The AI Trust Crisis: Why Your Data Might Be Lying
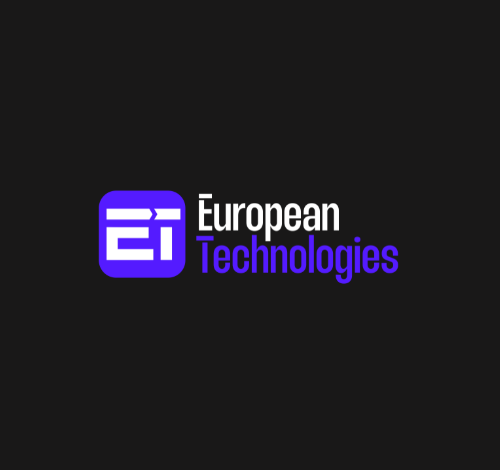
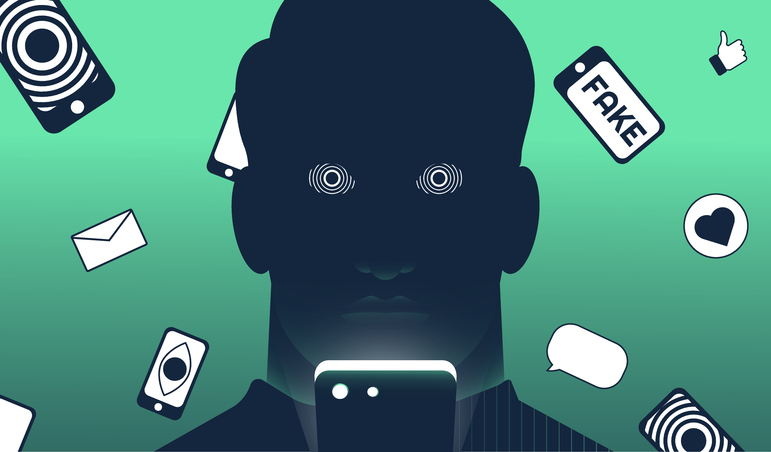
In the fast-paced world of data engineering and AI innovation, where every byte of information has the potential to transform industries and disrupt markets, the path to success is paved with data-driven insights. Yet, beneath the gleaming surface of technological advancement lies a tangled web of challenges that can ensnare even the most seasoned data engineers and leaders.
A recent survey by Alteryx, a leading data analytics platform, has illuminated a glaring discrepancy between organizations’ trust in their data and the actual quality of that data. Philip Madgwick, regional vice president for Asia at Alteryx, observes, “In order to really get the best use out of artificial intelligence… we have to trust the data that is contributing to any AI sort of insights.”
Trust, it seems, is the cornerstone of data-driven decision-making. However, as Madgwick points out, “Different countries are at different maturity levels” regarding AI and data readiness. While some organizations are racing towards AI adoption with robust data pipelines and ETL processes, others are taking a more cautious approach, recognizing the need to establish a solid data quality and governance foundation before embarking on their AI journey.
The trust gap in the data lake
One intriguing finding of the Alteryx survey is the high level of trust many organizations express in their corporate data. This raises the question: Is this trust a reflection of genuine confidence in data quality, or is it simply a lack of understanding of what constitutes “good” data?
Madgwick suggests that organizations often pull data from various sources owned by different teams or individuals. This fragmented approach to data management can create a disconnect between the source of the data and the people responsible for extracting and manipulating it. As a result, those not directly involved in data ownership may be more skeptical about its quality and reliability, leading to data swamps rather than well-structured data lakes.
The leadership disconnect: Data literacy is key
While many organizations aspire to leverage AI and data analytics, there seems to be a disconnect between leadership aspirations and the actual capabilities of their workforce.
Madgwick emphasizes the importance of upskilling employees in data and analytics, stating, “It’s all well and good saying we want to leverage AI…but if you don’t have the skill sets internally in order to do that, that’s where perhaps you can use the phrase gap or not, but that’s where most organizations are sort of trying to tackle today.”
This sentiment is echoed in the experiences of companies like Coca-Cola, which partnered with Alteryx to address challenges related to merging and preparing data from disparate sources. By focusing on both upskilling employees in data wrangling techniques and leveraging advanced data analytics platforms, Coca-Cola was able to unlock valuable insights and optimize various aspects of its business.
Breaking down data silos with unified platforms
One of the most pervasive roadblocks to data-driven success is the siloed mentality in many organizations.
Departments often hoard their data, reluctant to share it with others for fear of losing control or revealing weaknesses. This creates a fragmented data landscape that hinders collaboration and stifles innovation.
To overcome this challenge, Madgwick suggests adopting a unified platform approach to data management with clear policies and governance frameworks. This enables organizations to create a data-driven culture where data is accessible to the right people while maintaining appropriate controls and ensuring data lineage.
The legacy data dilemma: Data modernization to the rescue
Many organizations are grappling with legacy data systems that are not designed for the speed and agility required in the AI era. These systems can impede progress, making it difficult to extract, transform, and load (ETL) data efficiently.
Madgwick acknowledges this challenge but points out that modern data platforms make accessing and extracting data from legacy systems easier. He advises organizations to focus on developing a clear data strategy and investing in the right ETL tools and technologies to unlock the value of their data.
Critical lessons for data leaders
As organizations navigate the complex data landscape, several vital lessons emerge that Madgwick highlights:
- People First: Invest in upskilling your workforce in data engineering and analytics best practices. Empower them with the knowledge and tools to extract insights from your data.
- Break Down Silos: Foster a data-driven culture where data is shared, and collaboration is encouraged through unified platforms and tools.
- Centralize Data Management: Adopt a centralized approach to data management, with clear policies and governance frameworks in place, including robust data lineage tracking.
- Invest in Modern Data Platforms: Leverage modern data platforms with ETL capabilities to overcome the limitations of legacy systems.
- Develop a Clear Data Strategy: Align your data strategy with your business goals and invest in the right tools and technologies to unlock the value of your data.
By heeding these lessons, data leaders and engineers can navigate the roadblocks of data for AI and pave the way for a future where data-driven insights drive innovation and growth. The path may be winding, but the destination is within reach with the right approach, Madgwick concludes.
Image credit: iStockphoto/Moor Studio