Data Science and Effective Business Analytics in Route Planning
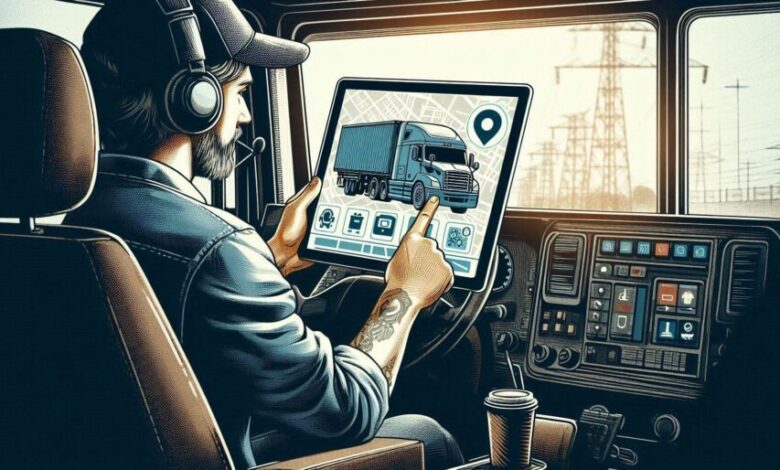
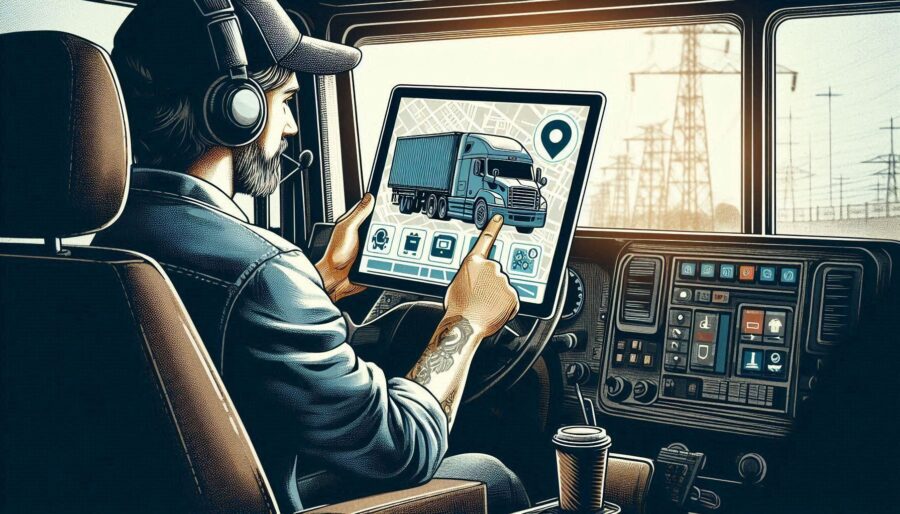
There’s effectively no area of modern business in which data science and analytics do not have some form of influence, and logistics is an example where they matter more than in most contexts.
In particular, it’s the application of these overlapping specialisms to the process of route planning that holds the most relevance for organizations operating today, so here’s a look at what aspects are most in the spotlight right now, and why they matter.
Advanced machine learning algorithms in route optimization
It’s no surprise that we’re seeing the widespread implementation of machine learning (ML) in route planning, thanks to its ability to offer up more precise and efficient solutions than were achievable via manually programmed methods.
Key innovations include:
- Reinforcement learning: Utilizes trial-and-error methods for continuous improvement in route decisions.
- Neural networks: Process complex datasets like historical traffic flows, weather conditions, and road closures to find the best routes.
- Clustering techniques: Group similar routes together for efficiency gains in delivery or transit systems.
Practical applications
This tech is already being put to work by all sorts of businesses, for the obvious reason that it both improves efficiency and saves money – to the tune of $50 million annually in some cases. Its abilities factor in the likes of:
- Dynamic Routing: Adjusts paths on the fly based on current traffic situations.
- Predictive Maintenance: Identifies potential vehicle failures before they happen, minimizing downtime.
- Fuel Efficiency Optimization: Select routes that save fuel costs by avoiding congested areas or steep inclines.
These are the kinds of benefits that can of course be achieved by hand using widely available tools. For instance, you can optimize your route with Google Maps by adding stops and picking particular routes based on local knowledge. However, ML makes it swifter, slicker, and more business-focused.
Integrating IoT and sensor data into logistics planning
The integration of Internet of Things (IoT) devices and sensor data has turned logistics planning on its head. Collecting real-time information from various sources means companies can make informed decisions to optimize their routes and operations.
The key benefits here include:
- Real-time tracking: Monitors vehicle locations, traffic conditions, and delivery statuses continuously.
- Environmental monitoring: Sensors detect weather changes, road hazards, or unexpected delays.
- Vehicle diagnostics: Collects data on engine performance, fuel levels, and maintenance needs. Since the cost of fleet disruption due to vehicle downtime can spiral into thousands of dollars for a single day, this has far-reaching financial consequences.
Practical implementations
In terms of actually putting IoT sensors and the data they generate to work, there are a few main examples you need to know about:
- Smart fleet management: Uses GPS tracking combined with sensor data to optimize fleet routes dynamically.
- Condition-based maintenance: Schedules repairs based on actual wear-and-tear instead of regular intervals.
- Enhanced safety protocols: Alerts drivers about hazardous conditions in real-time to prevent accidents.
These advancements enable logistics managers to respond proactively rather than reactively. It also applies across the supply chain, such as in the case of FedEx using IoT hardware and software to provide comprehensive visibility into its operations for customers in industries where this matters most, such as healthcare.
Autonomous vehicles and AI-driven routing decisions
Autonomous vehicles (AVs) combined with artificial intelligence (AI) are another player in the game of revamped route planning that’s ongoing worldwide as we speak. These technologies promise unprecedented efficiency, safety, and adaptability in logistics.
Some of the key advantages here are:
- Precision navigation: AVs use AI to navigate complex routes accurately.
- Continuous operation: Operate around the clock without fatigue or human error.
- Adaptive learning: As mentioned in regard to ML earlier, AI systems can also improve their decision-making over time based on accumulated data.
Practical applications
We’re still a long way from seeing AVs being the dominant force in global transport, and the market is only worth around $1.9 billion at the moment, but the future looks bright for the following reasons:
- Last-mile delivery automation: Self-driving delivery vans optimize the final leg of deliveries, reducing costs and increasing speed.
- Real-time route adjustments: AVs receive live updates on traffic conditions, road closures, or accidents to choose optimal paths instantly.
- Platooning techniques: Multiple AVs travel in close proximity to reduce air drag and save fuel.
Once the kinks in this technology have been ironed out, it should not be long before the dream of truly autonomous delivery becomes a reality, and companies along with their customers reap the benefits.
Wrapping up
In short, route planning is an example of the overarching influence of data science and business analytics on commercial operations in the 21st century.
Businesses have more data than they know what to do with, so it makes sense to squeeze value from it in novel ways, whether that’s machine learning applied to route optimization or IoT for vehicle monitoring and maintenance.