Reality check: Real generative AI game changers
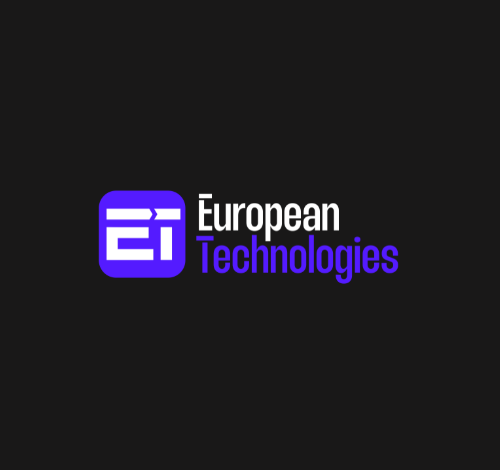
May 30, 2024
JAKARTA – Generative artificial intelligence exploded into the public consciousness in 2022, and in 2023 AI began to take root in the business world. Now is the pivotal year for the future of AI, as researchers and enterprises seek to establish how this evolutionary leap in technology can bring the most impact to businesses that make up our everyday lives.
Generative AI has already reached its “hobbyist” phase, with last year seeing an increasing number of foundation models with open licenses. Enhanced with fine-tuning techniques and datasets developed by the open-source community, many open models can now outperform all but the most powerful closed-source models on most benchmarks, despite far smaller parameter counts.
Although the ever-expanding capabilities of state-of-the-art models will garner the most media attention, the most impactful developments may be those focused on finding the right business use cases, the ability to customize based on real business needs and the right governance that make generative AI more trustworthy, sustainable and accessible, for enterprises and end users alike.
Here are some important AI game changers to look out for as we approach mid-2024.
First, small(er) language models advancements.
In domain-specific models – particularly large language models (LLMs) – we’ve likely reached the point of diminishing returns from larger parameter counts. Massive models jumpstarted this ongoing AI golden age, but they are not without their drawbacks. Only the very largest companies have the funds and server space to train and maintain energy-hungry models with hundreds of billions of parameters.
According to one estimate from the University of Washington in the United States, training a single GPT-3-sized model requires the yearly electricity consumption of over 1,000 households; a standard day of ChatGPT queries rivals the daily energy consumption of 33,000 US households. Smaller models, meanwhile, are far less resource-intensive.
These advances in smaller models have three important benefits: they help democratize AI, running at a lower cost on more attainable hardware; they can be run locally on smaller devices, allowing more sophisticated AI in scenarios like edge computing and the Internet of Things (IoT); and they make AI more explainable – the larger the model, the more difficult it is to pinpoint how and where it makes important decisions. Explainable AI is essential to understanding, improving and trusting the output of AI systems.
Second, Bring Your Own Model (BYOM).
Key to the vision of AI for business must be the notion of empowerment. Every organization must be able to deploy open AI models to meet its own goals, and every enterprise should have its own regulations to conform to, whether they come from laws, social norms, industry standards, market demands or architectural requirements.
No one model can rule the world, organizations want the right models for the right use cases. They must also be able to use their own model based on their needs. As such, custom foundation models are becoming more popular as enterprises seek to avoid sending their data to third parties and to build models that are tailored to their specific needs. These customized language models are able to better recognize nuances in language that generic models may miss.
For example, a customer service chatbot may be more effective when it is customized with data from the company’s customer service department, as this data can help the model better identify customer needs and respond appropriately. Similarly, a model used to detect fraud can be more effective when it is customized with data from the company’s fraud detection system.
Third, responsible AI.
Without responsible AI and AI governance, organizations will not be able to adopt AI at scale. There is no doubt that businesses will gain a competitive advantage by adopting AI, but organizations must also weigh the rewards against the investment and the risks it could introduce, including privacy, accuracy, explainability and bias. Generative AI is ushering in a new era, but it must be trusted above all else.
Trust is our license to do business and customers are judging every decision we make. Organizations cannot make AI break that trust. For one, several years ago, IBM published our high-level Principles for Trust and Transparency.
We believe that the purpose of AI is to augment – not replace – human expertise, judgment and decision-making. Data and insights generated from data belong to their creator, not their IT partner. And finally, powerful new technologies like AI must be transparent, explainable and free of harmful and inappropriate bias in order for society to trust them.
Organizations cannot pass the buck on AI ethics. CEOs must champion policies and processes that provide transparency and accountability across the board, offering clarity on how and where technology is being used, as well as the sources of the data sets or underlying foundation models. Business leaders must foster a culture focused on AI ethics, which aims to optimize AI’s beneficial impact while reducing risks and adverse outcomes for all stakeholders. Responsible AI cannot be an afterthought.
In the end, despite the hype, AI is a long game, not a quick match. Businesses must carefully consider how AI can help their business in the long term, instead of simply installing it for immediate gains.
By carefully considering the right models to bring real value to their business, with the right governance from the start to the end of their AI journey, businesses can be more agile in scaling up their business while at the same time ensuring they retain the trust of all their stakeholders.