Data Science Demystified: Leveraging Big Data for Insights and Innovation | by Silent Scientist’s Sphere | May, 2024
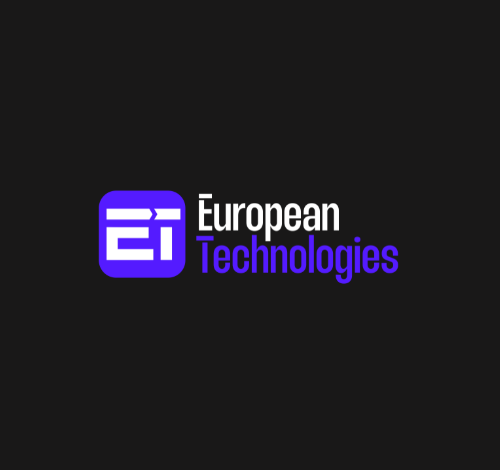
In today’s digital age, the term “data science” frequently appears in discussions about business strategy, technological advancement, and innovation. Despite its buzzword status, data science remains an enigma to many. This article aims to demystify data science by exploring its fundamentals, the role of big data, and how organizations can harness its power for insights and innovation.
Understanding Data Science
At its core, data science is an interdisciplinary field that utilizes scientific methods, algorithms, and systems to extract knowledge and insights from structured and unstructured data. It encompasses a range of techniques from statistics, machine learning, and data mining to big data analytics.
Key Components of Data Science
- Data Collection: Gathering raw data from various sources such as databases, social media, sensors, and more.
- Data Processing: Cleaning and organizing data to make it suitable for analysis.
- Data Analysis: Applying statistical methods and machine learning algorithms to analyze data.
- Data Visualization: Presenting data in graphical or pictorial formats to make findings accessible and understandable.
- Interpretation: Translating analytical results into actionable insights for decision-making.
The Role of Big Data
Big data refers to the vast volumes of data generated at high velocity and in diverse formats. These characteristics are often described by the three V’s: Volume, Velocity, and Variety.
Why Big Data Matters
The explosion of data from digital devices, social media, and IoT (Internet of Things) has created unprecedented opportunities for businesses. Big data analytics allows organizations to:
- Enhance Customer Experience: By analyzing customer behavior, preferences, and feedback, companies can tailor their services and products to meet customer needs more effectively.
- Improve Operational Efficiency: Identifying patterns and trends in operational data helps businesses streamline processes, reduce costs, and increase productivity.
- Drive Innovation: Insights derived from big data can lead to the development of new products, services, and business models.
- Risk Management: Predictive analytics can forecast potential risks and enable proactive measures to mitigate them.
Leveraging Data Science for Innovation
To fully leverage data science, organizations need to foster a data-driven culture and invest in the right tools and talent. Here are some strategies to achieve this:
1. Building a Data-Driven Culture
Encouraging a data-driven mindset requires leadership commitment and a shift in organizational culture. Employees at all levels should be trained to understand the value of data and how to use it in their daily tasks.
2. Investing in Technology
Advanced analytics platforms, cloud computing, and AI-powered tools are essential for handling big data. Investing in these technologies enables businesses to perform complex analyses and gain deeper insights.
3. Hiring Skilled Professionals
Data scientists, analysts, and engineers play a crucial role in unlocking the potential of big data. Organizations should focus on hiring and retaining talent with expertise in data science and analytics.
4. Fostering Collaboration
Collaboration between data scientists and domain experts ensures that analytical models are relevant and aligned with business objectives. Cross-functional teams can drive more innovative solutions by combining technical and industry knowledge.
5. Ensuring Data Governance
Effective data governance frameworks ensure data quality, security, and compliance. Clear policies and procedures for data management help organizations maintain trust and integrity in their data practices.
Real-World Applications
Many organizations have successfully leveraged data science to transform their operations and gain a competitive edge. For instance:
- Healthcare: Predictive analytics in healthcare can forecast disease outbreaks, improve patient care, and optimize hospital operations.
- Retail: Retailers use data science to optimize inventory management, personalize marketing campaigns, and enhance customer experience.
- Finance: Financial institutions employ machine learning algorithms to detect fraud, assess credit risk, and automate trading.
Conclusion
Data science, with its ability to transform raw data into valuable insights, is a powerful tool for innovation and growth. By understanding its principles and investing in the necessary resources, organizations can demystify data science and harness the power of big data to drive success in today’s competitive landscape.