The Potential of Generative AI in 5G Networks: Enhanced User Experiences with Content Optimization, Virtualization, and Network Slicing
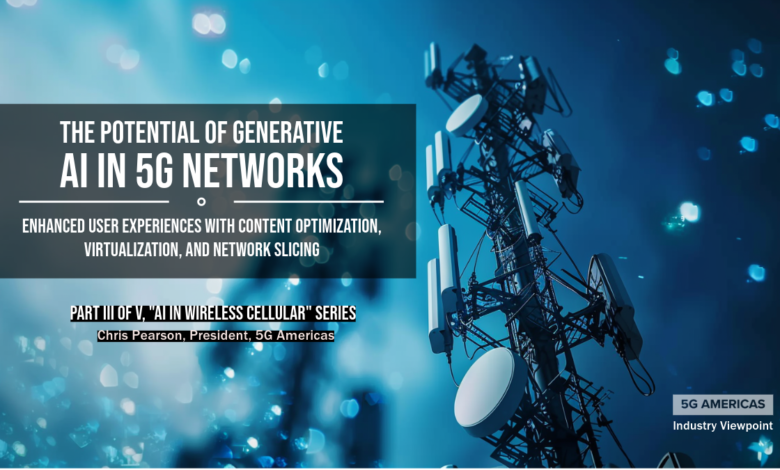
This is Part III of a five-part blog series on AI/ML in 5G and future wireless cellular networks. Part I and Part II are here.
Chris Pearson, President, 5G Americas (April 2024) – Welcome to Part III of our five-part series on the potentially pivotal role of generative AI in shaping the future of 5G and subsequent wireless cellular networks. Building upon the insights presented in Parts I and II, this blog post delves into potential future cutting-edge developments in generative AI and its possible groundbreaking impact on content optimization, network virtualization, and the innovative concept of network slicing in the realm of high bandwidth and low latency 5G networks. The blog looks out toward the future possibilities of generative AI and its possible impact on wireless cellular networks.
Generative AI, by analyzing user behaviors, network conditions, and content preferences, plays a crucial role in optimizing and personalizing content delivery, making the digital experience smoother and more enjoyable for users. One of its key innovations is predictive content caching, where AI anticipates user demand for content, pre-caching it at the network edge to significantly reduce latency. Imagine the scenario: before a major sports event, AI forecasts a surge in viewership, ensuring that the content is readily accessible, minimizing delays and buffering. While content delivery networks (CDNs) have been around for decades, predictive AI can be used to greatly refine a network’s capabilities to assess when and how content should be delivered.
Additionally, generative AI can be used to dynamically adjust streaming quality based on network congestion and user device capabilities, ensuring uninterrupted viewing. For instance, during network strain caused by multiple household internet activities, AI seamlessly lowers and then restores video quality, maintaining a smooth viewing experience without any manual adjustment needed from the user.
AI customization of content delivery paths for users based on their internet speed and content preferences ensures a tailored content experience for each user, whether providing high-definition videos for those with high-speed 5G connections or optimizing for lower bandwidth without compromising content accessibility. In interactive environments like online gaming or VR, AI adjusts content complexity and detail in real-time to match the user’s network capacity, providing an immersive experience without lag.
AI can also be used to enhance the user experience further by optimizing ad timing and placement based on user engagement patterns and network conditions, ensuring ads are less intrusive and more effective. It also could dynamically convert content into the most suitable format for users on the move or with varying device capabilities, improving accessibility and satisfaction. For instance, an example of this would be a streaming music service that uses generative AI to insert ads in a way that minimizes listener disruption and optimizes ad performance. By analyzing listener habits, the AI predicts less disruptive moments for ad insertion, such as after a set of three upbeat songs when a listener’s engagement is highest. For users identified with fluctuating network speeds, it chooses audio ads of shorter duration and lower bitrate during periods of low bandwidth, ensuring ads are delivered smoothly and listener satisfaction is maintained.
Beyond content delivery, generative AI could revolutionize network management through the concepts of virtualization and network slicing. By deploying virtualized network functions at the network edge, AI could ensure low-latency services for applications requiring real-time data processing, crucial for technologies like autonomous vehicles and IoT devices. Generative AI could aid in planning network upgrades and maintenance with minimal service disruption, testing changes in a virtual environment to predict their impact and determine the optimal implementation strategy.
For instance, through intelligent resource allocation, a generative AI system might continuously monitor the virtualized network’s performance, user demand, and service requirements. It predicts patterns in data traffic and automatically reallocates resources such as processing power, storage, and bandwidth across different virtual network functions (VNFs) and services. For instance, if the AI anticipates a spike in video calls in the evening, it dynamically increases resources allocated to video streaming services, ensuring high-quality calls without manual intervention.
Through predictive anomaly detection, AI proactively addresses potential network failures, enhancing reliability and minimizing downtime. It also dynamically enhances network security, generating and applying updated policies across the network to defend against evolving threats. By reducing 5G networks’ energy consumption through intelligent adjustments of power states and decommissioning non-critical functions during low usage periods, AI strikes a balance between efficiency and user experience.
Ultimately, in the future this could potentially lead to automated network healing, where a generative AI model detects anomalies indicating potential failures in a virtualized network component, such as a virtual router. Before the failure affects service, the AI generates a temporary routing solution that bypasses the problematic component and initiates a virtual “healing” process to either restart or redeploy the affected function without service disruption. This proactive approach could minimize downtime and enhance network reliability.
In a virtualized 5G network, generative AI can also be used to enhance security measures dynamically. In the future, AI could generate and update security policies based on evolving threat landscapes and apply these policies across virtualized network functions. For example, if a new type of DDoS attack is detected, the AI can immediately simulate potential defenses and deploy the most effective one across the network’s virtual firewalls, ensuring robust security with minimal human intervention.
At the apex of these capabilities lies a desired feature for many network operators: the ability to dynamically create network slices to accommodate specific needs, such as low-latency gaming or high-volume streaming. Generative AI can be used to anticipate data traffic patterns and dynamically reallocate network resources to maintain optimal service quality without manual intervention.
From a technical perspective, there are still many hurdles to overcome for network slicing to become a reality in the manner operators and customers envision. 5G Americas covered several of these challenges in our white paper, Commercializing 5G Network Slicing, but advances in Generative AI do offer potential new avenues to infuse the network with more intelligence, making network slicing even more dynamically predictive and responsive.
For instance, network slicing generally involves several steps, including data collection and analysis, slice design and creation, real-time optimization and automated configuration, slice lifecycle management, security and slice isolation, and inter-slice coordination.
During data collection and analysis, Generative AI has the potential to collect data from User Equipment (UE), the RAN, the Core Network (CN), and the Transport Network (TN). This includes performance metrics, user behavior patterns, device types, and service demands. Machine learning algorithms then analyze this data to identify patterns, predict demand, and understand the specific requirements for different types of network slices, such as latency sensitivities for Ultra-Reliable Low Latency Communication (URLLC) or bandwidth demands for Enhanced Mobile Broadband (eMBB).
Slices can then be designed and created in the three parts of the network:
- RAN Slicing: In the RAN, network slicing starts with generative AI designing virtualized RAN functions (vRAN) tailored to specific use cases. This involves configuring the gNodeB (Next Generation NodeB) with specific radio resources, scheduling policies, and Quality of Service (QoS) parameters for each slice.
- Core Network Slicing: Within the CN, the AI customizes the control plane and user plane functions (CP and UP functions) for each slice. This includes setting up the Access and Mobility Management Function (AMF) for connection and mobility management per slice, and the Session Management Function (SMF) and User Plane Function (UPF) for data handling and routing specific to the slice’s requirements.
- Transport Network Slicing: For the TN, generative AI configures the Software-Defined Networking (SDN) controllers to create isolated paths that meet the latency, throughput, and reliability requirements of each slice, ensuring that the transport layer supports the differentiated services of the slices above.
Using predictive models, the AI could then forecast the need for adjustments in slice configurations, such as reallocating spectrum in the RAN or adjusting the capacity of UPFs in the core network. Changes are dynamically applied through orchestration tools, such as the NFV Orchestrator (NFVO) for VNF lifecycle management and the SDN controllers for TN configuration. This ensures that each slice is optimally configured in real-time to meet its SLA.
Finally, each slice can then be measured and managed throughout its lifecycle, using metrics collected from virtualized network functions (VNFs). This is managed through the VNF Manager (VNFM) and NFVO in coordination with the SDN controllers. AI can then generate security policies tailored to the needs and risk profiles of each slice, applied across the network via policy control functions like the Policy Control Function (PCF) in the core network.
But again, technical challenges and hurdles remain in the integration of generative AI in this process.Ensuring coordination and non-interference between slices is critical. AI algorithms must be developed to account for the complex interactions between slices, especially when they share physical resources.As the number of slices and their complexity grow, maintaining scalability without compromising performance or security becomes a challenge. Generative AI must continuously optimize resource utilization and slice configurations to meet evolving demands.Finally,adhering to global standards (e.g., those set by the 3GPP) while ensuring compatibility with legacy and future technologies is essential for the seamless operation of network slices across different vendors and technologies.
This blog has looked to the future and has touched on the transformative potential of generative AI in enhancing 5G networks through innovative content delivery and advanced network management strategies. As we continue to explore the role of generative AI in meeting the diverse needs of modern digital services, its ability to dynamically manage and optimize network slicing, ensuring efficient and reliable service delivery, marks a possible significant step forward in the evolution of 5G technologies. Stay tuned for the remaining parts of this series, where we will explore more deeply the intricacies and future prospects of AI/ML in the context of 5G and beyond, paving the way for a more connected, efficient, and user-centric digital world.
In Part IV, I will take a deeper dive into how generative AI can be used to secure 5G networks.
-Chris