AI is bridging the gap between physical and digital engineering
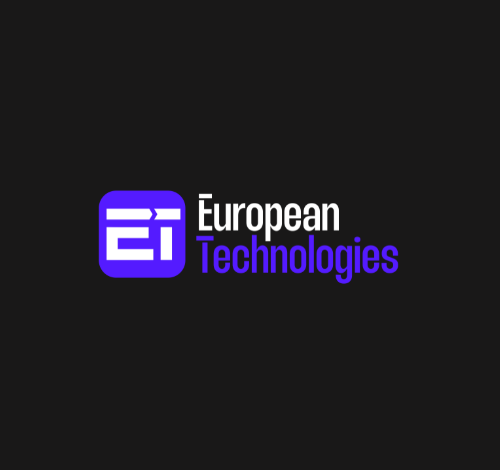
By Keith Perrin, Dr. Kambiz Kayvansash and Dr. Guillaume Boisot, Hexagon’s Manufacturing Intelligence division
In the ever-evolving landscape of technology, the convergence of physical and digital realms is no longer a distant vision—it’s a reality unfolding before our eyes. Nowhere is this more evident than in the field of Computer-Aided Engineering (CAE). As we stand at the precipice of this transformation, let’s explore the implications and possibilities in this future.
Current practices in CAE stand at the forefront of this convergence, because high-quality physics-based simulations can accurately represent the tangible world of physical prototypes with the limitless possibilities of digital simulations. The cloud democratizes access to simulation, enabling engineers and designers to harness immense computational power and without the constraints of on-premises infrastructure and to aggregate and process real-world data when needed.
Digital twins, the offspring of this union, bridge the gap between the virtual and the tangible. Imagine a world where geometric variability, thermal fluctuations, vibrations, and noise are seamlessly integrated into simulations. Digital Models that know what they are and how they’ll react in real world environments. Ready to influence design. Readily available to trade against each other.
And machine learning and AI were the missing technologies that are now making it possible.
Multi-physics simulation comes to life
Easier, more accessible, and increasingly linked simulations will enable more multi-physics modelling and deliver a more holistic understanding of performance before something is real. However, unlike today, where linking simulations can be complex and difficult, AI/ML present new capabilities to bring together different performance insights. The march towards more readily accessible and usable reduced order models (ROMs) that summarise different system properties, that can be linked together effectively, should also enable solution independence and more flexibility and adaptability.
Increasingly, physical testing, (CAE), and Artificial Intelligence (AI) are applied together to make digital twins a reality. AI algorithms can learn from physical tests and simulations as a combined set of inputs, delivering increased overall accuracy and confidence in optimized designs & processes.
This mixture of real and digital data provides an intriguing challenge. Consider a simple loading constraint in a conventional physics-based CAE tool. In many tools this load is a finite, simple, number. In the real world a load is usually anything but simple, and more of a dynamic variable. Wouldn’t it be great if we could bring that real world load variation into our simulation? With reality capture and AI/ML we can. All of this is now possible. Specifically though, what else can we consider looking forward?
The “no right answer” paradigm
As every quality engineer will tell you, performance, reliability and robustness thrive within a bounded box of answers, not a single point answer. There’s no single solution, but a spectrum of possibilities tailored to specific contexts. Quality experts like to talk about the idea of accuracy vs precision when it comes to the real world effects of variation in manufacturing processes and real world conditions. It’s time that we come to accept that CAE is not about a singular answer, but a measure of uncertainty – be that understanding the tolerances required, the risk or safety margins of performance.
Consider this, when thinking about the real-world load example mentioned above.
AI enables holistic optimisation
It’s well known that AI can assist in trade-off of engineering decisions by leveraging advanced algorithms to analyse multiple design variables and constraints simultaneously. It can quickly evaluate numerous potential solutions, identifying optimal trade-offs between competing objectives such as cost, performance, and sustainability. By providing data-driven insights and predictive analytics, AI enables engineers to make informed decisions that balance various factors, ultimately leading to more efficient and effective design outcomes.
Operationalising digital twins
Live, predictive intelligence embedded in systems and equipment—already seen in automotive driver assistance or robotics —should become increasingly more commonplace. By summarising the learnt response of complex systems into simpler executable models, we can increasingly run them in real time to predict equipment performance and maintenance based on their usage and environment. For example, we use digital twins to help industrial equipment operators predictively schedule costly maintenance for offshore wind turbines or agricultural machinery, based on their performance and need for maintenance.
As a design engineer, gathering more and higher fidelity information back to our (design) simulation models there are of course valuable opportunities to improve elements of the design.
Off-the shelf digital twins
The internet is already full of CAD repositories, increasingly coupling supply chains together via exchange models. If suppliers provide a behavioural model of their component or system to the market that captures all the key performance characteristics, then a customer has more, and better, information with which to evaluate their suitability within their system design.
Hexagon pioneered this for composite behaviour modelling working with material suppliers such as Solvay and Sumika, making it possible to evaluate new low-carbon alternatives within new or existing structural analyses or crash tests.
At the component level, designing digitally using off-the-shelf “digital components” is already becoming a reality in the Electronic Design Automation (EDA) world, in which online catalogues of behavioural models and processes can be downloaded and plugged into wider systems. It’s easy to see how the sort of co-engineering and collaboration that we might only see after a rigorous legal agreement is signed today can become easier for all parties integrating electro-mechanical systems. Imagine routinely purchasing digital models—tyres, airbags, bushings—alongside the physical goods. By extension, these could form part of a digital passport of that serial component capturing aspects of its authenticity, traceability, or sustainability digital thread.
Making digital twins deliver with AI
At Hexagon we feel we’re in a unique position, pioneering many new applications that bring together the physical and virtual worlds with cutting-edge technologies including AI that help to transform those data into actionable insights.
If you’d like to see more about what we’re doing to make both complex physics-based simulations and digital twins feasible with AI and machine learning, and approaches you can adopt please join our resident experts as they explore these multi-faceted topics with advice and real-world applications in our free bite-sized webinar series.