Casting a Cybersecurity Net to Secure Generative AI in Manufacturing
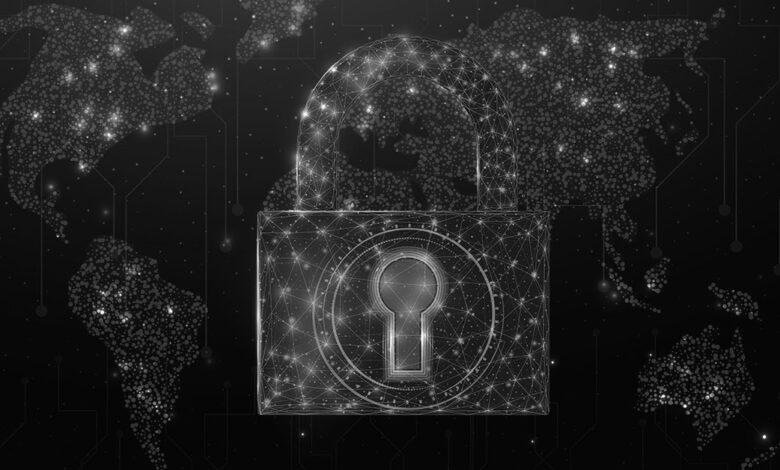
Generative AI has exploded in popularity across many industries. While this technology has many benefits, it also raises some unique cybersecurity concerns. Securing AI must be a top priority for organizations as they rush to implement these tools.
The use of generative AI in manufacturing poses particular challenges. Over one-third of manufacturers plan to invest in this technology, making it the industry’s fourth most common strategic business change. As that trend continues, manufacturers — often prime cybercrime targets — must ensure generative AI is secure enough before its risks outweigh its benefits.
Risks of Generative AI in Manufacturing
Securing generative AI in manufacturing starts with recognizing its risks. That can be an area of concern, as industrial sectors aren’t as experienced in cutting-edge tech. Consequently, they may be less likely to understand its potential dangers and overlook needed protections.
One of generative AI’s most significant cybersecurity threats is its vulnerability to data poisoning attacks. Attackers can manipulate the behavior of AI models, altering their training data by inserting misleading or false information or deleting essential parts of otherwise good information. This manipulation limits AI’s trustworthiness and efficacy, and organizations over-relying on AI may not catch it until it’s too late.
Because generative AI models require so much data, they may also make manufacturers bigger targets. Training AI on company information could leave lots of sensitive information in one place. These large, consolidated datasets could make it easier for cybercriminals to steal large amounts of high-value data.
Many use cases for generative AI in manufacturing also connect models to Internet of Things (IoT) data. Consequently, a compromised AI solution could let attackers control or disrupt IoT processes. That could lead to extensive physical damage and process delays.
It’s worth noting that AI also has many security advantages. It can lower data breach costs by 15% and shorten response times by 12% in many instances. Given these advantages, manufacturers can’t ignore AI entirely, but its security deserves special attention.
Securing AI in Manufacturing
Manufacturers must revamp their cybersecurity efforts to secure generative AI models. That begins with these best practices:
Encrypt All Data
The first step in securing AI in manufacturing is encrypting data. That applies to all IoT traffic within a facility and any information used to train generative AI models.
Encryption can be challenging in AI training datasets because AI models typically must decrypt information before using it. However, there are a few emerging solutions to this issue. Multiparty Computation (MPC) and Homomorphic Encryption (HME) let machine learning models use data without exposing it, though both technologies are still in their early stages.
Manufacturers may need to forgo conventional encryption methods anyway, as quantum computing poses new threats. Using quantum-resistant cryptography will ensure that data remains virtually useless to attackers even if there is a breach.
Restrict AI Access
Next, manufacturers must restrict access to AI models and training datasets. Thankfully, organizations are already taking access controls more seriously, with over 50% embracing zero-trust frameworks. Even if manufacturers haven’t implemented these restrictions in their larger workflows, they should apply them to AI.
The key is limiting privileges to the point where only people who must access AI models and information for their work can do so. The fewer people with this access, the fewer entry points attackers have for data poisoning.
It’s important to remember that access restrictions are only effective if they go hand in hand with strong authentication measures. Steps like multifactor authentication, biometrics, or cryptographic keys can provide the necessary assurance. Given the severity of these risks, simple username and password combinations aren’t enough.
Monitor AI Data
Generative AI in manufacturing also requires real-time monitoring. The industry has already made significant strides in this area. More attention around IoT risks led to a 458% increase in IoT security scans, and it’s time to apply the same care to AI models.
Continuous monitoring solutions can watch AI models and training databases to identify suspicious activity. The threshold for that suspicion could be anything from repeated access attempts from unauthorized accounts to unusual data transfers within the training dataset. Whatever the specifics, it’s essential to establish a baseline for normal behavior to recognize potential breaches more effectively.
This monitoring lets manufacturers spot AI attacks early. They can then respond to them and stop the attack before it causes too much damage.
Perform Regular Penetration Testing
Best practices for securing generative AI in manufacturing in the future may involve different steps than they do today. Threats evolve quickly, so cybersecurity measures must adapt just as rapidly. That adaptation requires regular penetration testing.
Pen testing is essential in any sector to reveal and address weak points before cybercriminals capitalize on them. Manufacturers face more pressure in this area than most, as they may be less familiar with cybersecurity concerns and measures. That knowledge gap is part of why manufacturing is the most-attacked industry, but penetration testing can close it.
Manufacturers should pen test their systems at least once a year, ideally more. Testing all areas of their networks is important, but if they must focus on one thing at a time, AI models and connected IoT devices deserve the most attention.
Use AI Carefully
Regardless of what other steps manufacturers follow, they must remember that AI is just a tool. Even if no cyberattacks occur, it can still be inaccurate. Consequently, they must not over-rely on it.
Human experts should always have the final say in business decisions. Using AI as a support tool, not the sole source of truth will help temper expectations around it. That’s important for preventing misuse and minimizing the dangers of attacks like data poisoning.
Always confirm AI insights before acting on them. Test models extensively before deploying them. Steps like this will reduce risks around compromised models and misleading data, with or without cybercrime.
Generative AI in Manufacturing Needs Better Security
Generative AI is a promising technology. At the same time, it can be dangerous if organizations aren’t careful.
Manufacturers must balance AI’s potential benefits with its risk to use it to its fullest potential. Following these best practices and taking a security-first approach to AI implementation will ensure that the industry enjoys the advantages of this promising technology without suffering from its shortcomings.
Editor’s Note: The opinions expressed in this guest author article are solely those of the contributor and do not necessarily reflect those of Tripwire.