Best Practices for AI and Data Integration: CRE Leaders Weigh In
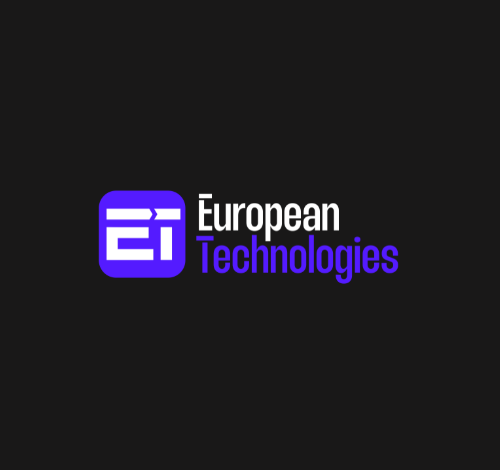
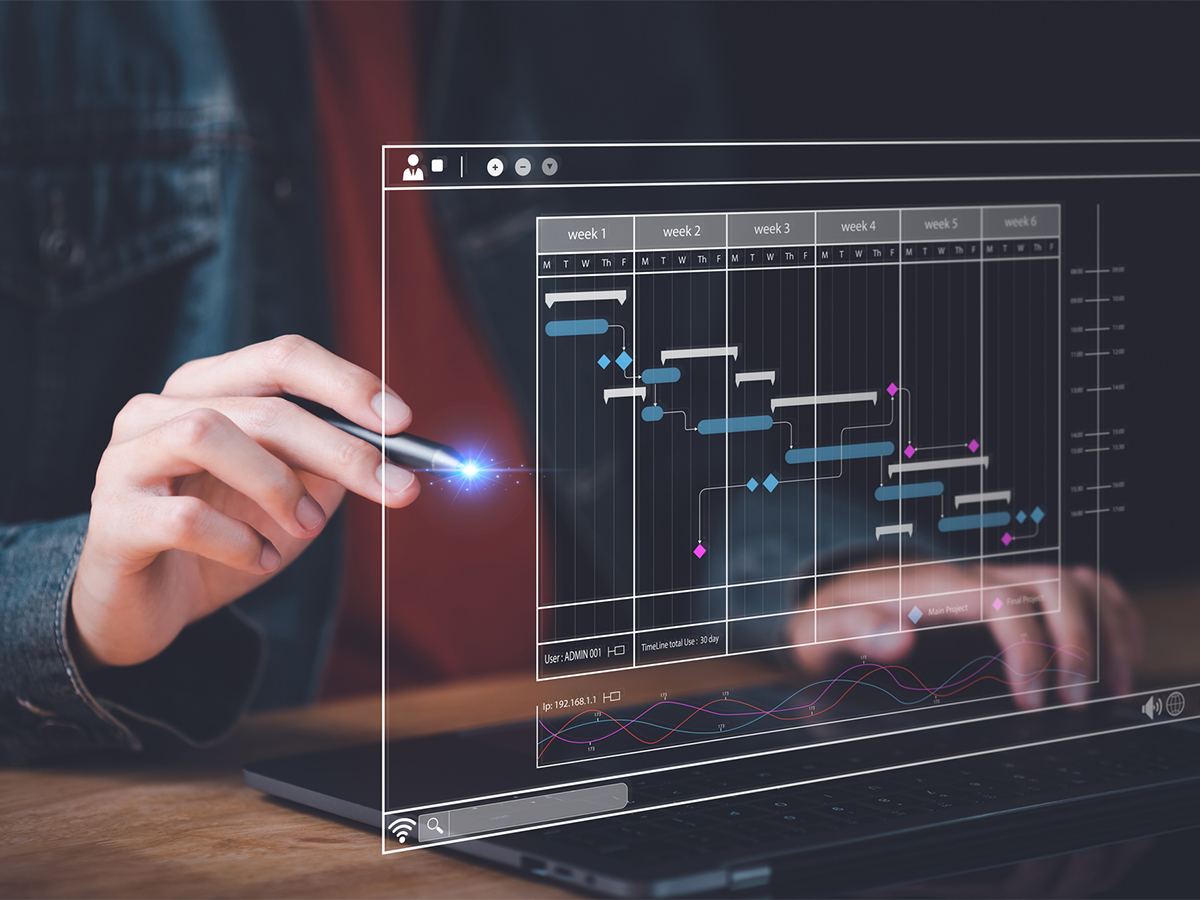
Excel was the be-all and end-all for commercial real estate firms’ analysts when making decisions to buy or sell, and when and where.
But the onset of artificial intelligence (AI), especially in the past few years, has many leading companies of all sizes rethinking and restructuring their research data analytics departments to meet technology’s deeper and more efficient way of measuring data.
It’s not just hiring data scientists, but finding those with advanced skills in problem-solving, those who respectably understand all aspects of commercial real estate—from acquisitions to operations to market analysis.
On top of that, companies are seeking employees whose skills include expertise with computer usage, hands-on knowledge of one or more programming languages and familiarity with building and customizing systems and automation possibilities.
By finding those with the optimal combination—that also includes creativity and agility—firms can thrive in this technological, innovation acceleration climate, according to a panel of CRE research and strategy professionals speaking on a recent podcast hosted by Lisa Flicker, head of real estate at Jackson Lucas. Other speakers included Sabina Reeves, head of insights & intelligence, chief economist, CBRE Investment Management; Umar Riaz, North America hospitality and leisure segment lead, Ernst & Young; Sabrina Unger, managing director, research & strategy, American Realty Advisors; and Erin Patterson, managing director & global co-head of research & strategy, real estate, Manulife Investment Management.
“When we think about managing change and human capital, we are always considering how to successfully integrate individuals with diverse skill sets and ensure that existing teams are upskilling to meet them at a high level,” Flicker said. “Recently, we have found that seamlessly weaving tech-savvy quants into existing research teams has become an art. Our most successful placements occur when leadership has developed deep trust internally.”
Riaz said that first, companies must get their data infrastructure right.
READ ALSO: Setting the Bar for Data Center Infrastructure
“In real estate, there are a lot of data that resides in different systems and the connective tissue is Excel, and Excel is not a very long-term strategy for having a data platform,” he said.
Reeves, who is based in London, has forbidden the use of Excel on her team for a while.
She said hiring computer programmers—those who can code—is now table stakes.
“You want people who are annalistic and who think in a ‘quacky,’ interesting kind of way,” Reeves said. “You can’t future-proof by hiring for what’s useful in this moment, it needs to be for what could come in this world of incredibly rapid change.”
Unger emphasized that companies must figure out how to take raw output and data and translate it into something acceptable for the real estate staff and leadership.
Making this difficult, she said, is that employees who have been at a company for 20 or 30 years, and who aren’t used to using the tools of today, can become reluctant to use it when making deals.
“They don’t want to forego that decision power they have,” Unger said. “There will be hesitation and it creates friction.”
When hiring a data scientist for the first time, the panel said, it’s about developing trust with them, pulling the curtain back on the process, having transparency and showing conviction when acting on what comes from the data model.
Companies need a person who can bring the data to life in a less technical way. “Don’t just stick them in the corner and say I don’t know what they’re doing,” the panel advised.
In some cases, the head of research can become the champion for the data scientist.
“Integrating acquisitions and data science leaders into a cohesive team sometimes requires finding the right executive to serve as a ‘bridge’ or intermediary, effectively translating needs and abilities to achieve the desired outcome,” Flicker said. “Some companies that possess both senior data leadership talent and senior acquisitions talent have successfully created a bridge between the two.”
Thinking Long-Term
Many jobs related to AI didn’t exist even a year ago, and this will continue because things will pop up along the way that we can’t anticipate today, the panel said.
“We have been using data scientists and quants for a while, but their skills have changed along the way,” Reeves said. Her department at CBRE includes 60 team members. “They have to be able to code, but you also have to upskill your current teams. From the CIO to the junior hires, they all have to have a connection to how we’re putting data out and what our processes are.”
The latest AI tools, including generative AI, “are unleashing creativity for those who are literally prisoners to excel and to the low-value repetitive work,” Reeves continued.
“These tools not only free up your time, but they also free up possibilities,” she said. “Because now, rather than your preconceived notions of what three or four data variables might have driven as outcomes, you can run try to run factor analysis over 1,000 or even over 10,000. It’s quite liberating.”
Where to Start Digging
The panel said AI shouldn’t be looked at solely as a research and strategy tool but as a corporate strategy.
For companies first building a data analysis department, Riaz said they must start by asking what the data will be used for, what will it drive and what will it solve.
“The value is not going to come from the data you have but the questions that you ask,” he explained. “You have to ask creative questions.”
Reeves quipped a recent expression told to those who are first curious about AI’s capabilities, “It is not data in and miracles out.”
According to Riaz, what is most compelling about CRE firms using AI today is how these new tools drive active management decisions such as how to maximize rent, how to maximize occupancy and how to do due diligence on prospective tenants more efficiently.
“Asset management decisions should be made quantitatively as much as possible, based on advanced data analytics that are available either on the buy side or sell side,” he said.
Firms want to use generative AI to make investment decisions, but they find that setting those algorithms is very complex, Riaz said, because their volume of data is much greater than before.
He said internal and external data is needed “because you’re trying to project business and consumer trends and then transfer those into real estate trends.”
Big Company, Little Company
Many companies subscribe to the same third-party data sets, so that’s no longer a differentiator.
“The proprietary information from your portfolio will be the differentiator,” Riaz said. “Unequivocally you should not be sharing your own company data in public.
The advantages of scale do not exclusively apply to larger companies regarding managing and using data.
Riaz explained that big companies have a much more difficult task ahead than smaller companies because the more complex these larger companies’ comps are, the more disaggregated the current state of data will be.
“If you’re a smaller company, data is more defined,” he said. “You don’t have as many silos and the good thing is that the investment required to create these capabilities is quite achievable now.”
Unlike 10 years ago, there’s a significant investment in cloud-based tools that can be used—it’s not an insignificant investment. Still, even for smaller real estate companies, it’s highly accessible, he said. Large, more sophisticated organizations might also face a disadvantage in overall change management for their employees.