Industrial-Level Generative AI is Put to the Test
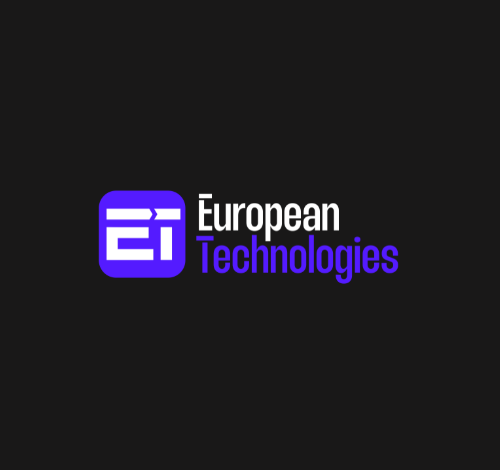
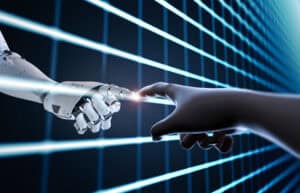
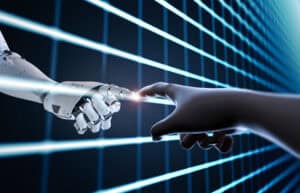
Generative AI is increasingly being used in industrial settings to add adaptability and greater intelligence to automated workflows and human-robotic interactions.
Generative AI has been made famous for its abilities to generate marketing content, script emails, and answer queries about business trends. Now, GenAI is being put to the real test: providing support to production and other heavy-duty operations. Use cases now emerging include digital twins, robots, and multi-agent systems.
The potential use cases in heavy-duty business settings is explored by Dhakshinamoorthy Renganathan of Tata Consultancy Services in a recent article published by the Industrial IoT Consortium. “Generative AI is a major step towards achieving full general-purpose AI,” he pointed out. “Generative AI provides tools which can be leveraged to build autonomic systems. Adaptive systems are a major step towards building autonomic systems.”
Industries such as telecom, mobile phones, and automotive manufacturing stand to benefit from adaptive systems built and maintained with GenAI, Renganathan observed. Such systems “can extend the lifespan of systems and can create new business models and revenue opportunities.”
For example, generative AI adds an entirely new dimension to digital twins, he illustrates. “While traditionally digital twins have been built towards bringing together structured information in engineering, production, operations, and enterprise information, few digital twins have integrated with 3D models to improve visualization aspects of the digital twins,” he explained.
“However, a significant portion of industrial knowledge is present as documents and unstructured information. GenAI contextualizes this information very well and can be used to enrich the knowledge repository of digital twins. Such a digital twin can power many use cases.”
See also: Generative AI Poised to Unleash More Independence for Humanoid Robots
Generative AI as a go-between
Generative AI also provides natural language interfaces to robots. For example, a user can “provide high-level feedback through the generative AI large language model [LLM] while monitoring the robot’s performance,” Renganathan stated. The human can apply “prompt engineering to generate policy-based codes to adapt behavior of robots” that provide guardrails to the robot’s behavior. GenAI can also play a role in guiding robots through unplanned situations.
Generative AI helps keep humans engaged with the automated processes that power today’s production systems. It “provides a mechanism to understand unknown knowns, identify root causes and generate a plan that could help in resolving a problem,” Renganathan explained. “The resolution needs to be reviewed and approved by human-in-middle.”
An interaction between a human administrator and a generative AI-powered assistant that is networked into operational systems data may go as follows:
- Query: Give me xx KPI of a manufacturing line.
- AI assistant interprets the context and invokes the digital twin for xx KPI.
- Digital twin retrieves xx KPI values from operational data store.
AI assistant invokes the digital twin for xx KPI specification. - Digital twin retrieves xx KPI specification from design context store.
- AI assistant LLM gives response with KPI value and specification to user.
When it comes to systems, designers try to build in as many contingencies as technically possible — addressing the “known knowns” and “known unknowns.” But with any industrial-level system with a lot of moving parts, there are “unknown unknowns” that creep in. Generative AI adds adaptability and greater intelligence to help meet these situations.