Comcast, Roblox Put Generative AI to Work, but Other Orgs Struggle
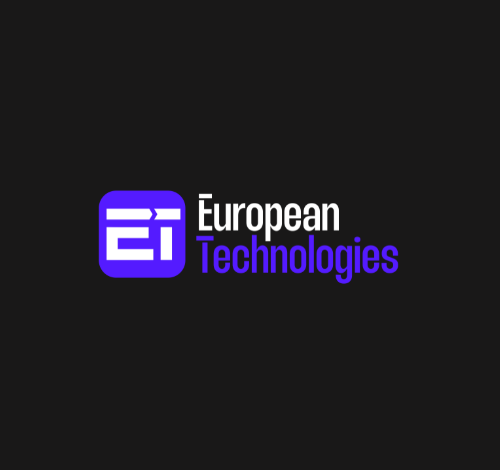
Comcast saw reductions in average time to handle calls and received positive feedback from agents about its generative AI proof of concept last year, according to Rama Mahajanam, who leads applied AI for customer experience at the media conglomerate. But there’s still work to be done, she said at the GenAI Productionize 2024 virtual event hosted by Galileo, a generative AI company.
This is the year enterprises will “productionize” generative AI, the panel agreed. In addition to Comcast, the panel included representatives from gaming platform Roblox and startup Enterprise Machine Assistant (EMA) Unlimited.
“Businesses I think are getting a little bit impatient about that so we need to deliver on the value, and 2024 is all about delivering value prompt GenAI,” Mahajanam said. “We are trying to apply RAG wherever any search-based approaches are required so I expect to see a lot more from that.”
RAG, or retrieval-augmented generation, is used to improve the accuracy and reliability of generative AI models.
Cross-Functional Teams Critical to AI Success
Comcast also has a dedicated AI technology group comprised of AI experts. But for any particular use case, organizations need a cross-functional team to work with the AI experiences team, Mahajanam said. That cross-functional team includes a product team that monitors metrics, she added. The cross-functional team also ensures that any experience is one that “you want to give to the customer,” she said.
“For every use case, you will have not just a modeler and a fine-tuner, but you will also have the annotators, you will also have the engineering lead, who is going to deploy the model, all of them working in tandem,” she said. “That’s absolutely crucial.”
The Comcast AI was deployed last year as part of a 90-day challenge in Comcast’s assistance platform.
“We saw some pretty phenomenal results, like pretty good reductions in average handle times and a lot of good feedback from agents,” Mahajanam said. “That being said, there are challenges when it comes to fine-tuning these large language models, and so we are trying to fine-tune the GPT 3.5, which is the one that we have access to, and also any open source models as well.”
Comcast AI Challenges
With GPT 3.5, Comcast saw high latency of responses, which can be a challenge with larger LLMs, Mahajanam said.
“If you look at the fine-tuned models that are the open source fine-tuned models, we do see that they are very quick and obviously cheaper to operate, but they can be very targeted,” she said. “But you do have to make sure that it is custom to your specific fine-tune task. So that’s one of the challenges.”
The other big challenge Comcast has observed is around evaluating responses, she added. The metrics used to evaluate natural language processing responses have fallen flat, so Comcast still relies primarily on humans evaluating responses right now, although it is actively exploring how to automate that, Mahajanam added.
“The biggest risk, as I see it, and especially if you are going ahead with a fine-tuned model, is hallucinations and making stuff up,” Mahajanam said. “We’re seeing that when we are doing this testing, as I said right now to go to production, even for employee trials, we are actually seeing the LLM making up stuff or assuming stuff, and this is a this is a huge problem. And that’s why you need humans annotating and making sure that you’re catching it when the LLM is making these assumptions.”
This despite implementing guardrails, such as making certain topics as taboo, she said. But AI is something of a machine learning (ML) “black box” right now, she added.
“The other key thing that we are seeing is how does the LLM handle out-of-domain queries,” she said. “Even when you’re using an open source model, you really don’t know all of the data that it was pre-trained on. So like all of the ML engineers on this call, we all know what data went into our models when you are training them right in the classical ML approach. But now you really don’t know what went into the pre-training of it, and so that makes the model very unpredictable.
Robox Upgrades Its First AIex
Roblox deployed a large model (now considered a small model) called Bert back in 2020. Today, Roblox supports a billion inference calls a day and is in the process of replacing Bert with a larger model, said Anupam Singh, an engineer at Roblox. Singh previously worked at Cloudera during the heydays of Hadoop.
“What we have seen is the problem/innovation opportunity now gets bigger, because the models are bigger,” said Singh. “For us, it is about replacing a bunch of infrastructure to support models that are 10 billion parameters or 70 billion parameters.”
Roblox has found that use cases that include a human in the loop are preferable to wholesale generative AI.
“Wherever humans are in the loop, we can be more aggressive,” said Singh “Explainability is going to be very important.”
Explainable AI is when the AI can justify or explain how it arrived at the information it offers.
The ROI of AI
Other organizations are still hoping to achieve as much as Roblox and Comcast, said Surojit Chatterjee. Chatterjee is the founder and CEO of EMA, but previously worked as the chief product officer at Coinbase and as a former product mobile ads lead at Google.
“Kudos to Rama, because most companies are not where Comcast probably is. Many companies are struggling to build GenAI applications that even work because they have seen the demos, the demos are very easy to build, you can build a demo in like three hours,” he said. “Then the actual application takes three quarters or more.”
Many organizations are struggling to implement and then scale GenAI applications, he said.
“In an enterprise mission-critical use case, it cannot be like 20%, inaccurate or 10% inaccurate,” he said. “The time of reckoning is coming almost right now. The time to follow seems to be much larger, longer than people originally anticipated.”
Successful companies are starting small. Rather than adding GenAI to everything, they’re looking at tangible use cases where they can deliver, measure and quantify the value, he added.
AI Demos and Lawyers
That led Galileo co-founder and panel host Yash Sheth to wonder how organizations can realize a return on investment quickly when generative AI can take multiple quarters to put something into production.
“The speed and power of building a demo with a large model is lightspeed, and so a lot of times your boards, your senior executives will see a demo. They’ll get excited, and they’ll say, ‘Wow, we are a month away from production,’” Singh said. “So some of our job as AI leaders is to put some level of practical guardrails around how to calculate ROI or how to make sure that it doesn’t hallucinate and put your company at risk.”
Chatterjee said the most successful customers look at what is time spent on a particular task and how much time is saved, then calculate the value of that time.
“Some of our customers actually have done that calculation, and they can say, ‘OK, I can reduce time spent by this particular role,’” he said. “What’s the compounded time saved over the year over, hundreds of people, thousands of people and so on? That’s a little bit of an exercise companies have to do task by task internally,”
And then there are the lawyers, who understandably worry about the potential for hallucinations or other bad actions on the part of generative AI. The legal team at Comcast allowed the IT team to put generative AI into production only with legal warnings saying the information had been generated with AI, said Mahajanam.
“Without that, you know, you really can’t go into production because of the unpredictability of the responses, so those are the key things that I see as big risks with using LLMs in production,” she said. “It’s like the Wild, Wild West — it can come back [with] whatever answer and so that is a big challenge.”
YOUTUBE.COM/THENEWSTACK
Tech moves fast, don’t miss an episode. Subscribe to our YouTube
channel to stream all our podcasts, interviews, demos, and more.