A systematic review and meta-analysis of artificial intelligence versus clinicians for skin cancer diagnosis
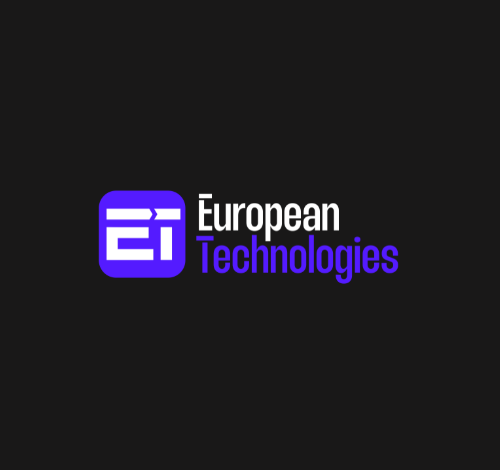
Lakhani, N. A. et al. Total body skin examination for skin cancer screening among U.S. adults from 2000 to 2010. Prev. Med. 61, 75–80 (2014).
Wu, Y. et al. Skin cancer classification with deep learning: A systematic review. Front Oncol. 12, 893972 (2022).
Jones, O. T. et al. Artificial intelligence and machine learning algorithms for early detection of skin cancer in community and primary care settings: a systematic review. Lancet Digit Health 4, e466–e476 (2022).
Sangers, T. E. et al. Position statement of the EADV Artificial Intelligence (AI) Task Force on AI-assisted smartphone apps and web-based services for skin disease. J. Eur. Acad. Dermatol Venereol https://doi.org/10.1111/jdv.19521 (2023).
Whiting, P. F. QUADAS-2: A revised tool for the quality assessment of diagnostic accuracy studies. Ann. Intern Med. 155, 529 (2011).
Cabitza, F. et al. The importance of being external. methodological insights for the external validation of machine learning models in medicine. Comput. Methods Prog. Biomed. 208, 106288 (2021).
Shung, D., Simonov, M., Gentry, M., Au, B. & Laine, L. Machine learning to predict outcomes in patients with acute gastrointestinal bleeding: A systematic review. Dig. Dis. Sci. 64, 2078–2087 (2019).
Steyerberg, E. W. & Harrell, F. E. Prediction models need appropriate internal, internal–external, and external validation. J. Clin. Epidemiol. 69, 245–247 (2016).
Piccolo, D. et al. Dermoscopic diagnosis by a trained clinician vs. a clinician with minimal dermoscopy training vs. computer-aided diagnosis of 341 pigmented skin lesions: a comparative study. Br. J. Dermatol 147, 481–486 (2002).
The International Skin Imaging Collaboration. https://www.isic-archive.com/
Tschandl, P. The HAM10000 dataset, a large collection of multi-source dermatoscopic images of common pigmented skin lesions. Sci. Data 14, 180161 (2018).
Friedman, R. J. et al. The diagnostic performance of expert dermoscopists vs a computer-vision system on small-diameter melanomas. Arch. Dermatol 144, 476–482 (2008).
Marchetti, M. A. et al. Results of the 2016 International Skin Imaging Collaboration International Symposium on Biomedical Imaging challenge: Comparison of the accuracy of computer algorithms to dermatologists for the diagnosis of melanoma from dermoscopic images. J. Am. Acad. Dermatol 78, 270 (2018).
Brinker, T. J. et al. Comparing artificial intelligence algorithms to 157 German dermatologists: the melanoma classification benchmark. Eur. J. Cancer 111, 30–37 (2019).
Brinker, T. J. et al. Deep neural networks are superior to dermatologists in melanoma image classification. Eur. J. Cancer 119, 11–17 (2019).
Maron, R. C. et al. Artificial intelligence and its effect on dermatologists’ accuracy in dermoscopic melanoma image classification: Web-based survey study. J. Med. Internet Res 22, e18091 (2020).
Lee, S. et al. Augmented decision-making for acral lentiginous melanoma detection using deep convolutional neural networks. J. Eur. Acad. Dermatol Venereol. 34, 1842–1850 (2020).
Marchetti, M. A. et al. Computer algorithms show potential for improving dermatologists’ accuracy to diagnose cutaneous melanoma: Results of the International Skin Imaging Collaboration 2017. J. Am. Acad. Dermatol 82, 622–627 (2020).
Fink, C. et al. Diagnostic performance of a deep learning convolutional neural network in the differentiation of combined naevi and melanomas. J. Eur. Acad. Dermatol Venereol. 34, 1355–1361 (2020).
Tognetti, L. et al. A new deep learning approach integrated with clinical data for the dermoscopic differentiation of early melanomas from atypical nevi. J. Dermatol Sci. 101, 115–122 (2021).
Haenssle, H. A. et al. Man against machine reloaded: Performance of a market-approved convolutional neural network in classifying a broad spectrum of skin lesions in comparison with 96 dermatologists working under less artificial conditions. Ann. Oncol. 31, 137–143 (2020).
Pham, T. C., Luong, C. M., Hoang, V. D. & Doucet, A. AI outperformed every dermatologist in dermoscopic melanoma diagnosis, using an optimized deep-CNN architecture with custom mini-batch logic and loss function. Sci. Rep. 11, 17485 (2021).
Yu, Z. et al. Early melanoma diagnosis with sequential dermoscopic images. IEEE Trans. Med. Imaging 41, 633–646 (2022).
Ferris, L. K. et al. Computer-aided classification of melanocytic lesions using dermoscopic images. J. Am. Acad. Dermatol 73, 769–776 (2015).
Tschandl, P., Kittler, H. & Argenziano, G. A pretrained neural network shows similar diagnostic accuracy to medical students in categorizing dermatoscopic images after comparable training conditions. Br. J. Dermatol. 177, 867–869 (2017).
Yu, C. et al. Acral melanoma detection using a convolutional neural network for dermoscopy images. PLoS One 13, e0193321 (2018).
Phillips, M. et al. Assessment of accuracy of an artificial intelligence algorithm to detect melanoma in images of skin lesions. JAMA Netw. Open 2, e1913436 (2019).
Dreiseitl, S., Binder, M., Hable, K. & Kittler, H. Computer versus human diagnosis of melanoma: evaluation of the feasibility of an automated diagnostic system in a prospective clinical trial. Melanoma Res. 19, 180–184 (2009).
Winkler, J. K. et al. Monitoring patients at risk for melanoma: May convolutional neural networks replace the strategy of sequential digital dermoscopy? Eur. J. Cancer 160, 180–188 (2022).
Tschandl, P. et al. Comparison of the accuracy of human readers versus machine-learning algorithms for pigmented skin lesion classification: an open, web-based, international, diagnostic study. Lancet Oncol. 20, 938–947 (2019).
Minagawa, A. et al. Dermoscopic diagnostic performance of Japanese dermatologists for skin tumors differs by patient origin: A deep learning convolutional neural network closes the gap. J. Dermatol 48, 232–236 (2021).
Tschandl, P. et al. Human-computer collaboration for skin cancer recognition. Nat. Med. 26, 1229–1234 (2020).
Combalia, M. et al. Validation of artificial intelligence prediction models for skin cancer diagnosis using dermoscopy images: the 2019 International Skin Imaging Collaboration Grand Challenge. Lancet Digit Health 4, e330–e339 (2022).
Wang, S. Q. et al. Deep learning-based, computer-aided classifier developed with dermoscopic images shows comparable performance to 164 dermatologists in cutaneous disease diagnosis in the Chinese population. Chin. Med. J. (Engl.) 133, 2027–2036 (2020).
Lucius, M. et al. Deep neural frameworks improve the accuracy of general practitioners in the classification of pigmented skin lesions. Diagnostics (Basel) 10, 969 (2020).
Zhu, C. Y. et al. A deep learning based framework for diagnosing multiple skin diseases in a clinical environment. Front Med. (Lausanne) 8, 626369 (2021).
Van Molle, P. et al. The value of measuring uncertainty in neural networks in dermoscopy. J. Am. Acad. Dermatol 87, 1191–1193 (2022).
Hekler, A. et al. Superior skin cancer classification by the combination of human and artificial intelligence. Eur. J. Cancer 120, 114–121 (2019).
Maron, R. C. et al. Systematic outperformance of 112 dermatologists in multiclass skin cancer image classification by convolutional neural networks. Eur. J. Cancer 119, 57–65 (2019).
Winkler, J. K. et al. Collective human intelligence outperforms artificial intelligence in a skin lesion classification task. J. Dtsch Dermatol Ges. 19, 1178–1184 (2021).
Ba, W. et al. Convolutional neural network assistance significantly improves dermatologists’ diagnosis of cutaneous tumours using clinical images. Eur. J. Cancer 169, 156–165 (2022).
Han, S. S. et al. Classification of the clinical images for benign and malignant cutaneous tumors using a deep learning algorithm. J. Investig. Dermatol. 138, 1529–1538 (2018).
Fujisawa, Y. et al. Deep-learning-based, computer-aided classifier developed with a small dataset of clinical images surpasses board-certified dermatologists in skin tumour diagnosis. Br. J. Dermatol. 180, 373–381 (2019).
Han, S. S. et al. Keratinocytic skin cancer detection on the face using region-based convolutional neural network. JAMA Dermatol 156, 29–37 (2020).
Huang, K. et al. Assistant diagnosis of basal cell carcinoma and seborrheic keratosis in Chinese population using convolutional neural network. J. Health. Eng. 2020, 1713904 (2020).
Polesie, S. et al. Discrimination between invasive and in situ melanomas using clinical close-up images and a de novo convolutional neural network. Front Med (Lausanne) 8, 723914 (2021).
Chang, W. Y. et al. Computer-aided diagnosis of skin lesions using conventional digital photography: A reliability and feasibility study. PLoS One 8, e76212 (2013).
Zhao, X. Y. et al. The application of deep learning in the risk grading of skin tumors for patients using clinical images. J. Med. Syst. 43, 283 (2019).
Pangti, R. et al. Performance of a deep learning-based application for the diagnosis of basal cell carcinoma in Indian patients as compared to dermatologists and nondermatologists. Int J. Dermatol 60, e51–e52 (2021).
Agarwala, S., Mata, D. A. & Hafeez, F. Accuracy of a convolutional neural network for dermatological diagnosis of tumours and skin lesions in a clinical setting. Clin. Exp. Dermatol 46, 1310–1311 (2021).
Kim, Y. J. et al. Augmenting the accuracy of trainee doctors in diagnosing skin lesions suspected of skin neoplasms in a real-world setting: A prospective controlled before-and-after study. PLoS One 17, e0260895 (2022).
Han, S. S. et al. Assessment of deep neural networks for the diagnosis of benign and malignant skin neoplasms in comparison with dermatologists: A retrospective validation study. PLoS Med. 17, e1003381 (2020).
Han, S. S. et al. Augmented intelligence dermatology: Deep neural networks empower medical professionals in diagnosing skin cancer and predicting treatment options for 134 skin disorders. J. Investig. Dermatol. 140, 1753–1761 (2020).
Jinnai, S. et al. The development of a skin cancer classification system for pigmented skin lesions using deep learning. Biomolecules 10, 1–13 (2020).
Esteva, A. et al. Dermatologist-level classification of skin cancer with deep neural networks. Nature 542, 115–118 (2017).
Tschandl, P. et al. Expert-level diagnosis of nonpigmented skin cancer by combined convolutional neural networks. JAMA Dermatol 155, 58–65 (2019).
Haenssle, H. A. et al. Man against Machine: Diagnostic performance of a deep learning convolutional neural network for dermoscopic melanoma recognition in comparison to 58 dermatologists. Ann. Oncol. 29, 1836–1842 (2018).
Brinker, T. J. et al. A convolutional neural network trained with dermoscopic images performed on par with 145 dermatologists in a clinical melanoma image classification task. Eur. J. Cancer 111, 148–154 (2019).
Li, C. X. et al. Diagnostic capacity of skin tumor artificial intelligence-assisted decision-making software in real-world clinical settings. Chin. Med. J. (Engl.) 133, 2020–2026 (2020).
Willingham, M. L. et al. The potential of using artificial intelligence to improve skin cancer diagnoses in Hawai’i’s multiethnic population. Melanoma Res. 31, 504–514 (2021).
Huang, K. et al. The classification of six common skin diseases based on xiangya-derm: Development of a chinese database for artificial intelligence. J. Med. Internet Res 23, e26025 (2021).
Han, S. S. et al. Evaluation of artificial intelligence-assisted diagnosis of skin neoplasms: a single-center, paralleled, unmasked, randomized controlled trial. J. Invest Dermatol 142, 2353–2362.e2 (2022).
Muñoz‐López, C. et al. Performance of a deep neural network in teledermatology: A single‐centre prospective diagnostic study. J. Eur. Acad. Dermatol. Venereol. 35, 546–553 (2021).
Han, S. S. et al. The degradation of performance of a state-of-the-art skin image classifier when applied to patient-driven internet search. Sci. Rep. 12, 16260 (2022).
Liu, X. et al. A comparison of deep learning performance against health-care professionals in detecting diseases from medical imaging: a systematic review and meta-analysis. Lancet Digit Health 1, e271–e297 (2019).
Haggenmuller, S. et al. Skin cancer classification via convolutional neural networks: Systematic review of studies involving human experts. Eur. J. Cancer 156, 202–216 (2021).
Takiddin, A., Schneider, J., Yang, Y., Abd-Alrazaq, A. & Househ, M. Artificial intelligence for skin cancer detection: Scoping review. J. Med. Internet Res. 23, e22934 (2021).
Brinker, T. J. et al. Skin cancer classification using convolutional neural networks: Systematic review. J. Med. Internet Res. 20, e11936 (2018).
Marka, A., Carter, J. B., Toto, E. & Hassanpour, S. Automated detection of nonmelanoma skin cancer using digital images: A systematic review. BMC Med. Imaging 19, 21 (2019).
Wu, K. et al. Characterizing the clinical adoption of medical AI devices through U.S. insurance claims. NEJM AI https://doi.org/10.1056/AIoa2300030 (2023).
Navarrete-Dechent, C. et al. Automated dermatological diagnosis: Hype or reality? J. Investig. Dermatol. 138, 2277–2279 (2018).
Navarrete-Dechent, C., Liopyris, K. & Marchetti, M. A. Multiclass artificial intelligence in dermatology: Progress but still room for improvement. J. Investig. Dermatol. 141, 1325–1328 (2021).
Adamson, A. S. & Smith, A. Machine learning and health care disparities in dermatology. JAMA Dermatol 154, 1247–1248 (2018).
Daneshjou, R., Smith, M. P., Sun, M. D., Rotemberg, V. & Zou, J. Lack of transparency and potential bias in artificial intelligence data sets and algorithms: A scoping review. JAMA Dermatol 157, 1362–1369 (2021).
Jobson, D., Mar, V. & Freckelton, I. Legal and ethical considerations of artificial intelligence in skin cancer diagnosis. Australas. J. Dermatol 63, e1–e5 (2022).
Daneshjou, R. et al. Checklist for evaluation of image-based artificial intelligence reports in dermatology: CLEAR derm consensus guidelines from the international skin imaging collaboration artificial intelligence working group. JAMA Dermatol 158, 90–96 (2022).
Tenenhaus, A. et al. Detection of melanoma from dermoscopic images of naevi acquired under uncontrolled conditions. Skin Res. Technol. 16, 85–97 (2010).
Brinker, T. et al. Deep learning outperformed 136 of 157 dermatologists in a head-to-head dermoscopic melanoma image classification task. Eur. J. Cancer 113, 47–54 (2019).