AI Is Driving Memory And Storage Demand And Product Introductions
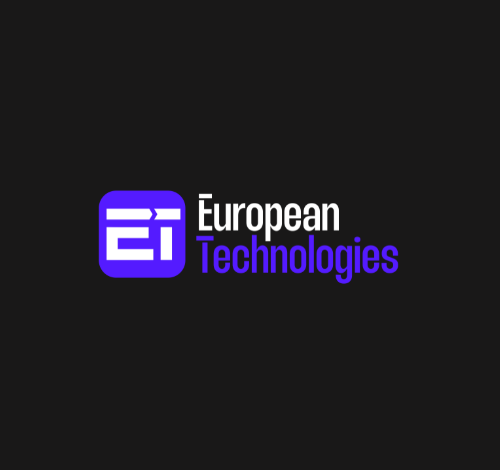
Artificial intelligence.
The growing implementation of AI is driving demand for memory and storage to support the processing of data and data retention, both for the training sets and the results of AI training used in inference engines. DRAM, NAND flash, HDD and even magnetic tape vendors will benefit from this growing demand for storage and memory. Likely also emerging non-volatile memories will also benefit, especially for end point AI inference applications.
As a consequence of this growing demand, and also because of cuts in memory and storage technology manufacturing cutbacks in the Fall of 2023, prices of these products, particularly solid-state memory and storage, have been increasing. On the volatile memory side, DRAM for high bandwidth memory (HBM) for AI applications is a particular focus. At the 2024 Computex in Taiwan Dinesh Bahal, GM or Micron Consumer and Components Group said that Samsung, SK hynix and Micron are putting a lot of effort to build HBM products to meet increased demand to support AI.
Western Digital (WDC) recently announced new products to support AI workloads and provided the interesting visual below showing the data workflow for AI applications. WDC called this a six-stage AI Data Cycle framework that defines the optimal storage infrastructures to maximize AI investments and increase the efficiency and low the cost of ownership for AI workflows.
Concept of the operations in AI workflows
This illustration shows this workflow results in a continuous and reinforcing loop of data consumption and data generation involving the processing of all types of data including text and images as well as audio and video content. This includes preparation and curation of training data, training and preparation of the inference engine to make use of the trained model. The results of this processing are new data reflecting the model training that must be stored to support AI inference.
Processing and storing this data effectively and cost efficiently can require the use of various types of storage and memory products including HBM for the actual AI training, but also various types of NAND-based solid-state storage to support the data flows needed to and from the HBM. These include higher performance NAND SSDs as well as lower performance but higher capacity SSDs. These SSDs can be called primary storage.
In addition to these SSDs, hard disk drives can provide more cost-effective secondary storage. Finally, data no longer needed for training and some results from the training might be stored in an archival media for longer term retention. This might be particularly appropriate for data (such as scientific or engineering data) that might be used for later training and analysis to find new insights and relationships.
WDC announced digital storage products that are built to support many of these elements of the storage supply chain to support AI training and inference. Among these announcements was a 32TB ePMR Enterprise HDD that the company said it was sampling to select customers. The Ultrastar DC HC690 UltraSMR (shingled magnetic recording) HDD is the highest capacity HDD currently available from WDC. Note that Seagate and Toshiba have announced 32TB HAMR (heat assisted magnetic recording) HDD without shingling.
WDC is also a manufacturer of NAND flash and SSDs (which will be spun out to a newly recreated SanDisk in the near future). The company announced various types of SSDs to support these workflows including high performance PCIe Gen5 SSDs for training and inference and a high capacity (up to 64TB) SSD for fast AI data lakes. The complete WDC AI storage portfolio is shown in the image below.
Western Digital Products for storage in AI workflows
AI workflows are driving demand for memory and storage to prepare data, train the model and create useful inference engines. This is driving announcements of storage and memory products that support the various elements of these AI workflows.