A Beacon of Hope in Antibiotic Resistance Crisis
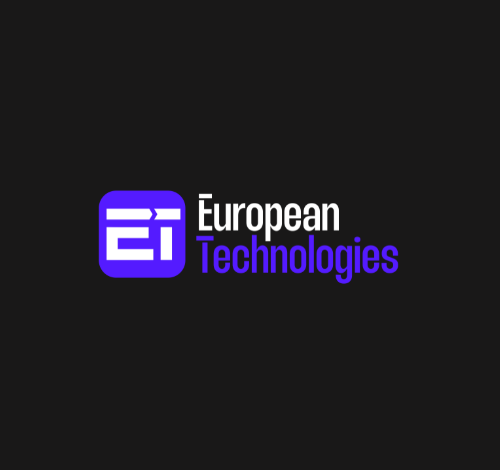
The escalating global health crisis of antibiotic resistance, responsible for around five million fatalities yearly, is being challenged with the innovative prowess of artificial intelligence (AI). Researchers from Stanford University School of Medicine and McMaster University pioneered life-saving research that could be a game-changer in combating drug-resistant diseases.
Their groundbreaking study, published in Nature Machine Intelligence, introduced SyntheMol, an AI model that has created new chemical structures and blueprints for six potential drugs targeting the tough Acinetobacter baumannii bacteria. This organism is a major cause of lethal infections due to its resilience against current antibiotics.
Before AI, scientists had limited methodologies that merely scraped the surface of the vast potential antibacterial compounds. However, SyntheMol leverages over 130,000 molecular components and validated chemical reactions to not only design novel drug compounds but also provide the recipe for their synthesis.
In under nine hours, this sophisticated AI technology produced approximately 25,000 potential antibiotics and their respective synthetic procedures. To outsmart bacteria’s rapid adaptation, the new compounds were filtered to ensure they were structurally distinct from existing antibiotics, reducing the potential for resistance development.
This study signifies a huge leap towards resolving the antibiotic resistance crisis. It shows that AI can unlock new dimensions in drug discovery, vastly expanding our arsenal against resistant pathogens. As SyntheMol continues to learn and refine its capabilities, we step closer to a future where antibiotic effectiveness is retained and enhanced, saving millions of lives.
Current Market Trends:
The application of AI in healthcare is a rapidly growing field, with a significant trend towards its use in drug discovery and development. Pharmaceutical companies and research institutions are increasingly investing in AI to capitalize on its potential for speeding up the traditionally time-consuming and expensive process of drug development. AI-driven platforms are now being deployed to identify novel drug candidates, predict their efficacy, and optimize their chemical properties to combat various diseases, including antibiotic-resistant infections.
Forecasts:
The global market size for artificial intelligence in healthcare is projected to experience robust growth in the coming years. According to recent market analysis reports, it is expected that AI in the healthcare market will reach multi-billion-dollar valuations by the mid-2020s. This growth is partly fuelled by the urgent need for new antibiotics due to the rising threat of antibiotic resistance.
Key Challenges and Controversies:
One of the key challenges in integrating AI into antibiotic drug discovery is the need for high-quality, comprehensive data to train AI models effectively. The quality and breadth of data directly influence the AI’s capacity to generate innovative and effective drug candidates. There’s also the controversy surrounding the “black box” nature of AI algorithms; the decision-making processes of these models are not always transparent, which may raise concerns about their trustworthiness and the reproducibility of their findings.
Important Questions Relevant to the Topic:
– How will AI change the traditional landscape of antibiotic drug discovery and development?
– What implications does the use of AI in this field have for regulatory approval processes?
– How can AI be integrated with existing research methodologies to yield the best outcomes?
Advantages:
The integration of AI in the fight against antibiotic resistance presents several advantages:
– Speed: AI can process vast amounts of data and perform complex tasks much faster than traditional research methods.
– Innovation: AI’s ability to identify novel chemical structures can lead to the discovery of antibiotics that are different from existing ones, potentially outmaneuvering resistant pathogens.
– Predictive Analytics: AI can predict the efficacy and resistance potential of new drug candidates, which may reduce the trial and error in the drug development process.
Disadvantages:
There are, however, disadvantages to consider:
– Data Dependency: The success of AI is heavily reliant on the availability of large and diverse datasets.
– Complexity: Designing and implementing AI models requires highly specialized knowledge and technical expertise.
– Regulatory Hurdles: Regulatory frameworks may struggle to keep pace with the rapid advancements in AI, potentially leading to delays in drug approval and market entry.
For further reading:
– Nature
– Stanford University
– McMaster University
To ensure validity, the links provided are to the main domains of well-established and reputable organizations, such as the journal Nature, and the universities involved in the AI research for combating antibiotic resistance.