AI system poisoning is a growing threat — is your security regime ready?
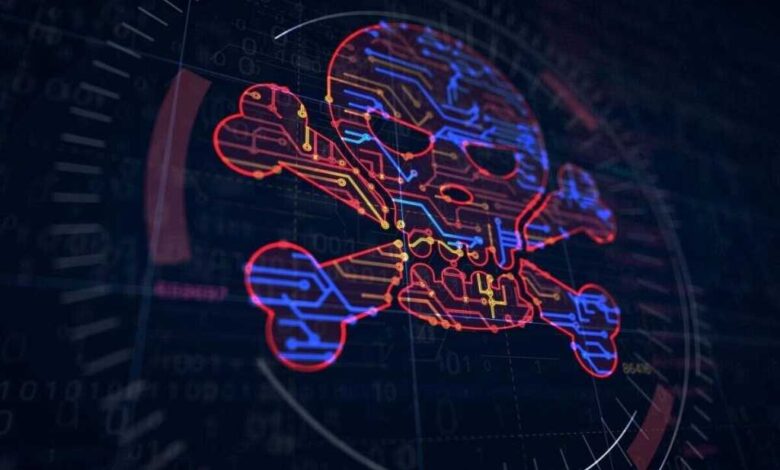
Consulting firm Protiviti recently worked with a client company experiencing an unusual attack: a hacker trying to manipulate the data being fed into one of the company’s AI systems. Company leaders are still unraveling the attack, but Protiviti managing director John Stevenson says the company suspects the hacker was trying to skew the AI system’s output.
Such attacks are not new, yet they remain outliers in the cybersecurity world. As the “2024 State of Security” report from software maker Splunk states: “AI poisoning remains a possibility, [but] has yet to become commonplace.”
That outlier status, however, is expected to change, with leading security experts predicting that hackers will increasingly target AI systems and, more specifically, will attempt to poison them by corrupting data or the models themselves.
CISOs everywhere need to prepare, as organizations of all sizes and types could be targets.
“Every company is exposed to this either through their own [in-house developed] AI models or through the third-party AI tools they use,” Stevenson says.
NIST issues warning about 4 types of poisoning attacks
The National Institute of Standards and Technology (NIST), a US agency, warned of what’s to come in a January 2024 paper. Researchers wrote that “poisoning attacks are very powerful and can cause either an availability violation or an integrity violation.”
“In particular, availability poisoning attacks cause indiscriminate degradation of the machine learning model on all samples, while targeted and backdoor poisoning attacks are stealthier and induce integrity violations on a small set of target samples,” NIST wrote.
The paper highlights four types of poisoning attacks:
- Availability poisoning, “which indiscriminately impacts the entire machine learning model and, in essence, causes a denial-of-service attack on users of the AI system.”
- Targeted poisoning, in which hackers “induce a change in the [machine learning] model’s prediction on a small number of targeted samples.”
- Backdoor poisoning, where “image classifiers can be poisoned by adding a small patch trigger in a subset of images at training time and changing their label to a target class,” with NIST further noting that although “the majority of backdoor poisoning attacks are designed for computer vision applications, this attack vector has been effective in other application domains with different data modalities, such as audio, NLP, and cybersecurity settings.”
- And model poisoning, attacks that “attempt to directly modify the trained ML model to inject malicious functionality into the model.”
NIST and security leaders note that poisoning is in addition to numerous other attack types targeting AI, such as privacy compromises as well as direct and indirect prompt injections.
“Deploying AI in your enterprise introduces a new attack surface that’s very different,” says Apostol Vassilev, a NIST research team supervisor and co-author of the NIST paper. “We have seen exploits demonstrated by academics and other researchers trying to point out potential problems, but the more this technology is deployed, the more value there is for hackers to attack it, and that’s why we’re going to get into the more consequential exploits.”
He adds: “Already we are starting to see it with an increasing pace.”
AI poisoning attacks can come from inside or outside an organization
Security experts say poisoning attacks could be launched by insiders as well as external hackers — as is the case with more conventional cybersecurity attack types.
And in another similarity to conventional attack types, “nation-states are probably one of the biggest risks here because they have the ability and resources to invest in this [type of attack],” says David Youssef a managing director at FTI Consulting and leader of the North America incident response efforts for the firm’s cybersecurity practice.
Bad actor motivations for poisoning attacks are also familiar, according to security experts, who say hackers may target AI systems for the same reasons they launch other types of cyberattacks, such as to cause disruption or damage an organization. Some say hackers may also use poisoning attacks to gain access to proprietary data or to get money.
“Could someone use this for extortion? Absolutely,” says Erik Avakian, technical counselor at Info-Tech Research Group and former state CISO for the Commonwealth of Pennsylvania. “If a hacker can compromise a system by poisoning, they can use that; they can say, ‘We poisoned the model, now you have to pay us money [to get information on what we did].’”
Primary targets will likely be tech companies that make AI systems
Although motivations like that mean any organization using AI could be a victim, Kayne McGladrey, a senior member of the Institute of Electrical and Electronics Engineers (IEEE), a nonprofit professional association, and field CISO at Hyperproof, says he expects hackers will be more likely to target the tech companies making and training AI systems.
But CISOs shouldn’t breathe a sigh of relief, McGladrey says, as their organizations could be impacted by those attacks if they are using the vendor-supplied corrupted AI systems.
A recent case illustrates the potential for far-reaching harm in such scenarios. Researchers at tech company JFrog discovered some 100 malicious machine learning models had been uploaded to Hugging Face, a public AI model repository. Researchers said in a February 2024 blog that the malicious ML models could enable threat actors to inject malicious code into users’ machines once the model is loaded, a scenario that could have rapidly compromised an untold number of user environments.
Experts say more such incidents are on the horizon.
“I’m thinking this is an emergent risk, and once AI technology scales, the poisoning threat will become more apparent,” says Mary Carmichael, managing director of risk advisory at Momentum Technology and a member of both the Emerging Trends Working Group and the Risk Advisory Committee at the governance association ISACA.
Preparing a response to AI poisoning now will help protect against what’s coming
Security experts and CISOs themselves say many organizations are not prepared to detect and respond to poisoning attacks.
“We’re a long way off from having truly robust security around AI because it’s evolving so quickly,” Stevenson says.
He points to the Protiviti client that suffered a suspected poisoning attack, noting that workers at that company identified the possible attack because its “data was not synching up, and when they dived into it, they identified the issue. [The company did not find it because] a security tool had its bells and whistles going off.”
He adds: “I don’t think many companies are set up to detect and respond to these kinds of attacks.”
A February 2024 report from ISC2, a nonprofit organization offering training and certifications for cybersecurity professionals, sheds light on whether CISOs feel prepared for what’s ahead.
The report found that 75%of more than 1,100 respondents said they are moderately to extremely concerned that AI will be used for cyberattacks or other malicious activities, with deepfakes, misinformation, and social engineering being the top three concerns for cyber professionals.
Despite that high level of concern, only 60% said they feel confident in their ability to lead their organization’s secure adoption of AI. Moreover, 41% said they have minimal or no expertise in securing AI and ML technology. Meanwhile, a mere 27% said their organization has formal policies in place on the safe and ethical use of AI.
“The average CISO isn’t skilled in AI development and doesn’t have AI skills as a core competency,” says Jon France, CISO with ISC2.
Even if they were AI experts, they would likely face challenges in determining whether a hacker had launched a successful poisoning attack.
As Vassilev explains, AI system owners and users would struggle to detect hackers, who can turn on and off behaviors without being detected. And they wouldn’t be able to look at the source code and find a trigger once a model is poisoned.
The non-deterministic nature of generative AI further challenges detection and response, he adds.
Defending against the threat to AI systems
As has long been the case in security, no single tool is going to stop poisoning attacks.
Similarly, long-standing security practices can mitigate risk, detect anomalies and speed recovery, experts said. They advise a multilayered defense strategy that includes a strong access and identity management program, a security information and event management (SIEM) system, and anomaly detection tools. “So, you know if someone has accessed your systems,” Avakian says.
Strong data governance practices as well as monitoring and oversight of AI tools are also musts, Avakian adds, “so you know what’s not real, what’s not good.”
So, too, is good vendor management to ensure that the vendors providing AI tools are doing what they should to prevent their products from falling victim to poisoning attacks, Carmichael says.
Vassilev says CISOs should work alongside other executives to identify and understand the risks (including poisoning attacks) associated with the AI tools they’re using, devise strategies to mitigate the risks that are too high, and articulate the residual risk that they’re willing to accept.
CISOs and their organizations should also know the provenance of the models they use and the lineage of their data, Vassilev says.
The NIST Adversarial Machine Learning paper provides more detailed mitigation strategies as well as more details on poisoning and other types of attacks.
Some security leaders advise CISOs to add talent specifically trained in AI security to their teams, too.
“This work requires advanced data scientists, teams who know how to evaluate training sets and models; it’s not going to be done by your average SOC teams,” Youssef says, adding that chief AI officers and CISOs should be working together on governance and security plans. “The typical protections we have in place today will not be enough, but the right approach isn’t to avoid AI but instead to understand the risk, work with the right people, evaluate it properly and take steps to minimize it.”