AI Demand Hits a Tipping Point
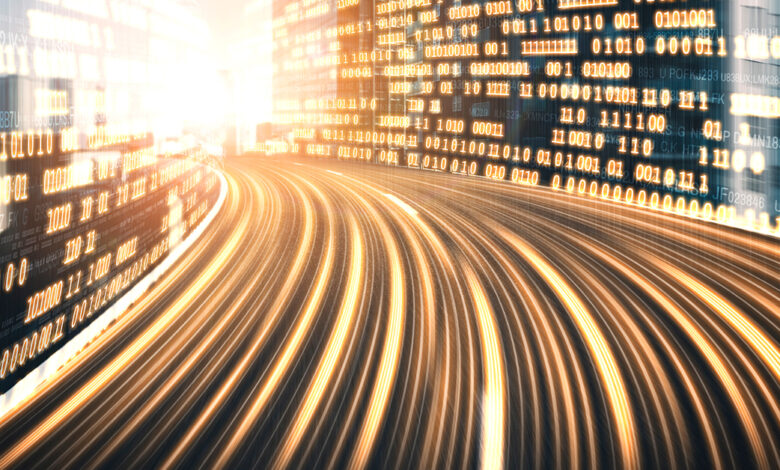
More than a year since generative artificial intelligence became the focal point of every tech company and investor, the pulse of conversation has moved from “platform shift” to meeting mass demand from companies and consumers looking to harness deep learning to be more efficient, productive and profitable.
A tipping point in AI demand was the overarching theme of Morgan Stanley’s Technology, Media & Telecom Conference in San Francisco—and over 1,600 industry investors and senior executives of more than 350 companies gathered—as businesses hasten to build more intelligent, fast, cost-effective and secure hardware and data platforms. One executive cited an estimated $3 trillion that may be spent on AI between 2023 and 2027, with generative AI spending composing 36% of that by 2027,1 highlighting the immense appetite as well as the opportunity for well-positioned companies and investors.
“Though cost savings and operational efficiencies remain a priority for large enterprises, they are also showing a willingness to spend, especially on generative AI and traditional AI hardware and software that may help reduce costs and increase productivity and revenue,” said Dave Chen, Head of Global Technology Investment Banking at Morgan Stanley.
Semiconductor Investing: Assessing Future Growth
Soaring demand for advanced AI chips have sent many semiconductor stocks into the stratosphere in the past year, meaning investors are particularity interested in understanding chip designers’ and makers’ runway for future growth. “Semiconductors are central to facilitating generative AI across industries, and companies are racing to bring next-generation products and end-to-end solutions to market, to address their customers’ proliferating needs around accelerated computing,” said Bill Frauenhofer, Head of West Coast Technology Investment Banking at Morgan Stanley.
Semiconductor companies cited the following potential growth factors for investors to consider:
- Demand from Data Centers: Chip designers are vying for market share for meeting the growing needs of enterprise data centers—warehouses of computers that store and process companies’ data. Fabless semiconductor companies are designing high-performing chips with different types of materials to meet the current computational needs of the market and support the next generation of demand, especially through graphics processing units (GPUs) that are in great demand because they facilitate the high-performance computing needed for generative AI workstreams that can produce multimodal content.
- Partnerships with Hyperscalers: Both chip designers and manufacturers shared that their alliances with large cloud service providers offer insights about enterprises’ generative AI use cases, which is helping semiconductor companies tailor solutions and drive revenue growth.
- Growing Interest from Sovereigns: While private-sector companies in health care, financial services, automotives, manufacturing and software have been some of the earliest adopters of accelerated computing, national governments are now showing growing interest in building computational infrastructure to harness generative AI to help with planning and decision making.
- Ramp-up in Inference Computing: The ability for trained generative AI models to make predictions based on live data, known as inference, takes significant computing power. Rising demand for these use cases should drive revenue growth for semiconductor companies, though supply will remain a challenge.
- Advanced Packaging for Edge Computing and Internet of Things (IoT): Advanced packaging, which aggregates wafer components to create high-performance system-on-chips, is helping meet the demand for semiconductors that accelerate AI and run applications on local edge devices, such as laptops, PCs, smartphones and medical devices, and IoT technologies such as autonomous or electric vehicles and robotics that connect to the cloud or data centers.
Indeed, as supply challenges remain a top question for investors, some chip fabricators shared that they are scaling their existing production facilities when possible and expanding in key geographies—especially in the U.S., Japan and Europe—to grow their wafer-manufacturing capacity. Many executives cited customers’ concerns around potential impacts to semiconductor supply from geopolitical tensions and export controls between the U.S. and China. Companies and investors are also assessing how local Chinese equipment vendors could be poised to gain market share.
AI Software Investing: Trends in Demand and Uses
With efficiency and productivity gains from AI a major area of investment interest, investors are also monitoring the landscape of software as a service (SaaS) companies that are offering traditional AI and generative AI products that automate workflows. “At the conference, software executives shared that their cost-conscious customers have appetite for AI products because they can see potential benefits for savings and productivity, but that they are eager to see a quick return on investment,” said Melissa Knox, Global Head of Software Investment Banking at Morgan Stanley.
Companies shared some of the biggest trends in AI software demand and some industry-specific verticalization:
- Automation in Customer Service: Executives pointed to AI powered chatbots as one of the most used and desired functionalities for enterprises to assist with work traditionally done by call center agents, store employees and field representatives. Generative AI’s advancements now enable chatbots to train on and produce multimodal content that includes images and videos, which is important for enterprises because much of their data is stored in PDFs, charts and graphs.
- Marketing AI: Some SaaS platforms are automating marketing across email, SMS text messaging and reviews that collect data, to help enterprises, as well as small- and medium-sized businesses, provide personalized customer journeys on desktop and mobile apps.
- Efficiencies in Financial Services: Firms such as banks are using generative AI to save them time and money on tasks including language translation, written summaries of research and sentiment scoring for earnings transcripts.
- Copilots for Data Insights and Engineering: Text-to-SQL and text-to-code generative AI software is helping enterprises democratize business intelligence and computer programming across their workforces, streamlining the need for technical expertise.
- Semiautonomous Workflow Agents: AI-powered copilots are helping organizations retrieve information and perform tasks in sophisticated ways across commonly used applications such as emails, calendars and meetings. One example: If an employee misses a meeting, generative AI can help summarize and propose action items.
Big Data Companies: Consolidated Offerings and AI Products
Big data is at the heart of generative AI’s promise, which gives big data companies even more sway in shaping how enterprises manage, store and deploy their vast troves of information. As the demand for solutions continues to grow, some are building multiple data tools as platforms vs. siloed specialty offerings (the so-called best-of-breed approach). “Investors are trying to determine which big data companies will gain and lose market share, as some customers may want to pare down their software and double down on a few data partners because of potential cost and security benefits,” said Brittany Skoda, Global Head of Software Investment Banking at Morgan Stanley. “While best-of-breeds could move faster and be more specialized, customers are also considering how unified platforms can help them reduce cloud usage and heighten visibility and control of security protocols.”
In addition to consolidated vs. best-of-breed offerings, big data companies shared additional trends in the solutions they are building for enterprise clients:
- Labeling Data: Before data can be useful to AI models, it must be labeled to add context or meaning. Companies cited opportunities resulting from large amounts of raw, unlabeled data held by corporates.
- Real-time Data Streaming: Big data companies are helping process customer data from various sources together in real time with large language models (LLMs) to improve customer service and drive employee productivity.
- Improving Accuracy: Enterprises care about whether LLMs can update with real-time information and avoid hallucinations, and they are interested in solutions that help fine tune their advanced machine learning systems to improve trustworthiness and scalability.
- Synthetic Data in Generative AI: Big data companies are helping customers build generative AI algorithms that can create synthetic data, or artificially-manufactured data made based on real-world data. Synthetic data is useful to generate large training datasets without manual labeling and for the ability to sidestep restrictions on regulated or sensitive data.