Navigating the Future of Generative AI: Fine-Tuning vs. Retrieval-Augmented Generation
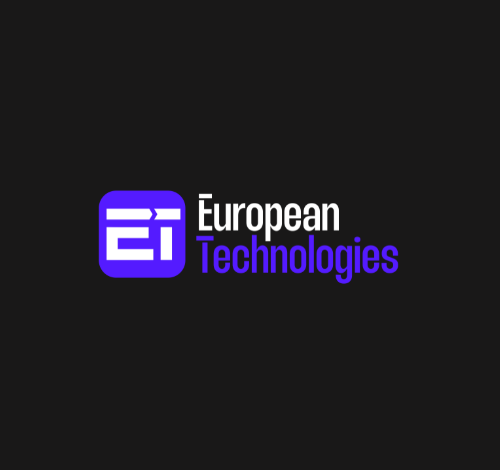
Generative AI’s influence in India shows no signs of slowing down. A report by NASSCOM predicts the technology’s market is expected to reach a high of USD 22 billion by 2027. The same report also found that investments in AI have surpassed the USD4 billion mark between 2022 and 2023.
With hopes riding on AI being good for businesses and the nation at large, there has never been a stronger case for ensuring the accuracy of generative AI. As is common knowledge now, the large language models that power AI are far from perfect. Many times, they provide comically inaccurate answers. Other times, less humorously, these answers can be dangerous. Because of their “stateless nature”, LLMs cannot store data, meaning they can only respond based on the data they were trained with. Any knowledge gaps are filled by crafting information that sounds believable.
There are two ways to resolve this: fine-tuning or retrieval augmented generation (RAG).
Fine-tuning to introduce meaning
Fine-tuning an AI model involves introducing smaller and more specific data sets to help it complete specific tasks or fix problems. Say that you are using an AI application to find recommended restaurants. While the LLM might be trained to provide restaurant, options located within the same area as your current location, it doesn’t know where you have eaten over the past week. By fine-tuning the model with data on recent meals you have eaten, it can provide users with a more personalized list of choices.
The problem, however, comes when you have to regularly introduce data to ensure relevant insights. Not only is this a cumbersome process, but it can create frustrated customers. For instance, fine-tuning an e-commerce app model may lead customers to recommend products that were sold out only a few minutes ago, making for a frustrating experience.
Moreover, inserting proprietary data like personally identifiable information (PII) and company secrets can increase the risk of privacy compromise. Because there is no guarantee that data will be kept secure, customers may be more likely to face assaults from cyberattacks and organisations will be forced to face increased sanctions from regulatory bodies.
That’s not to say that fine-tuning AI models is not feasible. In cases where embedding models do not understand certain words or names, data teams can provide the correct meanings to improve the accuracy of generative AI responses.
Speedy and secure insights require RAG
RAG techniques distinguish themselves from fine-tuning methods by not changing or introducing new data into the LLM. Instead, they extract data from other sources to help models produce relevant and accurate answers. Because data and prompts are stored as vectors, models can find the right answers easily and quickly.
Unlike fine-tuning methods which require the manual addition of information, RAG systems can automatically update data and make them available for querying. This, in turn, eliminates the need for organizations to group data in batches before processing them all at once.
RAG systems also provide data teams with increased security through fine-grained access controls. In particular, data teams that manage chatbots can build code that enables customers to view only their personal information.
Make the right call
Fine-tuning methods work well in situations where organizations need to introduce words to certain meanings or information that is not likely to change, or which requires constant updates. It is also ideal for certain tasks that demand accuracy to such a degree that it warrants organizations throwing a large number of resources at it.
However, in situations where real-time information needs to be protected at all times, organizations should turn to RAG. By providing relevant data at the exact moment, it is required while limiting access, models will be able to empower apps to deliver highly effective personalisation.
Whether it is providing incisive medical treatment recommendations or planning out a travel itinerary tailored to an individual’s preferences, RAG is a game-changer for content generation. Ultimately, the fact that it demands multi-step reasoning or synthesis of information from various sources is what makes it ideal for apps that require depth, context, factual accuracy, and security at speed and scale.
Authored by Mukundha Madhavan, APAC Tech Lead, DataStax