Cybersecurity With Human-AI Collaboration – USC Viterbi
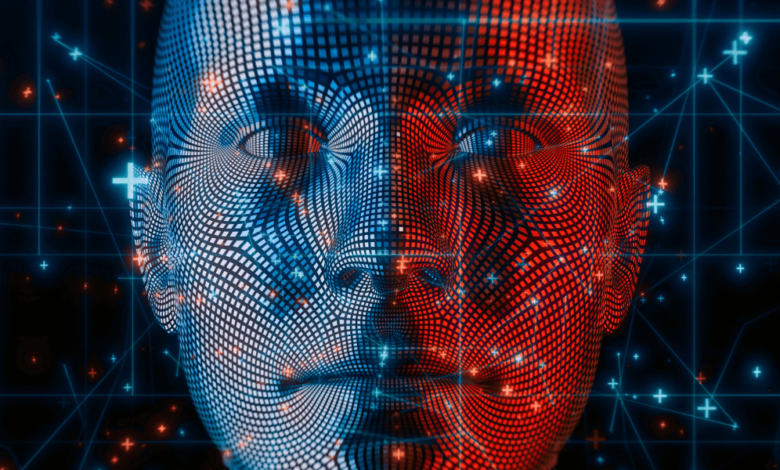
ILLUSTRATION BY DEM10/ISTOCK
As organizations increasingly rely on networks, online platforms, data and technology, the risks associated with data breaches and privacy violations are more severe than ever. Couple this with the escalating frequency and sophistication of cyber threats and it becomes clear that fortifying cybersecurity defenses has never been more important. Cybersecurity analysts are on the front lines of this battle, working around the clock in security operations centers (SOCs) – the units that safeguard organizations from cyber threats – to sift through a massive volume of data as they monitor potential security incidents.
They are faced with vast streams of information from disparate sources, ranging from network logs to threat intelligence feeds, trying to prevent the next attack. In short, they are overwhelmed. But too much data has never been a problem for artificial intelligence, so many experts are looking to AI to bolster cybersecurity strategies and ease the strain on analysts.
Stephen Schwab, director of Strategy for USC’s Information Sciences Institute’s (ISI) Networking and Cybersecurity Division, envisions symbiotic teams of humans and AIs collaborating to improve security, so that AI can assist analysts and improve their overall performance in these high-stakes environments. Schwab and his team have developed testbeds and models to research AI-assisted cybersecurity strategies in smaller systems, such as protecting a social network. “We’re trying to ensure that machine learning processes can ease, but not add to, these worries and lighten the human analyst’s workload,” he said.
David Balenson, associate director of ISI’s Networking and Cybersecurity division, emphasizes the critical role of automation in alleviating the burden on cybersecurity analysts. “SOCs are flooded with alerts that analysts have to analyze rapidly in real time, and decide which are symptoms of a real incident. That’s where AI and automation come into play, spotting trends or patterns of alerts that could be potential incidents,” says Balenson.
Looking for Transparency and Explainability
However, the integration of AI into cybersecurity operations is not without its challenges. One of the primary concerns is the lack of transparency and explainability inherent in many AI-driven systems. “Machine learning (ML) is useful for monitoring networks and end-systems where human analysts are fatigued,” Schwab explains. “Yet they are a black box – they can throw off alerts that may seem inexplicable.
This is where explainability comes in, as the human analyst has to trust that the ML system is operating within reason.” A solution that Schwab proposes is building explainers that present the ML system’s actions in computerized English, similar to natural language, that the analyst can understand. Marjorie Freedman, a Principal Scientist at ISI is researching this. “I’ve been looking at what it means to generate explanations, and what you want from the explanation. We are also exploring how an explanation can help a person verify a model’s generation,” she said.
The Art of Flagging
One example of an explanation for an AI decision in cybersecurity is the process of online authentication. When authenticating to a system, users type in a password or PIN code. However, different people punch in the data in different patterns, which the AI might flag even if the code was correctly entered.
These “potentially suspicious” patterns might not actually be security breaches, but the AI still factors them into consideration. If, along with flagging them, an explainer is provided to the human analyst listing the input pattern as one of the reasons for the flagging, the analyst will better understand the reasoning behind the AI’s decision-making. And armed with that additional information, the analyst can make more informed decisions and take appropriate action (i.e., validate or override the AI’s determination). Freedman believes that cybersecurity operations should run their best ML model to predict, identify, and address threats in parallel with approaches that effectively explain the decision to experts.
“If somebody is shutting down a system that will cost the company a lot of money, it’s a high-stakes situation where we must confirm it’s the right decision,” said Freedman. “The explainer may not exactly be the AI’s derivation of how it got there, but it might be what the human analyst needs to know to determine whether it’s correct or not.”
Keeping the Data Safe and Private
While trust between the human analyst and the machine is one challenge with AI in cybersecurity, trust that the sensitive or proprietary information that AIs are trained on will remain private is another.” For example, to train a machine learning model to keep data safe or protect systems, an organization might use operational details or security vulnerabilities.
The potential exposure of this type of sensitive information about an organization’s cyber posture is a concern when integrating AI into cybersecurity operations. “Once you’ve put information into systems like large language models, even if you try to remove it, there’s no guarantee that you’ve been successful in preventing it from discussing that information. We need to search for ways to make that sharing space safe for all,” said Schwab.
Schwab, Freedman and the ISI team hope their work will lead to new ways to harness the strengths of both humans and AI to bolster cyberdefenses, stay ahead of sophisticated adversaries, and alleviate the overwhelm in the SOCs.
Published on May 29th, 2024
Last updated on May 29th, 2024