (2023).
Ashrafian, A., Panahi, E., Salehi, S. & Amiri, M. J. T. On the implementation of the interpretable data-intelligence model for designing service life of structural concrete in a marine environment. Ocean Eng. 256, 111523 (2022).
Ashrafian, A., Behnood, A., Golafshani, E. M., Panahi, E. & Berenjian, J. Toward presenting an ensemble meta-model for evaluation of pozzolanic mixtures incorporating industrial by-products. Struct. Concr. https://doi.org/10.1002/suco.202300452 (2023).
Ashrafian, A., Hamzehkolaei, N. S., Dwijendra, N. K. A. & Yazdani, M. An evolutionary neuro-fuzzy-based approach to estimate the compressive strength of eco-friendly concrete containing recycled construction wastes. Buildings 12(8), 1280 (2022).
Ashrafian, A., Panahi, E., Salehi, S., Karoglou, M. & Asteris, P. G. Mapping the strength of agro-ecological lightweight concrete containing oil palm by-product using artificial intelligence techniques. In Structures (Elsevier, 2023).
Parhi, S. K., Dwibedy, S. & Panigrahi, S. K. AI-driven critical parameter optimization of sustainable self-compacting geopolymer concrete. J. Build. Eng. https://doi.org/10.1016/j.jobe.2024.108923 (2024).
Parhi, S. K., Panda, S., Dwibedy, S. & Panigrahi, S. K. Metaheuristic optimization of machine learning models for strength prediction of high-performance self-compacting alkali-activated slag concrete. Multiscale Multidiscip. Model. Exp. Design 1, 28. https://doi.org/10.1007/s41939-023-00349-4 (2024).
Rafiei, M. H., Khushefati, W. H., Demirboga, R. & Adeli, H. Neural network, machine learning, and evolutionary approaches for concrete material characterization. ACI Mater. J. 113(6), 781–789 (2016).
Basheer, I. A. & Hajmeer, M. Artificial neural networks: fundamentals, computing, design, and application. J. Microbiol. Methods 43(1), 3–31 (2000).
Kazemi, R., Shadnia, R., Eskandari-Naddaf, H. & Zhang, L. The properties of cement-mortar at different cement strength classes: Experimental study and multi-objective modeling. Arab. J. Sci. Eng. 47(10), 13381–13396 (2022).
Kazemi, R. & Naser, M. Towards sustainable use of foundry by-products: Evaluating the compressive strength of green concrete containing waste foundry sand using hybrid biogeography-based optimization with artificial neural networks. J. Build. Eng. 76, 107252 (2023).
Korouzhdeh, T., Eskandari-Naddaf, H. & Kazemi, R. Hybrid artificial neural network with biogeography-based optimization to assess the role of cement fineness on ecological footprint and mechanical properties of cement mortar expose to freezing/thawing. Constr. Build. Mater. 304, 124589 (2021).
Kazemi, R. & Gholampour, A. Evaluating the rapid chloride permeability of self-compacting concrete containing fly ash and silica fume exposed to different temperatures: An artificial intelligence framework. Constr. Build. Mater. 409, 133835 (2023).
Parhi, S. K. & Panigrahi, S. K. Alkali–silica reaction expansion prediction in concrete using hybrid metaheuristic optimized machine learning algorithms. Asian J. Civ. Eng. 25(1), 1091–1113 (2024).
Kazemi, R., Eskandari-Naddaf, H. & Korouzhdeh, T. New insight into the prediction of strength properties of cementitious mortar containing nano-and micro-silica based on porosity using hybrid artificial intelligence techniques. Struct. Concr. https://doi.org/10.1002/suco.202200101 (2023).
Felix, E. F., Carrazedo, R. & Possan, E. Carbonation model for fly ash concrete based on artificial neural network: Development and parametric analysis. Constr. Build. Mater. 266, 121050 (2021).
Kellouche, Y., Boukhatem, B., Ghrici, M. & Tagnit-Hamou, A. Exploring the major factors affecting fly-ash concrete carbonation using artificial neural network. Neural Comput. Appl. 31(2), 969–988 (2019).
Tran, V. Q., Mai, H. V. T., To, Q. T. & Nguyen, M. H. Machine learning approach in investigating carbonation depth of concrete containing Fly ash. Struct. Concr. 24(2), 2145–2169 (2023).
Huo, Z., Wang, L. & Huang, Y. Predicting carbonation depth of concrete using a hybrid ensemble model. J. Build. Eng. 76, 107320 (2023).
Haykin, S. Neural Networks and Learning Machines, 3/E (Pearson Education India, 2009).
Lippmann, R. An introduction to computing with neural nets. IEEE Assp Mag. 4(2), 4–22 (1987).
Simon, D. Biogeography-based optimization. IEEE Trans. Evol. Comput. 12(6), 702–713 (2008).
Mirjalili, S., Mirjalili, S. M. & Lewis, A. Let a biogeography-based optimizer train your multi-layer perceptron. Inf. Sci. 269, 188–209 (2014).
Kaveh, M., Khishe, M. & Mosavi, M. Design and implementation of a neighborhood search biogeography-based optimization trainer for classifying sonar dataset using multi-layer perceptron neural network. Analog Integr. Circ. Signal Process. 100, 405–428 (2019).
Ma, H., Simon, D., Siarry, P., Yang, Z. & Fei, M. Biogeography-based optimization: A 10-year review. IEEE Trans. Emerg. Top. Comput. Intell. 1(5), 391–407 (2017).
Ma, H. & Simon, D. Evolutionary Computation with Biogeography-based Optimization (Wiley, 2017).
Jiang, L., Lin, B. & Cai, Y. A model for predicting carbonation of high-volume fly ash concrete. Cem. Concr. Res. 30(5), 699–702 (2000).
Chang, C.-F. & Chen, J.-W. The experimental investigation of concrete carbonation depth. Cem. Concr. Res. 36(9), 1760–1767 (2006).
Balayssac, J., Détriché, C. H. & Grandet, J. Effects of curing upon carbonation of concrete. Constr. Build. Mater. 9(2), 91–95 (1995).
Roziere, E., Loukili, A. & Cussigh, F. A performance based approach for durability of concrete exposed to carbonation. Constr. Build. Mater. 23(1), 190–199 (2009).
Hussain, S., Bhunia, D. & Singh, S. Comparative study of accelerated carbonation of plain cement and fly-ash concrete. J. Build. Eng. 10, 26–31 (2017).
Younsi, A., Turcry, P., Aït-Mokhtar, A. & Staquet, S. Accelerated carbonation of concrete with high content of mineral additions: Effect of interactions between hydration and drying. Cem. Concr. Res. 43, 25–33 (2013).
Turcry, P., Oksri-Nelfia, L., Younsi, A. & Aït-Mokhtar, A. Analysis of an accelerated carbonation test with severe preconditioning. Cem. Concr. Res. 57, 70–78 (2014).
Chen, Y., Liu, P. & Yu, Z. Effects of environmental factors on concrete carbonation depth and compressive strength. Materials 11(11), 2167 (2018).
Golafshani, E. M., Behnood, A., Hosseinikebria, S. S. & Arashpour, M. Novel metaheuristic-based type-2 fuzzy inference system for predicting the compressive strength of recycled aggregate concrete. J. Clean. Prod. 320, 128771 (2021).
Moosavi, S. K. R. et al. A novel artificial neural network (ANN) using the mayfly algorithm for classification. In 2021 International Conference on Digital Futures and Transformative Technologies (ICoDT2) (IEEE, 2021).
Hagan, M. T. & Menhaj, M. B. Training feedforward networks with the Marquardt algorithm. IEEE Trans. Neural Netw. 5(6), 989–993 (1994).
Kazemi, R., Golafshani, E. M. & Behnood, A. Compressive strength prediction of sustainable concrete containing waste foundry sand using metaheuristic optimization-based hybrid artificial neural network. Struct. Concr. https://doi.org/10.1002/suco.202300313 (2023).
Mehlig, B. Machine Learning with Neural Networks: An Introduction for Scientists and Engineers (Cambridge University Press, 2021).
Eskandari-Naddaf, H. & Kazemi, R. ANN prediction of cement mortar compressive strength, influence of cement strength class. Constr. Build. Mater. 138, 1–11 (2017).
Golafshani, E. M., Arashpour, M. & Kashani, A. Green mix design of rubbercrete using machine learning-based ensemble model and constrained multi-objective optimization. J. Clean. Prod. 327, 129518 (2021).
Kohavi, R. A study of cross-validation and bootstrap for accuracy estimation and model selection. In IJCAI, Montreal (1995).
Taylor, K. E. Summarizing multiple aspects of model performance in a single diagram. J. Geophys. Res. Atmos. 106(D7), 7183–7192 (2001).