Bryant data scientist uses AI to address human trafficking and homelessness
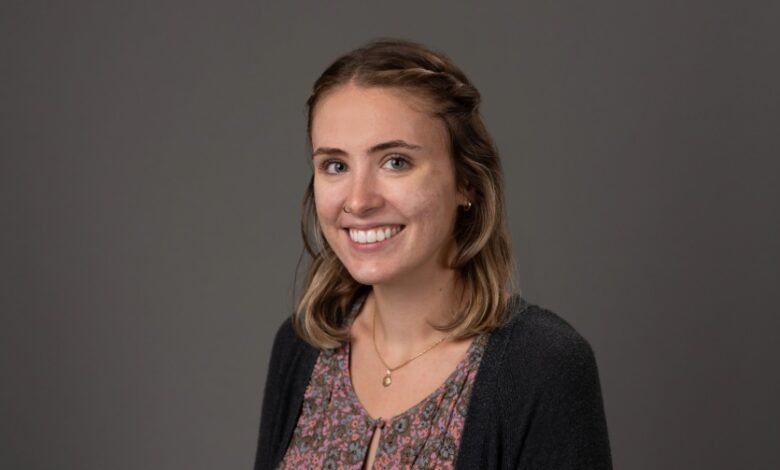
If Geri Louise Dimas, Ph.D., has her way, her research will one day be obsolete. An assistant professor of Information Systems and Analytics and co-director and fellow at the Stopping Trafficking and Modern-Day Slavery Project (STAMP) Research Lab at the Institute for the Quantitative Study of Inclusion, Diversity, and Equity (QSIDE), uses data to better understand and improve processes that involve vulnerable populations such as those experiencing homelessness, human trafficking, and forced migration.
“My dream is that my research becomes irrelevant someday because we no longer have a need to study these problems,” she says. “But, until that’s the case, it’s my job to help understand them.”
Below, Dimas discusses her work, what drives her, and how she hopes to help data science students discover their own passions.
What drew you to data science?
“When I was considering Ph.D. programs, I realized that my dream would be to use what I was learning to help vulnerable people, especially those affected by issues like human trafficking.
That was my long-term career goal, but I was really fortunate that my advisor for my Ph.D. had been on a team that just received a National Science Foundation (NSF) grant for an anti-human trafficking project and they were looking for a new data science student. I was really fortunate to be able do this right off the bat, to be able to look at these hard problems, and see how I can use math, stats, and other applied analytical methods to try to help improve these situations.”
Can you share a bit about one of the projects you’ve worked on?
“In New York City, we’ve focused on the problem of human trafficking, a crime that preys upon vulnerable people and that happens a lot more often in the United States than people realize.
Part of our focus has been understanding what puts people at risk. For example, runaway and homeless youth are known to be at risk for experiencing human trafficking or other forms of exploitation because of their age, lack of support or community, and lack of physical needs, such as housing, clothing, money, and food.
Our work in New York City, which was also funded by the National Science Foundation (NSF), allowed us to collaborate with a social scientist at New York University to investigate meeting their needs. For example, we looked at the current services specifically available to runaway and homeless youth and aimed to truly understanding their actual wants and needs.
“You don’t just have to know the data science tools available — you have to understand which are the correct ones for the job.”
Giving agency to the runaway and homeless youth population is a really important aspect of this project, so we designed a survey to ascertain their needs and deployed it in New York City. We also worked with different partners in the city to better understand how this data can be matched with the current supply of support resources that are available.
In Feb 2024, we presented to stakeholders including providers and city and state employees, and we are now finishing up a white paper on our survey findings to come out in the next month or so.”
How will the collected survey data be used to help people?
“We’ve created a large-scale mathematical optimization model that assists with finding these gaps that exist. Then, we can provide that information to decision makers in New York City to help them understand the current state of things and how to put in place the necessary additional support.
A second project that uses the survey data is a benefit-to-cost ratio analysis that looks at building out these additional services or shelter locations in the most effective locations. The framework would allow for decision makers to look through different scenarios to try to figure out what is the best possible plan going forward for capacity expansion.
A third project I’m currently working on involves the analysis of the survey data, looking at youth profiles, and trying to understand the difference in needs. One of the main research questions we’re interested in is if there are any differences in needs for those who have experienced, or are at a higher risk of experiencing, human trafficking.”
This is high-level data science analysis, but there’s also an intensely human element. Tell us about some of the skills you need to do this work.
“You don’t just have to know the data science tools available — you have to understand which are the correct ones for the job. I think of myself as an ‘applied data scientist’ in that I focus on the problem at hand and then pull from my knowledge base and think: ‘What kind of applications might be able to help with this?’
I always say, ‘numbers don’t lie, people do,’ because data is only as good as our understanding of it and the story it tells. I think it’s rare that people purposefully intend to use data in a misleading way, but if we’re not extra careful, it can happen in unintended ways very easily.
“I always love when I tell students about some of the research I work on and they tell me ‘Oh, I didn’t realize you can use this in that way.’ ”
It’s said that about 80 percent of the data scientist’s process is really cleaning, processing and making sense of the data.
And then, of course, you need to be able to adapt your model as needed. Oftentimes we’ll talk through the problems we’re considering and the solutions that we suggest with stakeholders, and they’ll note ‘Well, here’s something from our domain which might impact that.’ Then we go back to the drawing board or reconsider how we can make adjustments that take that feedback into consideration.”
Your work involves working closely with community organizations. Why is that so important?
“Coming together across disciplines is important because working in a silo is not sufficient to solve these complicated problems. That means not just taking a multidisciplinary approach, but a transdisciplinary approach as well.
I think one important thing data scientists always need to keep in mind is to avoid approaching problems with a hero complex, this idea that: ‘We’re going to come in and save the day.’ Instead, it’s about wanting to support partnering organizations and the great work they’re already doing.
So much depends on the context and situation. That’s why having conversations with stakeholders, hearing their concerns, and truly taking them into consideration is so important because they’re the ones who are actually going to be applying our work. It also gives us a chance to reevaluate our methods when they provide new information.”
You joined the Bryant faculty this fall; what do you enjoy about teaching?
“I love getting people excited about data science and I want to help them find the love that I’ve been able to find for data and apply it to their passion, whatever it is.
As someone who struggled with dyslexia in school, I want to be able to find ways to support students individually and discover their strengths and who they are. I also want them to see that they can use data science in so many different ways. I always love when I tell students about some of the research I work on and they tell me ‘Oh, I didn’t realize you can use this in that way.’ ”