Former OpenAI Board Member: AI Needs Reporting Mechanism
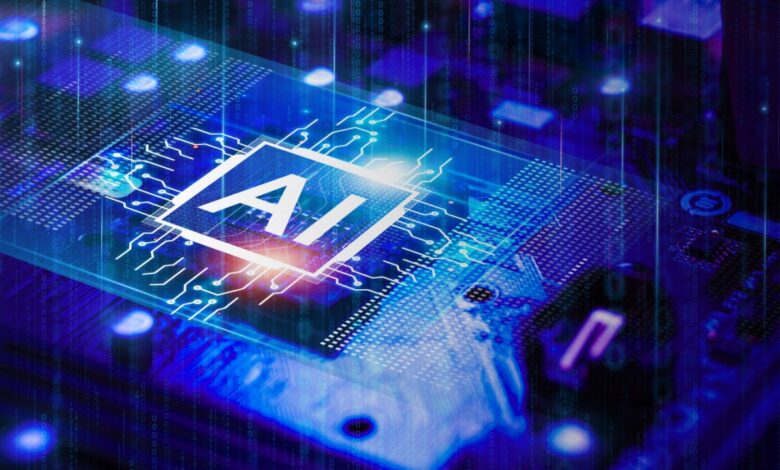
There should be a reporting mechanism in place for incidents when artificial intelligence (AI) goes wrong, just as there is for airplane crashes, a former OpenAI board member said Tuesday (April 16).
Helen Toner, who is a director at Georgetown University’s Center for Security and Emerging Technology, said this in a talk at the TED conference, Bloomberg reported Tuesday.
Toner resigned from OpenAI last year after supporting the ouster of the company’s CEO, Sam Altman, which was later reversed. Prior to that event, Altman attempted to have her removed from the board after she co-authored a paper critical of OpenAI’s safety practices, according to the report.
During her Tuesday TED talk, Toner said AI companies should have to “share information about what they’re building, what their systems can do, and how they’re managing risks,” per the report.
Toner also said that this information to be shared with the public should be audited, so that the AI companies are not the only people who check the information they provide, according to the report.
One example of a case in which the technology could go wrong would have to do with its use in an AI-enabled cyberattack, the report said.
Toner said she has been working on policy and governance issues around AI for eight years, and has had an inside look at how both government and industry have been working to manage the technology, adding, “I’ve seen a thing or two as well,” per the report.
In a June 2023 interview with CNBC, Toner said there is a question among industry players about whether there should be a new regulatory agency focused specifically on AI.
“Should you be handling this with existing regulatory authorities that work in specific sectors, or should there be something centralized for all kinds of AI?” Toner said to CNBC.
In another recent development in this space, the U.S. and the U.K. joined forces in early April to develop safety tests for advanced AI.
The agreement aims to align the two countries’ scientific approaches and accelerate the development of robust evaluation methods for AI models, systems and agents.