Businesses Grapple With AI Hallucinations
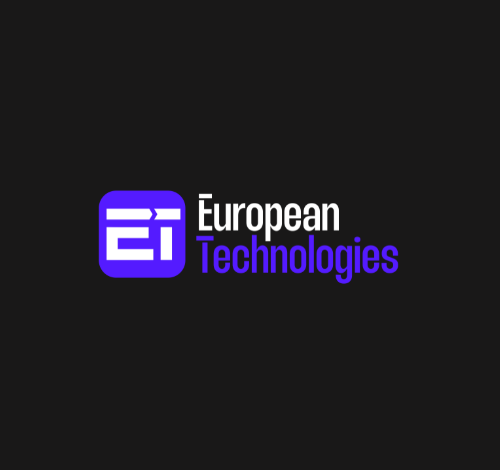
In the new world of artificial intelligence (AI)-powered automation, businesses face a vexing problem: AI systems that confidently generate plausible but inaccurate information, a phenomenon known as “hallucinations.”
As companies increasingly rely on AI to drive decision-making, the risks posed by these fabricated outputs are coming into sharp focus. At the heart of the issue are large language models (LLMs), the AI systems that power many of the newest tech tools businesses are adopting.
“LLMs are built to predict the most likely next word,” Kelwin Fernandes, CEO of NILG.AI, a company specializing in AI solutions, told PYMNTS. “They aren’t answering based on factual reasoning or understanding but on probabilistic terms regarding the most likely sequence of words.”
Confident but Wrong
This reliance on probability means that if the training data used to build the AI is flawed or the system misinterprets a query’s intent, it can generate a response that is confidently delivered but fundamentally inaccurate — a hallucination.
“While the technology is evolving rapidly, there still exists a chance a categorically wrong outcome gets presented,” Tsung-Hsien Wen, CTO at PolyAI, told PYMNTS.
For businesses, the consequences of acting on hallucinated information can be severe. Inaccurate outputs can lead to flawed decisions, financial losses and damage to a company’s reputation. There are also thorny questions around accountability when AI systems are involved. “If you remove a human from a process or if the human places its responsibility on the AI, who is going to be accountable or liable for the mistakes?” asked Fernandes.
Strategies for Mitigation
As the AI industry grapples with the challenge of hallucinations, a range of strategies are emerging to try to mitigate the risks. One approach is retrieval augmented generation (RAG), where AIs are fed curated, factual information to reduce the likelihood of inaccurate outputs. “With fine-tuning and safeguard frameworks in place, it helps to drastically limit the likelihood of a hallucinated response,” said Wen.
Roman Eloshvili, founder and CEO of XData Group, highlighted the role of Explainable AI (xAI) technologies in mitigating hallucination risks. Eloshvili emphasized that methods like Local Interpretable Model-agnostic Explanations (LIME) and Shapley Additive exPlanations (SHAP) can be instrumental in identifying and correcting hallucinations early on.
“LIME clarifies the prediction system and can help us better understand how AI models arrive at their outputs, allowing potential hallucinations to be identified,” he told PYMNTS. “SHAP, on the other hand, explains each feature’s influence on the model’s predictions and assigns importance to different features the model uses to make a prediction. By analyzing the importance of these features, users can identify features that might contribute to hallucinations and adjust the model accordingly.”
Other techniques, like self-critique and chain-of-thought methods that prompt AIs to rethink and explain their answers, can also help reduce inaccuracies.
Despite these technological advancements, Eloshvili underscored the enduring necessity of human oversight, especially in critical sectors like banking anti-money laundering (AML) compliance. “Nowadays, in many cases — for instance, in banking AML compliance — both human moderation and explainability of the AI tools are considered mandatory,” he said.
Experts caution that eliminating hallucinations may not be possible without sacrificing the creative capabilities that make LLMs so powerful.
“To err isn’t just human,” said Wen. “Just as you can’t guarantee 100% efficacy from the human brain, even the most sophisticated language models operating on near-perfect neural network designs will make a mistake on occasion.”
Navigating the challenges posed by AI hallucinations will require a multifaceted approach for businesses. Maintaining human oversight, implementing fallback processes and being transparent about AI’s use and limitations will all be critical. As technology evolves, finding the right balance between harnessing AI’s power and mitigating its risks will be an ongoing challenge for companies across industries.