Best Data Analytics Books 2024: Must-Read Books
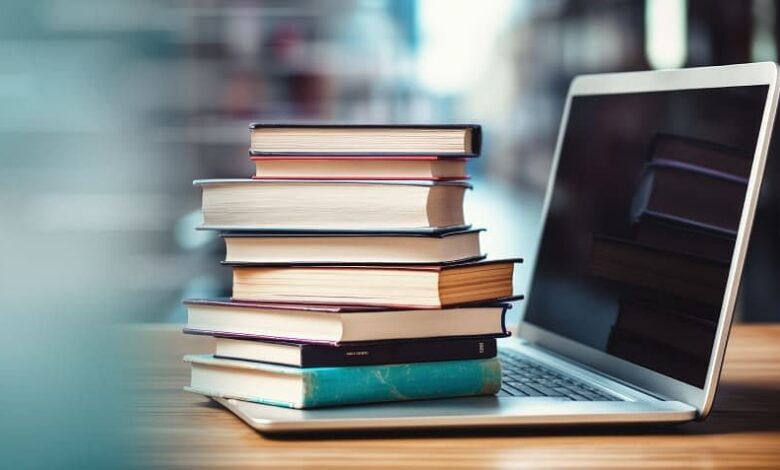
From bestsellers to must-reads, books exemplify the benefits of reading, where you find comfort, knowledge, challenge, and inspiration. Let’s revisit what the famous author Margaret Fuller once said about reading– “Today a reader, tomorrow a leader.” Good reads in any field are the roads to a successful journey toward one’s desired destination and beyond.
Today, the field of Data Analytics is surging as high as the U.S. Bureau of Labor Statistics predicts over 23% job growth for data analysts between 2020 and 2030. Nevertheless, this level of fast job growth can be brought upon when data professionals are supplemented with the best resources.
Let’s skim through one of the best resources for success in Data– the must-read Data Analyst books for beginners and experienced professionals.
Top Data Analytics Books of 2024
Books for data analysts are great ways for professionals who aspire to work in data analysis to learn about subjects, developments, and useful skills.
Here is a collection of the best data analytics books, from fundamentals to specifics, such as big data, AI, statistical programming languages, etc.
Storytelling with Data: A Data Visualization Guide for Business Professionals – Cole Nussbaum Knaflic, 2015
Cole Nussbaummer Knaflic, the CEO and founder of Storytelling With Data, wrote this remarkable data analyst book.
SWD is a book that emphasizes the importance of data storytelling in data analysis. Instead of just placing charts on report pages, data analysts should carefully choose the right chart and create a compelling story to engage their audience.
This piece is one of the must-read data analytics books for beginners, and it provides six useful steps for data storytelling.
Big Data: A Revolution That Will Transform How We Live, Work, and Think – Viktor Mayer-Schönberger, 2013
Viktor Mayer and Schönberger, domain experts, discuss the impact of big data on our world. Their book also focuses on the potential positive or negative changes in big data.
This book offers a good understanding of data analytics and its impact on various industries. It prepares readers for the big data revolution that is about to come. The book digs into the broader consequences of big data on societal aspects. It highlights the potential risks associated with digital technology. The book also provides a theoretical overview of big data’s importance in various life stages.
Python for Data Analysis: Data Wrangling with Pandas, NumPy, and IPython – Wes McKinney, 2011
The author of the Pandas library’s comprehensive book Python for Data Analysis teaches learners the fundamentals of using Python for data manipulation, processing, cleaning, and crunching. Real-world case studies are covered, along with an introduction to data science tools and instructions on how to use Matplotlib to build useful visualizations. Other techniques include loading, cleaning, manipulating, combining, and reshaping data.
Naked Statistics: Stripping the Dread from the Data – Charles Wheelan, 2012
The field of statistics is rapidly evolving into a “sexy” discipline, with applications in various fields such as politics, game shows, and medical research. Charles Wheelan’s book, Naked Statistics, focuses on the intuition behind statistical analysis, explaining key concepts like inference, correlation, and regression analysis. The book also highlights how biased parties can manipulate data and how creative researchers use natural experiment data to tackle complex questions. It is a valuable resource for those who missed Stats 101.
Data Science for Business: What You Need to Know about Data Mining and Data-Analytic Thinking – Tom Fawcett, 2013
This book, written by Foster Provost and Tom Fawcett, introduces the fundamental concepts of data science and data-analytic thinking. This data analytics book enables readers to extract valuable knowledge and business value from data. It educates readers on how to use data science techniques to help business decision-making and how to think analytically about data.
Business UnIntelligence: Insight and Innovation Beyond Analytics and Big Data – Barry Devlin, 2013
This book examines business intelligence’s past, present, and future while stressing the advantages and disadvantages of conventional methods. Dr. Devlin discusses how big data and analytics have revolutionized business intelligence today, highlighting tried-and-true methods and providing insights into how people, processes, and information interact to create competitive advantage and propel company success. Additionally, he suggests new frameworks and models for companies to enhance their future.
The Hundred-page Machine Learning Book – Andriy Burkov, 2019
This book offers a succinct introduction to machine learning in just 140 pages, making it appropriate for readers with no prior programming or statistical knowledge. Neural networks, cluster analysis, and supervised and unsupervised learning are among the important ideas covered. The book is short enough to read in one sitting, and the companion wiki provides resources and suggestions for further reading.
Artificial Intelligence: A Guide for Thinking Humans – Melanie Mitchell, 2019
Melanie Mitchell, a computer scientist, wrote this book to help us explore the historical background and people behind artificial intelligence. The book specifically draws attention to difficult ideas like neural networks, computer vision models, and NLP. It helps readers who do not require a thorough understanding of AI understand how AI affects data analytics.
Developing Analytic Talent: Becoming a Data Scientist – Vincent Granville, 2014
With his background in big data, business analytics, and predictive modeling, Granville provides helpful information in his handbook on data science and data scientists. The book discusses the significance of key information for data scientists in big data organizations. It is divided into three sections that address technological applications, case studies, tutorials, career opportunities, and the relationship between data science and other fields.
Educating decision-makers about specialized solutions and their applications also aids in the development of stronger analytics teams. Granville’s more than two decades of industrial experience offer quick suggestions for those wishing to build a data science firm.
Learning R: A Step-by-Step Function Guide to Data Analysis – Richard Cotton, 2013
This book offers a step-by-step introduction to the R language, making it an invaluable tool for non-technical learners. It covers environments, looping constructions, packages, and data structures. The book then covers the data analysis processes, including loading, cleaning, and converting data. The second section is a priceless resource for individuals unfamiliar with programming languages, as it offers further insight into exploratory analysis and modeling.
Weapons of Math Destruction – Cathy O’Neil, 2016
Cathy O’Neil’s book on data bias highlights the importance of using big data responsibly. It also discusses the consequences of machines making decisions about our lives and how algorithms often reinforce discrimination. Despite disagreements, the insights are crucial for those new to data science, ensuring future data is used for the benefit of all, not just the privileged.
Data Science and Big Data Analytics: Discovering, Analyzing, Visualizing and Presenting Data, 2014
Big Data analytics offers deeper insights and supports businesses by integrating real-time data feeds and queries. This book, by EMC Education Services, introduces key techniques and tools for Big Data analytics, guiding readers from basic methods to advanced methods like classification, regression analysis, clustering time series, and text analysis. It is suitable for business analysts, database professionals, and college graduates interested in data science or data analysis as a career field.
Too Big to Ignore: The Business Case for Big Data – Phil Simon, 2013
Phil Simon’s book Too Big to Ignore: The Business Case for Big Data explores businesses’ and local governments’ use of big data. It features case studies and quotes from professionals worldwide, providing valuable insights on turning data into intelligence and making it actionable.
The Elements of Statistical Learning – Trevor Hastie, 2001
This book thoroughly introduces statistical ideas in various industries, including marketing, biology, finance, and medicine. It employs color pictures for examples and prioritizes concepts over mathematical formulas. Classification trees, neural networks, support vector machines, boosting, and other subjects related to supervised and unsupervised learning are covered in this book, which is an invaluable tool for statisticians and data mining players.
Numsense! Data Science for the Layman: No Math Added – Kenneth Soo, 2017
This book offers a comprehensive introduction to data science, suitable for non-technical individuals. It provides clear language and visual explanations for algorithms, avoiding complex math. It is valuable for data scientists and beginners as a refresher for communicating work to business partners. The book’s algorithm explanations are useful for field communication.
Head First Data Analysis: A Learner’s Guide to Big Numbers, Statistics, and Good Decisions – Michael Milton, 2009
Head First Data Analysis is a book that teaches how to manage and analyze various types of data, including product development, marketing, sales, and entrepreneurship. It provides a unique approach to learning how to convert raw data into a vital business tool. The book uses the latest research in cognitive science and learning theory to create a visually rich format that caters to the brain’s workings, making it an efficient way to convert raw data into a valuable business tool.
SQL QuickStart Guide: The Simplified Beginner’s Guide to Managing, Analyzing, and Manipulating Data With SQL – Walter Shields, 2015
This book includes a thorough introduction to Structured Query Language (SQL), digital resources such as workbooks and reference guides, and an example database and SQL browser software. It addresses subjects like relational database communication, database structures, important SQL queries, and marketing SQL expertise to prospective employers. The book also offers suggestions on marketing newly acquired SQL abilities to possible employers.
Microsoft Excel Data Analysis and Business Modeling – Wayne L. Winston, 2004
Wayne Winston, a renowned consultant and business professor, has been teaching clients in the corporate sector and MBA students how to use Microsoft Excel for data analysis, modeling, and decision-making for over a decade. This practical guide offers real-world examples and learn-by-doing exercises to enhance data analysis and modeling expertise. The book is available as a searchable eBook and CD file for download.
FAQs
1. What are the 4 pillars of data analytics?
The four pillars of Data Analytics are descriptive, diagnostic, predictive, and prescriptive. Each pillar enhances a business’s understanding of data and ability to drive objectives through insights.
2. Is data analytics a tough job?
Working in data analytics can be tough, particularly if you’re not experienced in programming, statistics, or data processing. But if you make conscious efforts, it can also be enjoyable.
With dedication, the right resources (Data analyst books, courses from good learning platforms), stress management, and a strategic approach, data analysts can have a fruitful and rewarding career.
3. What is the salary of a data analyst in India?
India’s average annual salary for Data Analysts is ₹6.4 Lakhs, based on 97.1k salary estimates, with a range of ₹1.8 Lakhs to ₹12.8 Lakhs, with 0-6 years of experience.
4. Will AI replace data analysts?
Although AI provides useful tools, it can only support the efforts of a data analyst. Businesses will keep spending money on data analysts who can implement artificial intelligence technologies securely and confidently in the future. Rather than completely replacing data analysts, AI will advance, enhance, and make them more efficient.
5. Is data analyst still in demand?
Data analysts are in high demand in India, with 97,000 unfilled jobs annually. Demand has led to a 45% increase in Indian data analyst jobs. The U.S. Bureau of Labor Statistics predicts over 23% job growth globally for analyst professionals between 2022 and 2032.