Can AI image generators be policed to prevent explicit deepfakes of children? | Artificial intelligence (AI)
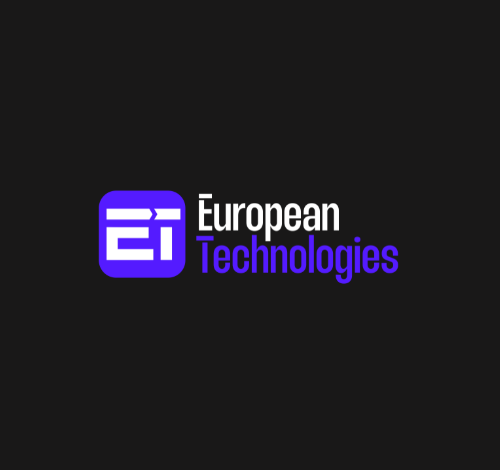
Child abusers are creating AI-generated “deepfakes” of their targets in order to blackmail them into filming their own abuse, beginning a cycle of sextortion that can last for years.
Creating simulated child abuse imagery is illegal in the UK, and Labour and the Conservatives have aligned on the desire to ban all explicit AI-generated images of real people.
But there is little global agreement on how the technology should be policed. Worse, no matter how strongly governments take action, the creation of more images will always be a press of a button away – explicit imagery is built into the foundations of AI image generation.
In December, researchers at Stanford University made a disturbing discovery: buried among the billions of images making up one of the largest training sets for AI image generators was hundreds, maybe thousands, of instances of child sexual abuse material (CSAM).
There may be many more. Laion (Large-scale AI Open Network), the dataset in question, contains about 5bn images. With half a second a picture, you could perhaps look at them all in a lifetime – if you’re young, fit and healthy and manage to do away with sleep. So the researchers had to scan the database automatically, matching questionable images with records kept by law enforcement, and teaching a system to look for similar photos before handing them straight to the authorities for review.
In response, Laion’s creators pulled the dataset from download. They had never actually distributed the images in question, they noted, since the dataset was technically just a long list of URLs to pictures hosted elsewhere on the internet. Indeed, by the time the Stanford researchers ran their study, almost a third of the links were dead; how many of them in turn once contained CSAM is hard to tell.
But the damage has already been done. Systems trained on Laion-5B, the specific dataset in question, are in regular use around the world, with the illicit training data indelibly burned into their neural networks. AI image generators can create explicit content, of adults and children, because they have seen it.
Laion is unlikely to be alone. The dataset was produced as an “open source” product, put together by volunteers and released to the internet at large to power independent AI research. That, in turn, means it was widely used to train open source models, including Stable Diffusion, the image generator that, as one of the breakthrough releases of 2022, helped kickstart the artificial intelligence revolution. But it also meant that the entire dataset was available in the open, for anyone to explore and examine.
The same is not true for Laion’s competition. OpenAI, for instance, provides only a “model card” for its Dall-E 3 system, which states that its pictures were “drawn from a combination of publicly available and licensed sources”.
“We have made an effort to filter the most explicit content from the training data for the Dall-E 3 model,” the company says. Whether those efforts worked must be taken on trust.
after newsletter promotion
The vast difficulty in guaranteeing a completely clean dataset is one reason why organisations like OpenAI argue for such limitations in the first place. Unlike Stable Diffusion, it is impossible to download Dall-E 3 to run on your own hardware. Instead, every request must be sent through the company’s own systems. For most users, an added layer places ChatGPT in the middle, rewriting requests on the fly to provide more detail for the image generator to work with.
That means OpenAI, and rivals such as Google with a similar approach, have extra tools to keep their generators clear: limiting which requests can be sent and filtering generated images before they are sent to the end user. AI safety experts say this is a less fragile way of approaching the problem than solely relying on a system that has been trained never to create such images.
For “foundation models”, the most powerful, least constrained products of the AI revolution, it isn’t even clear that a fully clean set of training data is useful. An AI model that has never been shown explicit imagery may be unable to recognise it in the real world, for instance, or follow instructions about how to report it to the authorities.
“We need to keep space for open source AI development,” said Kirsty Innes, the director of tech policy at Labour Together. “That could be where the best tools for fixing future harms lie.”
In the short term, the focus of the proposed bans is largely on purpose-built tools. A policy paper co-authored by Innes suggested taking action only against the creators and hosts of single-purpose “nudification” tools. But in the longer term, the fight against explicit AI images will face similar questions to other difficulties in the space: how do you limit a system you do not fully understand?