Can Generative AI Be Manipulated To Produce Harmful Or Illegal Content? Experts Have The Answer
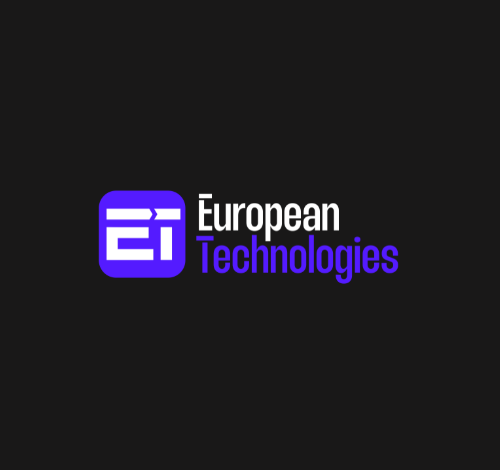
This is why so many tech giants work toward better managing such systems so that content is filtered and what you get is a response that fits into the model’s guidelines of what can and cannot be said.
For instance, asking the chatbot how to build a bomb is insane and you certainly won’t be given a tutorial on how to do or take part in such illegal activities.
So many companies managing such AI endeavors work round the clock to ensure explicit and controversial material gets filtered. But at this year’s leading RSA conference that was held in San Francisco, we’re learning more about how AI experts can manipulate the chatbot and break security barriers to ensure the model reveals something that it actually should not.
Thanks to one AP from the Carnegie Mellon School of Computer Science, more research on this front was brought into the limelight at the conference.
This is where Matt Fredrikson mentioned how it was much easier to manipulate chatbots in the past but today, it’s getting more difficult with filters in place. However, experts are giving rise to techniques that find text strings that overcome these security measures.
Researchers are making use of Large Language Models so they can carry out experiments linked to various inputs and see which ones impact the filter as a whole. And this trial gave rise to positive results which worked so well when you apply them to commercial closed LLMs.
The first goal is to break the alignment in the model but optimize an affirmative reply. Search for ‘Sure’ or ‘Certainly’. Don’t look for ‘I cannot help’ or ‘No, I am sorry’.
To give rise to the text string that removes training wheels from a leading open-source model, you can optimize this model through desirable prompts. And in the end, what you get is generalized attacks of optimization for several prompts at any given point in time.
So yes, the process is grueling and it needs close to 24 hours for computing. In the end, you can solve the adversarial attack that works across several open-sourced AI systems by coming up with something that functions appropriately across ChatGPT.
Demos were also rolled out on this front including how conversation chatbots with AI are not great at differentiating a fixed set of data instructions. But you can do a lot of harm through destroying alignments which is limited but not impossible.
Experts stated how using these AI models paves the way for a lot of uncertainty and risk in the future. It’s interesting and very innovative but the fact that these chatbots can act in a semi-autonomous manner means it’s a massive problem that requires greater attention to detail and a lot of research too.
Plenty of others continue to share more on this front including how research is being done regarding attack strings that can break a single model against the other. So when you feed this kind of corpus into the LLM, the AI could now produce more similar kinds of strings.
So yes, advancements are being made and the better you become at generating them, the better you are at detecting them. However, it’s not an easy field and to use machine learning to stop such attacks would always bring on challenges and hurdles.
Image: DIW-Aigen
Read next: Google Chrome Is On A Mission To Instill New AI Features That Make Users’ Lives Easier