Corporations looking at AI as a productivity tool are making a mistake
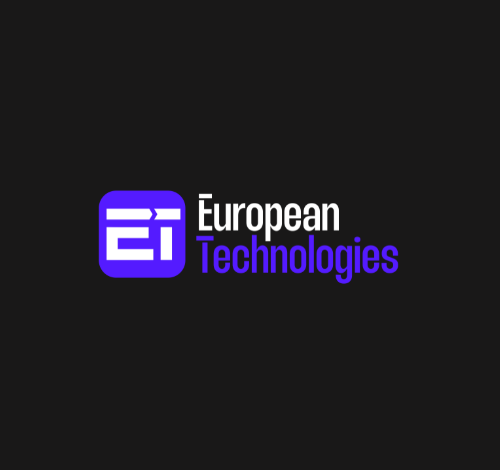
- Only 5% of companies have achieved mature generative AI initiatives, with 45% delaying investment, according to a new research report by Genpact and HFS Research.
- The research shows that about half of the executives have a ‘myopic’ view of the tech, framing gen AI solely as a productivity tool.
- To move AI from a pilot stage to production, companies need to make sure gen AI plans are aligned with specific business objectives.
Andriy Onufriyenko | Moment | Getty Images
Despite all the hype surrounding generative artificial intelligence, many organizations have yet to make significant investments in the technology. And that might not be a bad thing, as it gives the C-suite an opportunity to prepare their companies for widespread use of AI tools and services before taking the financial plunge.
Only 5% of senior leaders at global organizations say their companies have achieved mature gen AI initiatives, according to a new research report by professional services firm Genpact and research and analysis firm HFS Research. Some 45% are delaying investment, taking a wait-and-watch approach.
Part of the slow spending approach is coming from the fact that senior executives are looking at gen AI the wrong way, said Sreekanth Menon, global leader, AI and machine learning at Genpact. The research showed that about half of the executives are framing gen AI solely as a productivity tool.
“This is a case of myopic vision,” Menon said. “The infancy of gen AI, combined with a warped conception of its potential, has led to reluctant budgetary spending.”
With AI, organizations frequently focus on short-term, low-hanging fruit, “which results in substantial technology and process debt that becomes costly to manage as the organization grows,” said Paul Pallath, vice president of the applied AI practice at technology consulting firm Searce.
“This is why only a few organizations have mastered leveraging AI at scale and disrupting the marketplace effectively,” Pallath said. “The true potential of AI will never be realized if organizations pursue short-term goals or focus solely on quick wins without a long-term strategic plan.”
The research from Genpact and HFS showed that business leaders are dedicating up to 10% of their IT budgets to gen AI projects. But factors such as data governance concerns, talent shortfall, and proprietary data accessibility have contributed to low spending and have widened the barrier between pilot and production, Menon said.
So, what do companies need to be doing before deploying AI across business functions?
One thing is to get their priorities straight, Menon said. “To successfully move AI from a pilot stage to production, organizations need to pause and ensure their gen AI plan is aligned with specific business objectives, not just productivity,” he said. “Many organizations are limiting the rollout of successful, long-term AI strategies because they’re too focused on what it can do to boost productivity, rather than its broader benefits.”
Taking the time to focus on the bigger picture will set up organizations for more success in the future, Menon said.
AI is transformational and requires a comprehensive reevaluation of current business processes, data strategies, technology platforms, and people strategies, Pallath said.
“Implementing AI effectively necessitates simplifying and revamping business processes with an AI-first mindset,” Pallath said. “Effective change management and governance are crucial to ensure that the entire organization is prepared for and engaged in this transformation.” What often happens, he said, is that employees worry more about AI’s impact on their jobs, rather than how they can leverage the technology to help them work smarter, thereby hindering the necessary changes in process to make AI successful.
Executive leadership and sponsorship are also critical. “AI initiatives need strong leadership support to overcome inertia and gain the necessary resources,” Pallath said. “Without a clear vision from the top, AI projects are more likely to get stalled or diluted.”
A dedicated AI team headed by a chief AI officer can help ensure success. “A dedicated leader, ideally at the executive level, ensures AI remains a top priority and champions its integration into the company,” Pallath said. “AI requires significant investment in technology, talent, and infrastructure. Strong leadership prioritizes these resources and ensures their effective allocation to maximize return on investment.”
The AI team might include data scientists; machine learning engineers; an AI specialist with domain expertise; and software engineers to design, build, and deploy predictive models and algorithms. And even as they build specialized teams, companies need to prepare their existing workforce for a future where AI is ubiquitous, Pallath said. That includes letting everyone in the company know the importance of data, how to use it ethically, and how it’s being used within the business.
Another good practice is to establish a company culture of responsible AI. “Companies need to start their AI journey with a clear view of responsible and ethical AI considerations, and ensure they are understood across the organization,” Menon said.
To do this, they should create a responsible AI framework that provides a map to achieving responsible AI, which includes key components such as privacy/security, reliability/safety, and explainability/traceability — to name a few.
Beyond a framework, to create a culture where responsible AI is at the center, companies need to build awareness of responsible AI, identify which processes gen AI will impact and take action to mitigate legal, security, or ethical concerns, Menon said.
Finally, data quality is imperative for successful gen AI. As noted in the research report, companies looking to achieve business gains from gen AI within two years are grappling with challenges such as data quality and strategy, underscoring the urgent need for a robust data strategy.
“While AI is a disruptive technology, its effectiveness is heavily dependent on the quality of data,” Pallath said. “Many companies struggle with developing a comprehensive data strategy that includes proper governance and quality processes. Data often becomes an afterthought, and investments in data management are frequently viewed as costs rather than revenue enablers. This mindset leads to data swamps buried in silos, hindering the development of high-quality AI solutions.”