Data Analytics – Do a Better Deal
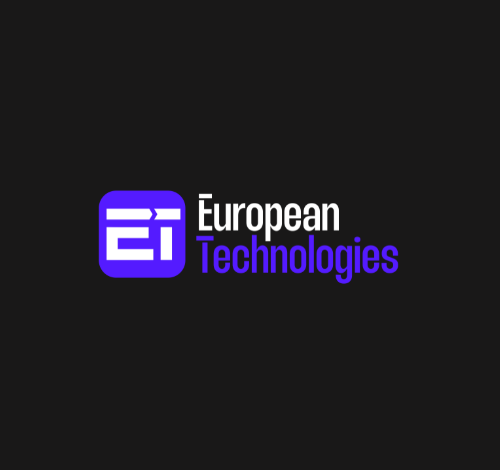
If you have been involved in mergers and acquisitions (M&A) transactions, even on the periphery, you have likely heard the term data analytics. Or, perhaps you have heard discussion regarding a data cube. But unless you are a data junkie (admittedly, I work with several of those!), you may not have explored these further or taken time to understand exactly what was meant by these terms. This article will examine the background of data analytics and a case study of the value it provides.
Data analytics utilizes client and publicly available data sets to create analysis-ready databases or standalone analytics/outputs that facilitate quicker and more supported decision making. A variety of processing tools are used to achieve these objectives, including Alteryx, Tableau, and SQL databases.
What roadblocks have you experienced during a potential M&A transaction? Perhaps, as a buyer, you have struggled to confirm your investment thesis or understand how or where the seller makes money. Or, as a seller, you received requests that seemed cumbersome due to file sizes, system limitations, or even requests that you would like to fulfill but had to tell the buyer, “We cannot get you that data.” Data analytics is designed to help solve these problems, and along the way results in outputs that buyers and sellers can use to help facilitate a transaction, including:
- Price/Volume Analysis
- Employee Utilization
- Customer Churn & Retention/Annual Recurring Revenue
- Project Estimating Accuracy
- Payor Reimbursement Trends
- Commodity Pricing
- Margins by Product/Customer
So, what does this look like in the real world? On a recent transaction in the plastic products manufacturing industry, the target experienced commodity cost changes that were not passed through to customers, creating fluctuations in material margins. The target was able to provide standard gross margin by stock-keeping unit (SKU) but not material margin. The target had been experiencing a shift in product mix category, with its primary category having the highest material margin. As a result, changes in material margins present in the financial statements could not be isolated to changes from commodity costs versus changes from product category mix.
Given the data challenges, our professionals obtained the bill of materials by SKU and annual raw materials cost to develop material costs by SKU from scratch. This was then applied to the invoicing register, allowing us to quantify the impact of movements in material margin from commodity costs versus product category mix (at the SKU level). Ultimately, the buyer was able to use this analysis to evaluate the business under various potential commodity cost scenarios.
In summary, data analytics assists buyers and sellers by reducing surprises and identifying and synthesizing issues early, potentially leading to increased buyer confidence and seller credibility. It allows for an objective analysis of the business, which may lead to an acceleration of transaction timeline. Finally, data analytics may assist sellers with increased awareness of critical deal components and decision points. If you have struggled articulating your business to buyers (or understanding the financials of a seller beyond a basic income statement or balance sheet), data analytics may be the key to providing deeper insights and bridging the gap between buyer and seller expectations.
If you have any questions or need assistance, please reach out to one of our professionals.