AI-Powered Cybersecurity: How Machine Learning is Revolutionizing Threat Detection
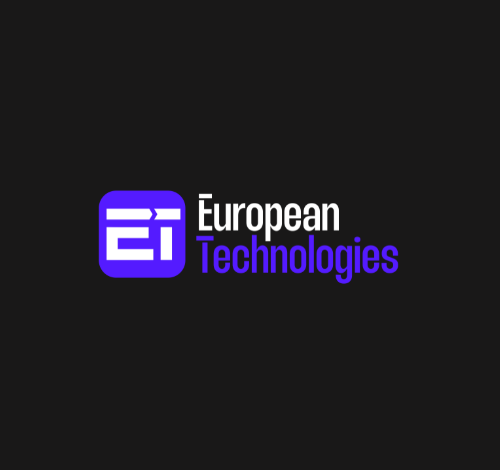
Traditional cybersecurity methods are falling short as cyber threats continue to evolve. Artificial intelligence (AI) and machine learning (ML) are revolutionizing threat detection through automated analysis of vast data sets, real-time identification of anomalies, and predictive capabilities to preempt attacks.
Introduction
The digital world is evolving rapidly, and cyber threats are escalating in sophistication and volume. Traditional cybersecurity measures are increasingly ineffective against advanced tactics like social engineering, zero-day exploits, and advanced persistent threats (APTs). Organizations must adopt more advanced threat detection capabilities to identify and neutralize threats before they cause harm.
Artificial Intelligence (AI) and Machine Learning (ML) offer powerful tools for enhancing cybersecurity efforts by automating threat detection and response processes. AI and ML algorithms can sift through vast amounts of data at incredible speeds, identifying patterns and anomalies that may indicate a cyberattack, enabling immediate identification and neutralization of threats.
Limitations of Traditional Threat Detection Methods
Signature-based and rule-based detection methods, which match known malware signatures or specific behavior patterns, have significant limitations. They struggle to keep up with evolving and unknown threats, such as zero-day exploits and polymorphic malware. Additionally, they have high false positive rates and require regular updates to remain effective, creating vulnerabilities.
The Evolution of Machine Learning in Cybersecurity
Machine learning enables systems to learn and improve from experience, without explicit programming. In cybersecurity, ML applications range from anomaly detection and network traffic analysis to automatic rule generation for intrusion detection systems. ML algorithms excel at processing vast datasets, identifying patterns and correlations that signal potential threats, with a level of precision and speed unattainable by traditional methods.
Applications of Machine Learning in Threat Detection
1. Malware Detection: ML algorithms analyze code behavior to detect anomalies that could indicate new or modified malware, improving detection of zero-day threats.
2. Network Traffic Analysis: By monitoring data flow, ML algorithms can quickly spot deviations from normal traffic behavior, such as increased activity in unusual ports or excessive data transfers, enabling earlier detection of potential security breaches, such as DDoS attacks or unauthorized data exfiltration.
3. User Behavior Analytics: ML algorithms can learn normal user behavior patterns and alert security teams to anomalous activities that may indicate a security breach. For instance, if a user suddenly accesses a large volume of sensitive data or logs in from a geographical location that deviates from their normal pattern, the system can flag these actions for further investigation. This aspect of machine learning helps in detecting insider threats and compromised user credentials, enhancing the overall security posture of an organization.
4. Predictive Analytics: By analyzing past incidents and current trends, ML algorithms can predict potential future attacks, allowing organizations to proactively strengthen their defenses against predicted threats. This forward-looking approach helps in preemptively addressing vulnerabilities and reducing the likelihood of successful cyberattacks.
Benefits of AI-Powered Threat Detection
- Enhanced Threat Detection Capabilities with improved accuracy and speed
- Improved Data Security Measures by identifying and mitigating threats more effectively
- Real-Time Response to Cyber Threats minimizing potential damage
- Scalability and Adaptability to evolving cyber threats
- Augmented Security Teams by automating routine tasks and providing actionable insights
Challenges and Limitations
- Data Quality and Availability: Machine learning models rely on high-quality and extensive datasets for accurate threat detection.
- Interpretability and Transparency: The “black box” nature of many AI algorithms makes it difficult for security professionals to understand the rationale behind threat detection.
- Integration and Deployment: Implementing and integrating AI into existing cybersecurity infrastructure can be challenging and resource-intensive.
- Vulnerabilities in AI Algorithms: Adversaries can use techniques like adversarial machine learning to deceive AI systems.
- Overcoming Bias in Machine Learning: Ensuring that training data is unbiased and representative is crucial for fair and effective AI-driven cybersecurity measures.
Conclusion
AI and machine learning are revolutionizing cybersecurity by enhancing threat detection and response times, continuously evolving to counter new threats, and driving innovation in creating more secure digital environments. As cyber threats become more sophisticated, the role of AI in cybersecurity will continue to expand, highlighting the importance of embracing these technologies to safeguard digital assets and maintain data security.