Locating carbon neutral mobility hubs using artificial intelligence techniques
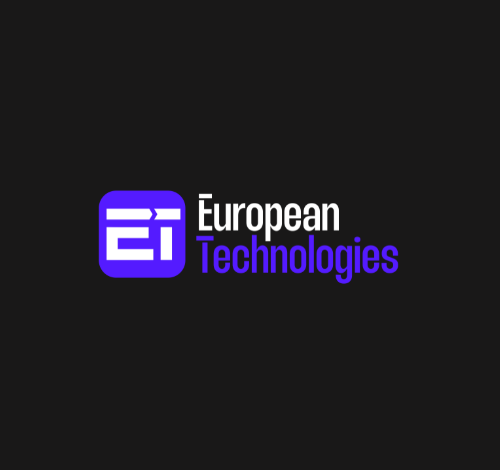
This section reveals the effectiveness of the chosen approach in enhancing the sustainability and efficiency of mobility hubs by examining their potential impact. This section then explores the study’s major contributions and policy implications.
First stage of hubs’ locations selection
This study optimized the selection of mobility hub locations using a genetic algorithm (GA). The GA process begins by initializing a population and selecting individuals based on their fitness. It then applies crossover mutations to iteratively evolve solutions, thereby emphasizing the survival of the fittest. This method systematically improves the selection of locations by adjusting key parameters such as the number of generations and mutation rates, thereby enhancing the quality of solutions17. Through the parallel exploration of solutions, the GA significantly increases the likelihood of identifying optimal hub sites owing to its efficient site optimization based on evolving fitness values.
The application of the GA in this study focused on optimizing traffic flow by minimizing travel times, successfully achieving a significant fitness value of 7.7e7. The GA-selected sites consistently favored areas with high traffic, as illustrated in Fig. 5. For instance, the selected site in Seoul’s central region is located in a densely populated district that is known for its commercial and tourist prominence. In contrast, the site in the east-north region features several transit junctions and routes. Meanwhile, selected sites in the west–north, west–south, and east–south zones are districts with high population density, concentrated academic institutions, and a well-connected commuting network within the Gyeonggi province.
Second stage of hub location selection
This study transcends the constraints of traditional MCDM tools for location selection by integrating Ensemble methods. These methods derive importance scores for various factors with carbon emissions as the dependent variable, thereby serving as weights for these factors. This approach not only enhances accuracy but also promotes sustainability and efficiency in the decision-making process.
Initial analysis
The carbon emissions factor demonstrated a lognormal distribution, with most values being centralized around the mean (Fig. 2). These values predominantly ranged from 10 to 30 carbon units (CO2ppm). According to Fig. 3, the analysis indicates that the carbon emissions factor has a positive correlation of 0.49 with parking accessibility. Conversely, it shows a negative correlation of − 0.43 with public transit and cycling accessibility.
Beyond traditional methods such as London’s congestion pricing18, Bogotá’s TransMilenio bus lanes19, Copenhagen’s bike lane expansion20, and Prague’s dynamic parking pricing21, truly effective green transportation initiatives require a shift in focus. Central to this approach are the concepts of the 15-min city22 and TOD-oriented neighborhoods23, alongside the development of efficient and eco-friendly mobility hubs24. These strategies collectively underpin sustainable transportation systems that aim to mitigate environmental challenges while promoting healthier and more resilient communities. For these mobility hubs to effectively fulfill their roles, it is crucial to adopt a holistic strategy that not only enhances existing mobility infrastructure and services but also incorporates flexibility to respond to changing urban needs. This foundation supports the creation of hubs that are not only environmentally sustainable, but also dynamically responsive to the evolving landscape of urban mobility.
Ensemble-based weights calculation of factors
The study employed Ensemble methods to address the complexities of high-dimensional and multicollinear data, focusing on identifying key variables for accurate predictions. The data were split into 80% for training and 20% for testing, evaluating various algorithms, including the Decision Tree Regressor, Random Forest, AdaBoost. Notably, the Random Forest, Gradient Boosting, Bagging, and XGBoost Regressors stood out, with XGBoost showing exceptional performance, achieving an R-squared of 0.99 for training and 0.53 for testing, along with low error rates. Conversely, the Decision Tree and KNeighborsRegressor experienced overfitting and the MLP Regressor underperformed. The research then leveraged the top-performing models for GIS suitability analysis, demonstrating how Ensemble methods can enhance urban planning decision-making through comprehensive data analysis.
Although a 53% R-squared score might initially seem modest, it holds considerable significance in the context of urban transportation planning, where factors such as human behavior and urban development introduce substantial complexity. This score indicates that the models successfully accounted for over half of the variability in the data, providing a robust basis for making well-informed decisions in urban transportation planning.
As illustrated in Fig. 4, the importance scores of the factors extracted from the best-performing Ensemble algorithms offer valuable insights into their contributions to carbon emissions. Cycling has the highest importance score of 0.3, indicating that promoting cycling can significantly reduce carbon emissions. Parking followed closely with a score of 0.232, emphasizing the importance of regulating parking spaces to reduce car dependency. Public transit has an importance score of 0.228, highlighting the role of well-connected and efficient public transit in reducing car usage, and consequently, carbon emissions. The land use mix and densities of population and employment have comparatively lower importance scores of 0.045, 0.069, and 0.087, respectively. Employment density plays a slightly stronger role, suggesting that job hubs influence mobility choices and, thereby, transportation emissions.
Suitability analysis
In the selection phase, the previously calculated weight values are crucial for conducting suitability analysis and identifying the most sustainable hub locations. According to Fig. 5, the five districts with the highest suitability scores are (1) Yeoksam 1-dong, (2) Myeongdong, (3) Yeouido, (4) Jongno 1, 2, 3, and 4-ga-dong, and (5) Hoehyeon-dong. However, given that three of the top five selected sites were within the central region, this study further refined the selection by comparing suitability scores across different regions. This regional comparison led to the final selection of hub sites (Fig. 5), which included Yeoksam 1-dong in the east-south, Myeongdong in the center, Yeouido in the west-south, Seogyodong in the west-north, and Yongsindong in the east-north.
Yeoksam 1-dong, distinguished by its high concentration of job hubs and a comprehensive public transit network, presents a compelling case for the establishment of a mobility hub. Myeongdong, serving as both a commercial nucleus and cultural center for locals and tourists, necessitates advanced mobility solutions to accommodate its bustling activity and economic significance. Yeouido, the financial hub of South Korea, not only hosts paramount financial institutions but also features many leisure attractions and a well-connected transportation network. In Seogyo-dong, a district celebrated for its IT industry leadership, the introduction of mobility hubs can further foster innovation. Meanwhile, Yongsin-dong, a densely populated area with several educational establishments and a mix of residential and commercial spaces, exhibits diverse and high travel demands. The strategic integration of mobility hubs into these districts promises to enhance accessibility and support the distinct economic, cultural, and educational fabric of each area, advocating a nuanced approach to urban and mobility planning.
Quantitative post-assessment
Sensitivity analysis was conducted to determine whether implementing mobility hubs in selected locations could effectively decrease congestion and carbon emissions. It was assumed that implementing a mobility hub would reduce travel time by 10%, followed by traffic assignment analysis using Emme software. The results indicated a significant shift in traffic flow: the use of private cars decreased on almost every road, favoring an increase in public transit modes, including bus, subway, or combined bus-subway trips (Table 1). Specifically, private vehicles and taxi trips showed a daily decrease of 37.75% and 14.22%, respectively, whereas the total number of buses, subways, and their combined trips increased by approximately 50% (Table 1).
These findings, displayed in Table 1, demonstrate that mobility hubs have effectively reduced reliance on personal cars and taxis, while promoting the usage of public transit modes. The combined subway and bus modes also saw an increase, indicating the hub’s effectiveness in facilitating multimodal journeys. Therefore, mobility hubs have successfully influenced modal choices, steering users towards more sustainable and efficient modes of travel.
In this study, a traffic assignment model was applied to measure the changes in VKT and VHT before and after the introduction of mobility hubs. These metrics are crucial in calculating key economic advantages, including operational cost savings, travel time savings, safety-improving benefits, and reduction in air pollution. The results showed a reduction of 771,074 VKT per day, demonstrating that these hubs have effectively influenced modal choices by making public transport or other sustainable modes more appealing, as confirmed by the modal shift (Table 1). Similarly, there was a decrease of 37,056 VHT per day, suggesting an improvement in trip efficiency due to reduced congestion and more direct routes facilitated by the implemented hubs.
Table 2 presents the economic benefits accrued from the introduction of mobility hubs following the instructions of the Korean feasibility study guidelines. The results indicated annual operational cost savings of approximately 65.2 million USD, largely due to more streamlined operations, decreased maintenance, and efficient use of transportation resources as hubs integrate and connect various modes. Annual travel time savings were around 153.75 million USD, reflecting the hubs’ roles in minimizing delays and facilitating faster commutes. Additionally, hubs have fostered safer transportation, with annual savings of approximately 3.1 million USD, mostly due to improved road use, modal transfer, and reduced congestion. On the environmental front, the hubs contributed to a reduction in air pollution, with annual savings of over 3.8 million USD, mainly attributed to decreased private vehicle usage and shifts to public transit. Consequently, the establishment of these mobility hubs generated significant annual economic gains, totaling 225.9 million USD.
This study delved into the outcomes of implementing mobility hubs with a focus on the impact of the methodologies used for site determination. Employing GAs, the research optimized travel times, favoring high-traffic areas in Seoul. Recognizing the need to consider additional environmental aspects, the second stage utilized Ensemble methods to (1) incorporate several key factors and (2) account for carbon contributions to the decision-making process. This provided a comprehensive approach for site selection. These findings identified factors such as cycling and parking proximity as significant contributors to carbon emissions. Following the application of GA, the Ensemble-based suitability analysis further refined site selection. A quantitative post-assessment confirmed the positive impacts of mobility hubs, showing significant reductions in the modal share of private vehicles as well as in the VKT and VHT metrics. The research estimated the calculated economic benefits to be approximately 225.9 million USD annually. This study intends to bridge theoretical methodologies with practical urban benefits to guide future sustainable urban and transportation planning initiatives.
Policy recommendations
Beyond traditional considerations of travel time in selecting mobility hub locations, this study employs an Ensemble-based suitability analysis that incorporates each factor’s contribution to carbon emissions. It integrates cycling, parking, and public transit proximity to determine optimal sites for mobility hubs. Additionally, a post-assessment quantitatively evaluates the environmental and economic benefits using traffic assignment modeling. Given the comprehensive findings, this study advocates for the following policies:
-
1.
Considering various factors:
-
Incorporating a range of factors beyond travel time for a more realistic approach, encompassing socioeconomic, land use, transportation infrastructure, and environmental criteria.
-
Tailoring these considerations to the unique characteristics of each city, incorporating the key elements influencing travel patterns.
-
-
2.
Leveraging AI and new technologies in decision making:
-
Utilising AI techniques to optimize site selection and predict possible outcomes.
-
Implementing IoT and big data abalytics to increase the accuracy of the optimization, and to continusouly monitor and refine mobility hubs functioning.
-
Employing AI tools to visualize different scenarios and facilitate stakeholders discussion and participation.
-
-
3.
Implementing ensemble-based suitability analysis:
-
Utilizing ensemble methods to ensure non-biased decision-making.
-
Applying these methods to achieve various goals, such as reducing carbon emissions, relieving congestion, and enhancing physical activity.
-
Customizing the reflection of carbon emissions in factors’ weights for the identification of mobility hub sites.
-
Implementing these carbon-based weights permits to quantify reductions in carbon emissions, supporting cities in achieving environmental goals like those outlined in the Paris agreement.
-
-
4.
Selecting hubs sites: must-included characteristics:
-
Considering cycling proximity in the selection of hub locations, as it significantly reduces carbon emissions.
-
Establishing a cycling network connecting major residential and commercial areas to hubs, ensuring safe and direct routes for cyclists, integrated with features like bike parking, repair facilities, and bike-only lanes.
-
Recognizing the significance of parking proximity in reducing carbon emissions, by integrating parking proximity in the site selection process and including parking spaces in mobility hubs to discourage car use.
-
Implementing parking policies such as dynamic parking pricing and limited parking authorization to deter car usage and generate revenues.
-
Integrating active mobility (cycling and pedestrian facilities) and public transit modes within mobility hubs to strengthen their attractiveness and usage convenience.
-
Promoting policies such as improving modal integration and providing financial incentives to choose active and public transit modes.
-
-
5.
Continuous monitoring and assessment:
-
Gathering qualitative insights through user satisfaction surveys to evaluate travel experience at mobility hubs.
-
Tracking quantitative measures such as changes in public transit ridership, reduction in private vehicle usage, air quality improvements, and public health metrics.
-
Systematically incorporating user feedback and quantitative real-time data into mobility planning.
-
Employing a dynamic approach to evaluation and adaptation, allowing mobility hubs to evolve in response to changing needs and conditions, and ensuring their long-term effectiveness.
-
By adopting these policy recommendations, cities can progress towards developing efficient, sustainable, and accessible mobility hubs, thereby significantly enhancing their sustainability and efficiency.