The Mobile NetworkAI and the RAN
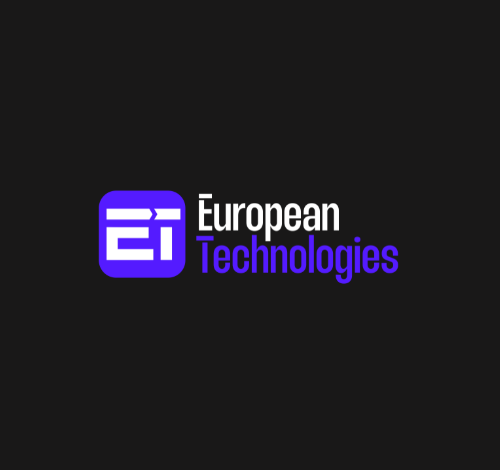
The AI-RAN Alliance launched during MWC. It said that it was aimed at “integrating artificial intelligence (AI) into cellular technology to further advance radio access network (RAN) technology and mobile networks”.
Nvidia was the lead company in terms of pushing out the announcement. Nokia, Ericsson and Samsung were representing the large RAN vendors. There were two operators – SoftBank and T-Mobile. There were the cloud players AWS and Microsoft. Arm was also present, as was “AI-native” mobile PHY software provider DeepSig, a company featured before on TMN.
AI in the RAN is far from new. SON (Self Optimising or Self Organising Networks) technology has incorporated elements of Machine Learning for years. RAN vendors incorporate machine learning and analytics into their RAN platforms to provide intelligence and control, and recently O-RAN specifications have disgaggregated such RAN controller technology into two functions that sit above the baseband, the near real time and non real time RAN Intelligent Controllers (RICs).
Nvidia itself has been seeing an opportunity for its processors to add AI capability to the RAN for a few years. An “AI-on-5G” white paper was published in June 2021. It argued that by implementing vRANs, operators could turn cell sites into AI-on-5G data centres. In December 2022 a developer blog post again exlored the concept of AI for RAN in lengthy terms, claiming this time that of all the areas that AI could impact telcos, the most profound impact would be in the RAN. It said then, “AI is transforming the RAN in four key ways: energy savings, mobility management and optimization, load balancing, and Cloud RAN.”
And the company continues to market its AI-on-5G platform – comprised of its converged accelerators, the Aerial SDK for software-defined 5G virtual radio area networks (vRANs), and a portfolio of enterprise AI applications and SDKs. It has announced that SoftBank will be experimenting with distributed data centres that can host telco and Gen AI functions.
So what is behind this new push from Nvidia and the other companies? Those in the know say it is not just about selling expensive GPUs to enable L1 processing in the Cloud RAN. It is also about build up a set of capabilities up the stack, including within the O-RAN RIC functions. It is also about enabling operators to use AI both to optimise and to monetise their cloud RAN investments.
Smartly, the Alliance divided its areas of focus into three headings:
- AI-for-RAN, where AI is used to improve RAN operations and efficiency – for example for channel estimation interpolation on a RIC platform.
- AI-on-RAN, where AI workloads run on top of the RAN infrastructure – for example running computer vision inferencing for connected drones and cameras;
- AI-and-RAN, where unused or idle compute capacity is offered back to the cloud to offer extra capacity for AI workloads.
AI-RAN Alliance demos
You can now see the demos that the Alliance was showing at MWC.
The “AI-for-RAN” demo had two use cases. One that showed how a RIC can assure slice SLAs by dynamically changing the resources dedicated to a slice to stay compliant with SLA policies, and another that shows how AI can help with channel interpolation.
The presenter says, “In the demo we’re showing the implementation of the Juniper RIC onto a Grace Hopper [Grace Hopper is Nvidia’s chip that combines the Grace CPU with the Hopper GPU] . We’re showing that an AI model trained for this kind of application greatly benefits in terms of reducing the number of SLA violations in the network.”
The second part of the demo shows AI Trained Channel interpolation and was presented by Softbank, Nvidia and Fujitsu.
Due to the complex building environment in urban areas, SINR [signal to noise ratio] is impacted by factors such as “noise”, doppler frequency shift due to moving devices, and delayed signal due to the multipath reflections. These radio signal attenuations are compensated by channel estimation. But channel estimation itself is difficult in these environments. In order to address these problems the demo shows AI and machine learning-based Channel Interpolation. The process is a bit like the way image AI can increase the resoultion of a poor quality image, by applying super resolution algorithms. In a similar way, channel interpolation can restore the original channel information using AI.
The demo presenter says the companies comfirmed about a -5 dB SINR gain and as a result achieved a 20 to 40% throughouput gain. “We believe that there is more room to apply AI and machine learning in the radio signal processing that can provide not only a better user experience but lower network cost for operators.”
A second demo, AI-on-RAN, developed by Supermicro, Radisys, NVIDIA, and Fujitsu, showed how an AI application can sit on the vRAN compute infrastructure. The example given was a computer vision applications, using YOLO Deepstream Metropolis application from Nvidia on the same MGX cluster that is running vRAN software from Radisys. A connected 5G camera streamed data into the visual inference application. Conceptually, AI-on-RAN looks like MEC (Multi Access Edge Computing, formerly Mobile Edge Compute) by another name. It sites applications that are enriched by RAN data and capabilities on RAN compute.
A third demo showed AI-and-RAN, which is the concept that uses an API to inform cloud infrastructure when there is spare capacity available on RAN compute.
This demo showed how free resources from a vRAN instance could be used for AI workloads, with the cloud sending tasks to clusters when computing resources are available. This demo is presented by Supermicro, Radisys, NVIDIA and Aarna Networks.
The idea here is that operators can monetise their 5G vRAN investements. An SMO looks at the vRAN workload and communicates with the cluster agent to advertise free resources to the cloud.
NOKIA
At the moment we simply don’t know which feature will be using that hardware, but sooner or later we will pick it up
Nvidia’s announcement of the Alliance was given a boost by Nokia, which said it was working in partnership to explore use of the company’s CPU and GPUs for vRAN processing. There was some confusion as to whether Nokia would be using Marvell for L1 RAN acceleration – the press release hinted yes, Nokia later clarified no, not for now – but there was no confusion that Nokia sees potential for using Nvidia’s Grace CPU “superchip” for Layer 2 and above in Cloud RAN deployments.
Speaking to TMN, Brian Cho who is CTO for Europe, said that the company’s current baseband unit that it launched in mid-2023, which is based on its Reef Shark chipset containing Marvell’s Octeon 10 chip, does include some capability to carry out AI/ML processing.
“Obviously, the majority of the portion we implemented for telco function, but we already included a little bit about the Arm core and the cache especially designed for AI inference. In the previous generation we didn’t put that because we didn’t expect that AI would be picking up; at the moment we simply don’t know which feature will be using that hardware, but sooner or later we will pick it up.”
Cho said that moving to an architecture where telco functions run as multi tenants on a cloud architecture that also supports AI processing is a long way off, but it is something that is being looked at in the long term.
“I think telco vendors already anticipate some of the AI/ML function residing inside of the gNodeB. The other extreme could be you know, this entire hardware is equipped for AI and ML and then we are using the same hardware architecture for telco functions. Quite coincidentally, this GPU does all this parallelisation, the hardware architecture is very good for graphic processing, very good for AI and very good also for LDPC on the channel coding – there are commonalities. So, you know, there is a possibility.”
Indeed some are already investigating just that possibility. As well as the Fujitsu work with SoftBank, in a 2023 demonstration Nvidia collaborated with Radisys and Aarna Networks to showcase RAN-in-the-Cloud, a 5G radio access network hosted as a service in multi-tenant cloud infrastructure running as a containerised solution alongside other applications. The Proof-of-Concept includes an orchestrator from Aarna and Radisys to dynamically manage 5G RAN workloads and 5G Core applications and services on NVIDIA GPU accelerators and architecture.
“It’s been there since 2021,” the spokesperson said, “But being a conservative industry people haven’t used it that much.”
MARVELL
When Cho mentions Nokia’s existing baseband containing AI/ML processing capability, he is referring to an ML/AI Acellerator capability built in by Marvell to its Octeon 10 chip.
In the same week as the AI-RAN Alliance announcement and also Nokia’s nVidia announcement, Marvell said that it was open sourcing this ML/AI Accelerator Software. [As a reminder, this is software that it has integrated into its vRAN chips, which are used by the likes of Nokia and Samsung for L1 acceleration.]
Through contributions accepted to the Apache TVM (Tensor Virtual Machine) project, developers can use Open-source tools to build machine learning (ML) models that can be executed in OCTEON 10’s integrated ML/AI acceleration engine, simplifying the adoption of these models for 5G Radio Access Network (RAN) optimisation.
A Marvell spokesperson said to TMN that vendors to this point had not done a lot with the AI/ML capability that is built into the Octeon 10 CN106 chipset, which was its first SKU in the Octeon 10 family and contains up to 24 Arm Neoverse N2 server processor cores, inline AI/ML acceleration, an integrated 1-terabit switch and vector packet processing (VPP) hardware accelerators.
“It’s been there since 2021,” the spokesperson said, “so that if a carrier or OEM had an AI application to run it wouldn’t need a GPU.”
Examples could be running applications to optimise the network, say for power efficiency. “But being a conservative industry people haven’t used it that much. So we opened the APIs, made some models, turned it over to the Linux Foundation. Now it’s open, carriers and OEMs can gain experience with it, and it also brings in third party developers.”
An advantage over the Grace Hopper architecture, Marvell says, is that this is out in the field in Samsung, Nokia and Fujitsu units.
Marvell’s announcement was welcomed by Nokia. “We are proud to be the first vendor to incorporate AI/ML into our ReefShark SoCs and AirScale portfolio. This technology allows us to differentiate our solutions and offer the best spectral efficiency and cell edge performance in the industry. We are now at the forefront of bringing machine learning technology to our customers and exploring its enormous possibilities ahead of the 6G era,” said Mark Atkinson, Head of RAN at Nokia.
One of the big things we’re saying is you really need to be putting out a compute infrastructure that can run a telco network as a workload
Nvidia
As for Nvidia, what does it see the Alliance achieving? Chris Penrose, Global Head of Business Development, Telco, says, “One of the biggest challenges with the telco network today is the fact that it’s purpose built to do what it does, but it means during the off peak hours it’s highly underutilised. We ran some studies that literally only 40 to 50% of time is the is the network fully utilised – compare that to a Cloud environment where you’re seeing 90-95% utilisation of the compute.
“And so one of the big things we’re saying is you really need to be putting out a compute infrastructure that can run a telco network as a workload – and you can prioritise it as the primary workload – and use the time you have excess capacity for Gen AI or other AI applications.” That, Penrose says, would create a very different monetisation model for operators.
It’s a concept that has some backers, at least conceptually, especially as the RAN air interface itself becomes more AI-reliant. BT’s Mark Henry, Director of Network and Spectrum Strategy, says, “Nvidia’s platform looks really interesting. I worry about GPUs and energy, but we would benchmark and work that out. But the interesting thing is if we centralise the RAN with a GPU platform like Grace Hopper we’ve got a GPU cloud available in the network for training. And the network is not heavily used between the hours of 1am and 6am. So for the first time we can actually make use of all of that compute resource. The dream of cloud was to abstract and then share the resources; we might get to do that in the RAN and that might come with a GPU cloud, or some other form of acceleration.”
“And I think with 6G, although it’s a long way off, this concept of an AI-based air interface and having this accelerated cloud to train and optimise it – it’s a big opportunity.”
Nor does Nvidia see its role just as burrowing into L1 processing deep in the baseband. The RIC is also absolutely part of Nvidia’s discussion. Penrose says, “There’s a whole set of things we’ll be announcing at GTC conference [happening from 18 March, 2024] where we’ll be announcing all the different layers , from going beyond L1 into L2 and above, all the different ways in which we really kind of look at as a full stack problem.”
“We started with L1 because it was the most challenging, but you know, we’re definitely getting into a whole different set of applications in L2 and above where can we actually apply accelerated computing to make those things run way more efficiently and effectively.”
AWS
Ishwar Parulkar, Chief Technologist, Telecom and Edge Cloud at AWS Telco vertical, agrees that the RIC can be a place where AI and the RAN “come together.”
“We created the telco vertical to look at how telcos as a business can build networks in a different way, with cloud technology. AI is a big area which we’ve been investing in for years, and in the past year with Gen AI has taken on a new life. One area where we see applications of both these come together is in the RAN space. To me there are several technical problems there that lend themselves very nicely to AI and ML. So for example what used to be called SON – a lot of these algorithms are heuristic and lend themselves well to ML. On the other hand RAN architecture has evolved to enable things like that. The RIC is an implementaiton of the stack that has already taken into account that telcos might need to build apps like that on top of the RAN.”
For Parulkar, the cloud will be central to this confluence.
“The RIC has an interface to xApps and rApps and to build those you need ML toolsets and services which we have. Our approach to the edge is that local zones, outposts, RAN servers – these are not just servers, they are pieces of the cloud outside of the Region but where you can run the same services that run in the Region. So it comes with the ML stack, instances that are purpose built for inference and training if you choose to use them.”