How AI and Machine Learning Can Transform Pharmacy Benefits Management
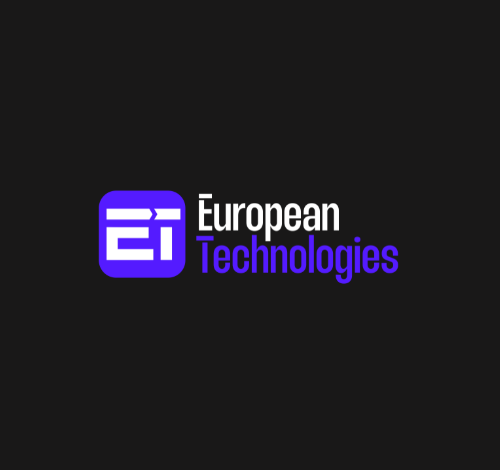
For years, leaders in the health and tech industries have hypothesized how AI can transform the healthcare experience. While there has been some progress in clinical applications – for example, diagnostic tools – Abarca sees a critical use case that can deliver a better experience for everyone: generating greater value from healthcare data.
Given their relationship with payers, providers, and consumers, PBMs are uniquely capable of leveraging AI to take friction out of pharmacy benefits. But integrating AI and machine learning is not straightforward. Spencer Ash, associate director of user experience at Abarca, and Simon Nyako, the company’s senior manager of actuarial services, spoke recently with MedCity News about the opportunities and challenges facing the adoption of AI and machine learning in healthcare, as well as the importance of maintaining a human touch.
Note: This interview has been edited for clarity.
Given how most healthcare companies manage and organize their data, how hard will it be for them to implement artificial intelligence and expand its use?
Spencer Ash: Data is often collected in different systems and/or stored on different servers, making it hard for all that information to interact together in one place. That’s a big challenge, especially for generative AI, which needs a lot of direction on where to get data.
Another challenge is regulatory requirements, such as HIPAA, that cover protected health information and other sensitive personal data. This makes it even more important to know and consider the data’s source and ensure that it is being managed
appropriately.
There are a number of other potential hurdles, including interoperability – which has been an ongoing challenge across the industry – and data integrity and accuracy. And the ethics of some of these potential data applications must also be considered.
Depending on the organization, these factors could make it very difficult to implement AI. But, that type of work also brings benefits beyond just this technology, and I believe it’s worth the effort.
In your experience as a PBM, what do you see as the best near-term applications for the technologies?
Simon Nyako: Machine learning is going to assist in any place where a prediction is involved as it can help to better define what will happen to one thing in reaction to another – which comes up a lot at PBMs. For example, formulary optimization, network
optimization and trend analysis, where making one change will impact many related components.
On the generative AI side, I’m really excited about the potential to help people become more conversational with their data, to type in a question in natural language and get a response back. This can empower people to be more curious in their analysis and enable them to retrieve and explore extra pieces of information without needing to submit another request to their data team.
Ash: As far as a specific example, there is the potential for the automation of prior authorizations. When done manually, it can be time-consuming to obtain approval from the payer even after a medication has been prescribed. As a result, the patient doesn’t
get their medications right away, which could have significant implications for health outcomes. But algorithms can be used to analyze patient data, clinical guidelines and payer policies to streamline the process, reduce the administrative burden and
accelerate access.
Similarly, this technology can be used to address another persistent problem in healthcare, medication adherence. Data, like refill behavior and previous responses to intervention, can be leveraged to understand and predict which members are likely to
discontinue their treatments so steps can be taken to lessen potential impacts to their health.
The use cases for AI are nearly endless, but these examples underscore its potential to make healthcare more accessible, effective and safe for consumers, and more streamlined for payers, providers and other stakeholders.
Where has Abarca been implementing AI and machine learning?
Nyako: Abarca is working on implementing machine learning in several ways – including to address the prior authorization and medication adherence opportunities we touched on previously. But we are continuously looking for new applications of this
technology and ways it can enrich our technology and services.
For example, we’ve incorporated it into our Fraud, Waste and Abuse (FWA) process to help identify potential cases for investigation more efficiently. We also have a program that helps to improve medication adherence by using machine learning to identify and risk-stratify patients who may become non-adherent, allowing for earlier and more
effective intervention.
Less formally, we also use AI and machine learning on an ad hoc basis for analysis to gain more insights and value from our data. It may seem simple, but this practice has a trickle-down effect that can facilitate greater understanding and innovations not only among our teammates, but for our clients and the members we serve.
What lessons are you learning that could help accelerate the use of AI and machine learning in healthcare?
Nyako: Don’t rush data exploration. It’s not the show-stealer of the process, but it is the most important part: You have to know what you’re putting into the machine-learning model. To develop a strong model, you need to understand the data’s relationship to the target and the range of values in the data, among other considerations.
The second thing I would share is the importance of communication and clear expectations. In most cases, a business subject-matter expert bringing the request to the data team doesn’t fully understand the process required. It can be surprising how
long it takes before we see the first set of results or how many rounds of adjustments are necessary to get to a usable model. Team members also need to understand that there’s no guarantee we’re going to be able to deliver usable results – some things are just unpredictable.
Ash: There are a lot of common traps that individuals and organizations can fall into when they’re working with machine learning and AI. One is focusing on technology for technology’s sake. It is important to really understand the context, how the technology is being used, what outcomes you’re looking to achieve and how you get the solution out into the world. You can’t just let the ship sail by itself. You got to give it a little bit of guidance and TLC along the way.
And that brings me to another critical lesson: Humans need to stay in the middle of these processes. In many cases, that means not only building with the end user in mind but partnering with them every step of the way. For example, a designer can create a
pharmacy tool that follows every best practice for UX, but that doesn’t mean it’s going to be able to deliver outputs in a way that is ideal for pharmacists.
Technology may have the power to transform healthcare, but meaningful evolution is not possible without proper stewardship.
What is the challenge posed by AI ‘hallucinations’ and what risks do they pose in healthcare settings?
Ash: Hallucinations occur when AI systems generate misleading or incorrect outputs based on the data they have been fed and the processes they have been trained to follow. In healthcare, if we’re feeding a system with patient data, drug data, clinical
protocols and the like, we don’t want it to make guesses. The consequences of this issue in the healthcare space can be severe – leading to misdiagnosis and patient harm – and need to be avoided at all costs. So, it’s going to be critical that we proactively work to make sure these systems are rigorously tested, validated, and monitored to minimize the risk of errors. And we also have to ensure that we’re not introducing biases or inaccuracies in the data.
Nyako: The hallucinations are mostly the result of AI’s failure to understand the question and not knowing well enough to ask for clarification. In a business context, you’re going to want to develop models specific to a task or domain to remove the bias
and make sure it’s going to interpret the questions correctly. But, in a lot of the applications that I see, there’s going to be a professional between the AI’s response and the end output. So, it’s making sure people understand AI isn’t infallible and that they need to use their professional judgment to evaluate what it’s giving back and making sure it’s reasonable.
Ash: Human oversight is paramount. Providers need to work collaboratively with data scientists and AI engineers on building these systems to achieve an optimal balance and minimize the risk. At Abarca, our mission is to influence and drive positive health outcomes and make healthcare seamless and personalized for everyone. These tools can help us get there, but we have to recognize the risks and do whatever we can to minimize them.
Photo: Yuichiro Chino, Getty Images