Artificial Intelligence Model Successfully Assesses T2D Risk
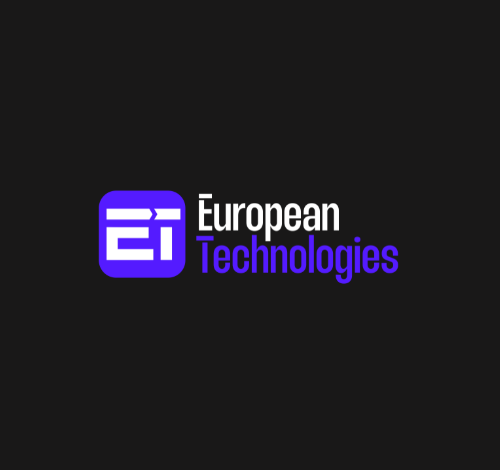
TOPLINE:
An artificial intelligence–based model that incorporates polygenic and multi-image risk scores with key demographic variables effectively identifies individuals at a high risk for type 2 diabetes (T2D), suggests a Taiwanese study.
METHODOLOGY:
- Early detection and risk assessment of T2D are critical for effective health management, as diabetes imposes a significant mortality and economic burden on patients.
- Researchers used an artificial intelligence machine learning approach (eXtreme Gradient Boosting) to design various risk assessment models for T2D by integrating genome-wide single-nucleotide polymorphisms, multimodality imaging data, and demographic information from 68,911 participants from the Taiwan Biobank.
- Taiwan Biobank collected baseline and follow-up questionnaires; blood and urine samples; biomarker measurements; and medical imaging data, including abdominal ultrasonography (ABD), carotid artery ultrasonography (CAU), bone mineral density (BMD), electrocardiography, and thyroid ultrasonography.
- In the genetic-centric analysis, 50,984 participants were included, 2531 of whom were self-reported patients with T2D and 48,453 were self-reported control individuals without T2D.
- In the genetic imaging integrative analysis, 17,785 participants whose genetic and medical imaging data were available were analyzed, 1366 of whom were self-reported patients with T2D and 16,419 were self-reported control individuals without T2D.
TAKEAWAY:
- The model that used polygenic risk scores (PRS) along with demographic variables such as age, sex, and family history of T2D showed a good accuracy in predicting the risk for T2D, with an area under the receiver operating curve (AUC) of 0.915.
- Integrating the image features with genetic information and demographic factors further increased the AUC to 0.949.
- A simplified version incorporating only eight key variables (family history, age, fatty liver from the ABD image, spine thickness from the BMD image, PRS, end-diastolic velocities in the right and left common carotid arteries from the CAU images, and RR interval from the ECG images) showed an AUC of 0.939.
- Lastly, the performance of this model was validated in a second independent dataset, which yielded an AUC of 0.905.
IN PRACTICE:
“We successfully developed artificial intelligence models that effectively combined genetic markers, medical imaging features, and demographic variables for early detection and risk assessment of T2D,” the authors commented.
SOURCE:
Yi-Jia Huang, Institute of Public Health, National Yang-Ming Chiao-Tung University, Taipei, Taiwan, led this study, which was published online in Nature Communications.
LIMITATIONS:
The model needs to be validated in external cohorts for improved generalizability. Owing to the limited follow-up time of this study, only a few participants reported a change in their T2D status from baseline to follow-up.
DISCLOSURES:
This work was supported by research grants from Academia Sinica. The authors declared no conflicts of interests.