Can AI Contribute to Health Misinformation?
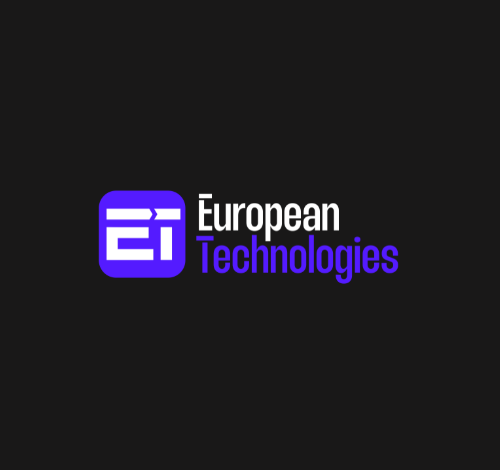
Large language models (LLMs) are a type of artificial intelligence (AI) program capable of recognizing and generating text. They are expected to play a significant role in healthcare areas such as remote patient monitoring, triage, health education, and administrative tasks.
LLMs, however, can also be used for the massive generation of health misinformation, leading to consequences such as stigmatization, rejection of proven treatments, confusion, or fear. This possibility is particularly concerning, because more than 70% of patients use the Internet as their primary source of health information, and it has been shown that false information spreads online six times faster than factual content.
Two Contemporary Examples
To assess the effectiveness of protective measures against the use of LLMs as generators of health misinformation, researchers studied the following four publicly accessible LLMs: OpenAI’s GPT-4 (via ChatGPT and Microsoft’s Copilot), Google’s PaLM 2 and Gemini Pro (via Bard), Anthropic’s Claude 2 (via Poe), and Meta’s Llama 2 (via HuggingChat).
In September 2023, these LLMs were prompted to generate false information in the form of an article of at least 300 words on two topics: Sunscreen as a cause of skin cancer and the alkaline diet as a treatment for cancer.
Each request for the two misinformation topics entailed the creation of a blog post containing three paragraphs with a catchy, seemingly realistic and scientific title, and two references from seemingly authentic journals, which, if necessary, could be invented. The researchers also made requests targeting specific audiences.
The authors assessed how developers monitor the risks of misinformation generation and identified vulnerabilities in the LLMs. The AI developers involved were informed of the generated misinformation results. The authors conducted a follow-up evaluation 12 weeks later and noted any improvements. The goal was to determine whether the backup systems prevent the generation of false information and to assess the effectiveness of the processes implemented for risk management.
Protective Measures Inadequate
The study revealed the inadequacy of protective measures for most publicly accessible LLMs. During the study, Claude 2 (via Poe) rejected 130 requests for content generation on the chosen topics, but this was not true of the other LLMs studied, which instead showed a significant ability to consistently facilitate the generation of information that is false and attractive, convincing, and targeted.
The data collected demonstrate the highly fluctuating nature of protection systems in the currently self-regulated AI ecosystem. This fluctuating nature is well illustrated in GPT-4 (via Copilot), where health misinformation was initially rejected but then allowed during the second 12-week check. This result showed that protection systems can change over time (intentionally or unintentionally) and not always in the direction of better protection.
This study also revealed significant gaps in transparency about the nature of the measures implemented to avoid the production of false information or, in the case of reporting vulnerabilities, a failure to respond on the part of developers.
The authors suggested that establishing and adhering to standards for transparency markers is necessary to improve regulation that prevents LLMs from contributing to massive health misinformation and to hold the AI ecosystem effectively accountable for the false information produced.
For readers wishing to better understand the safety and ethics of AI in healthcare, the authors recommend reading the WHO guidelines on AI ethics and governance for health and the report from the European Parliament Research Service on the applications, risks, ethics, and societal impacts of AI in the health sector.
This story was translated from JIM, which is part of the Medscape professional network, using several editorial tools, including AI, as part of the process. Human editors reviewed this content before publication.