California’s proposed AI measures will only add to a bloated bureaucracy – Orange County Register
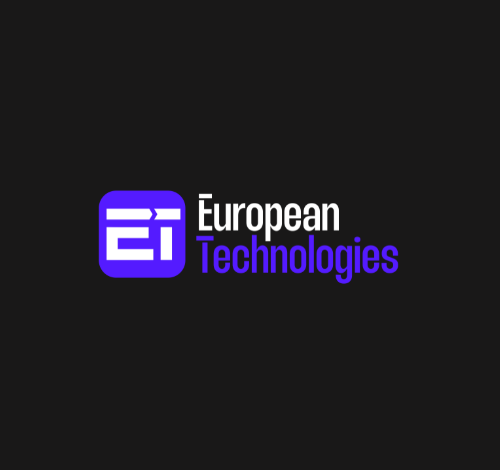
Newly-elected legislators stand to be sworn in in the Senate chambers during the opening of the Legislature in Sacramento, Calif., on Monday Dec. 5, 2022. California lawmakers briefly returned to the State Capitol to swear in new members and elect leaders for the 2023 legislative session. (Martin do Nascimento/CalMatters via AP, Pool)
This year, California has ramped up its efforts to regulate artificial intelligence, introducing dozens of proposals addressing many controversial aspects of AI. Artificial intelligence has brought with it concerns about privacy, bias, nonconsensual pornography generation, and once we begin to integrate AI into critical systems like our defense architecture, the possibility of errant AI causing catastrophic events.
Given that this technology is already in use in many industries and is widely available to the masses, these problems can’t wait and California is rightfully attempting to address them.
With that said, hasty and poorly thought out regulations also produce their own set of undesirable consequences.
In May, the California Civil Rights Department proposed new regulations that would affirm that it is illegal for employers to use AI for decision-making, such as hiring, that discriminates against people based on their being members of a protected group.
Oversight on use of AI in hiring would include internal bias reviews for neural networks and it would require institutions to reveal the data sets they use to train their AI.
No one is out there purposefully making biased neural networks of course. Depending on the type of AI, they may be trained on a data set composed of desirable characteristics or application markers, none of which include race, gender, or anything of the like.
Data sets are responsible for much of the bias that ends up manifesting in deep neural networks but it can be difficult to identify and remedy. One real-world example of this was in Amazon’s hiring algorithm, which discriminated against women because it favored certain terms on applications that were mostly used by men, such as “executed”.
Discrimination in AI is often detected by identifying pre-existing bias in the data set as an over or underrepresentation of a group in the sample, or by analyzing the network’s outputs for outcomes that are persistently unfavorable to certain groups.
Sensible AI regulations should take into account the subtle ways in which these metrics can mislead. In instances where there is an underrepresentation of a group in a data set, this may often be explained by a greater availability of data for a particular group. For example, there may be more data about males in engineering simply because there are more males in engineering. Is this a problematic form of bias?
To oversimplify, perhaps this could be remedied by cutting out some of the male sample to feed the neural network with a more representative data set. This could work in something like hiring algorithms but consider how this could impact healthcare AI. If a medical chatbot’s function is to recommend treatments for patients and it has a higher success rate for white people, this would be seen as a bias in the system given our methods for detecting them.
If the bias is produced because there is more healthcare data available for white people, then clearly, making the data set more representative would only result in the chatbot lowering its success rate for white people to match that of black people.
An over or underrepresentation of a group in the network’s outputs also doesn’t tell you whether the bias is on the basis of being a member of a protected group or if it is caused by any number of morally innocuous reasons.
New proposals for regulation don’t seem to be taking into account the distinction between problematic and non-problematic bias. If there is some sort of over or underrepresentation, it would be taken to be problematic and therefore developers and companies would be subject to penalties.
Deepfakes are also being targeted for regulation with Senate Bill 926. The bill would make it illegal to create and distribute AI-generated, sexually explicit images while representing them as authentic. Setting aside how this may conflict with our freedom of expression and the fact that these generated images aren’t actually explicit photos of the victim, this doesn’t appear to be enforceable.
Open-source AI is already widely available and there is no way to stop its spread. The world has struggled to enact legislation that limits revenge porn even though the culprit will often have direct ties to the victim. In the case of deepfakes, the creator will often have no ties at all to the victim. It’s simply too easy to post anonymously in a way that would make it impossible to track.
Stopping harmful deepfakes, if this is indeed what we should do, would take a monumental effort involving developers, law-enforcement, and open-source software hosts like Github. They won’t be going away any time soon irrespective of however many regulations California adopts.
None of this is to say that we shouldn’t regulate artificial intelligence or that we have no resources to address concerns like bias in hiring. I’m merely suggesting that California adopt AI legislation that takes into account some of the subtleties that go into the misuse of AI and refrain from taking the easy, knee-jerk reaction of simply banning things.
Rafael Perez is a doctoral candidate in philosophy at the University of Rochester. You can reach him at rafaelperezocregister@gmail.com.