Common gen AI misconceptions for the enterprise
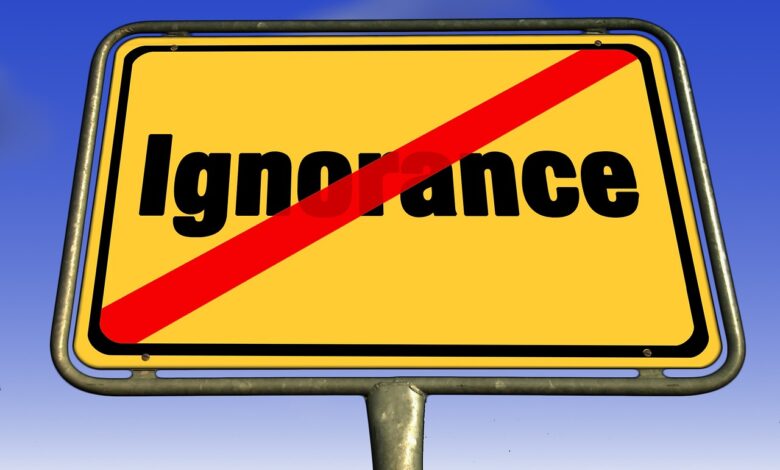
Generative AI has demonstrated an impressive ability to activate unstructured data to answer questions, write summaries, improve coding and enhance customer experience. These impressive capabilities are leading to many misconceptions about its potential, limitations, and the requirements for creating real business value. Here are misconceptions experts are seeing among enterprise users:
More data is always better
Large AI vendors have been in a hurry to hoover up as much data as possible to train more capable models. But Davi Ottenheimer, VP of Trust and Digital Ethics, Inrupt cautions that while data abundance can enhance model performance, it’s crucial to acknowledge that more data often means more noise or irrelevant information. He explains:
Without proper curation and validation mechanisms, accumulating large volumes of data can lead to technical debt and data cleanup challenges akin to those faced by hoarders who amass clutter. Thus, the focus should be on acquiring high-quality, relevant data rather than indiscriminately gathering vast quantities of information.
Ethical AI is a new endeavor.
Ottenheimer also often encounters the myth that ethical considerations in AI are a recent development, often tied to a specific year or technological advancement. However, ethical discussions in the context of artificial intelligence date back decades, with notable concerns emerging as early as the 1950s alongside the inception of AI as a field. Moreover, ethical deliberations about intelligence and its implications trace back even further, with philosophical debates spanning centuries. Ottenheimer argues:
Recognizing this historical context is essential for understanding the complexity of ethical challenges in AI and the need for ongoing interdisciplinary dialogue to address them effectively.
Gen AI will replace most jobs
Every week, a new survey or analysis claims that gen AI will increase a growing percentage of jobs. Bharath Thota, Partner and Chief Analytics Solutions Officer at Kearney, says:
AI isn’t going to take all our jobs. It automates some tasks, but most jobs will change and grow with AI’s help, not disappear. AI takes on the routine stuff, so we can do the more interesting, creative work.
There is no cost for gen AI
The growing availability of generative AI foundation models is leading to the myth that these can be consumed by the enterprise at little incremental cost. Rohit Kedia, Chief Growth Officer, LTIMindtree, a global tech consultancy, clarifies:
Implementing gen AI models demands substantial computational power for training and inference, straining infrastructure budgets and workflows. Creating scalable, enterprise-grade solutions entails a hefty expense and requires secure implementation for specific contexts.
Gen AI is a quick fix for infrastructure and process gaps
Many consultancies are also confronting the myth that AI is a quick fix to solve poor processes or infrastructure that can be applied to every problem. Thota says:
Generative AI isn’t a quick fix. It’s powerful but needs careful setup, good data, and regular updates to work well. It’s not a one-stop solution or an instant result maker.
AI can magically know the answer
Babak Hodjat, CTO of AI at Cognizant, observes that many enterprises are scratching the surface of what gen AI can do, such as improving software development and customer and employee productivity. He is also running into a false assumption around the idea that you can ask a gen AI model a question regarding your business and expect it to magically know the answer even if you don’t facilitate its access to relevant data or an API to help it reason its way to a more reliable response. He says:
This kind of output is just not possible yet.
AI is sentient
Nils Olsson, the Chief Strategy Officer at Tacton, a configure, price, quote platform, notes that many new technologies are initially viewed as able to solve every problem. In reality, it’s one type of tool that does certain kinds of tasks very well, and that will continue to be the case, but that doesn’t mean it will solve every type of problem. He says:
In the case of gen AI, it’s an application that generates an answer, based on your question, that it deems you’ll find acceptable — it’s not sentient, even if it appears to be.
AI is a panacea
Nick Kramer, VP of Applied Solutions at SSA & Company, sees many executives getting enchanted by the broad utility of gen AI, meaning that it can be incorporated into existing products in ways blockchain, Robotic Process Automation, and other previously hyped technologies could not. This is where it will provide the most value. This has led many executives and their boards to buy into promises that it is a panacea. Kramer says:
Unfortunately, there are a lot of areas in which our existing analytical methods are vastly superior. People believe its quantitative outputs even though math is not their strongest suit. They cannot escape creating hallucinations, and its creations are only a starting point, not a finished product.
Focus on use cases
A variety of new enterprise tools use gen AI to improve specific tasks like coding or content development. But Sanz Sáiz, data and AI Leader at EY Global Consulting says this risks missing out on larger opportunities. He explains:
Use cases, when taken in isolation, can commoditize AI, and CEOs end up lost in a sea of individual use cases that miss the potential impact and benefits that AI can bring to an entire company and its people. A better strategy is to prioritize their workforce in relation to gen AI. This approach is not just about enhancing people’s adaptability but also about equipping them for change, fostering a sense of trust, promoting acceptance, and preparing the workforce for the future.
It can replace traditional analytics
The value of gen AI lies in improving the use of unstructured data like text, audio, and video and is not a replacement for traditional data analytics tooling. Christine Livingston, global leader of Artificial Intelligence services at Protiviti, a digital transformation consultancy, observes:
Many organizations are approaching generative AI as one-size-fits-all AI and attempting to perform tasks heavily dependent on structured data analysis such as payment information or asset performance with generative AI models. Although generative AI models are improving on traditional analytics capabilities, they were not designed for, nor should they be positioned for structured data analysis. These problems should be solved with more conventional analytics and AI-based approaches.
Underestimating what gen AI can soon do
Donncha Carroll, Partner and Chief Data Scientist at Lotis Blue Consulting believes that many enterprises are underestimating the pace of gen AI development. The speed at which these tools (e.g., Llama, Gemini, GPT) and their surrounding ecosystem (e.g., LangChain, Hugging Face, MongoDB, Pinecone) are evolving makes it challenging for organizations to assess and manage both capability and enterprise risk. Valid concerns around hallucinations and data privacy are constraints, but are getting addressed quickly. These solutions need to be viewed as a component of overall work processes that still require human oversight and augmentation rather than as standalone technology. Carroll notes:
It’s only 18 months ago since ChatGPT hit the mainstream, and there are thousands of emerging companies that provide infrastructure to take advantage of these models. I agree with Roy Amara, a Stanford computer scientist from the 1960s, in that, “We overestimate the impact of technology in the short-term and underestimate the effect in the long run.”
My take
Generative AI promises fascinating advances in how we build enterprise systems and create value. Some executives may be tempted to over-invest in immature tools, replace staff with bots, or trust increasingly prosaic hallucinations.
In most industries, the biggest returns may come from investing in building out the culture, infrastructure, and processes that can take advantage of gen AI when the tools and best practices mature.