Don’t believe the hype about AI’s impact on productivity
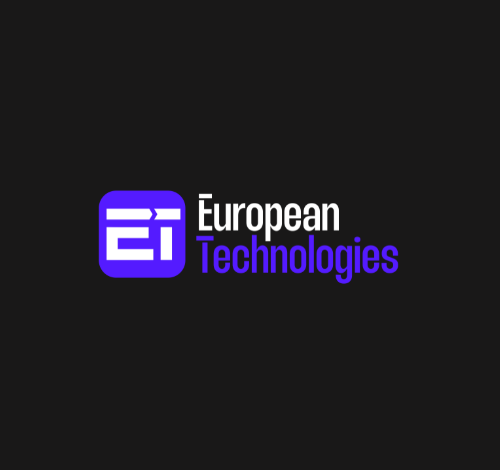
Taken together, this research suggests that currently available generative-AI tools yield average labour-cost savings of 27 per cent and overall cost savings of 14.4 per cent.
There is little to support the argument we should not worry about regulation, because AI will be the rising tide that lifts all boats.
What about the share of tasks that will be affected by AI and related technologies? Using numbers from recent studies, I estimate this to be around 4.6 per cent, implying that AI will increase TFP by only 0.66 per cent over 10 years, or by 0.06 per cent annually. Of course, since AI will also drive an investment boom, the increase in GDP growth could be a little larger, perhaps in the 1-1.5 per cent range.
These figures are much smaller than the ones from Goldman Sachs and McKinsey. If you want to get those bigger numbers, you either must boost the productivity gains at the micro level or assume that many more tasks in the economy will be affected.
But neither scenario seems plausible. Labour-cost savings far above 27 per cent not only fall out of the range offered by existing studies; they also do not align with the observed effects of other, even more promising technologies. For example, industrial robots have transformed some manufacturing sectors, and they appear to have reduced labour costs by about 30 per cent.
Similarly, we are unlikely to see far more than 4.6 per cent of tasks being taken over, because AI is nowhere close to being able to perform most manual or social tasks (including seemingly simple functions with some social aspects, like accounting).
As of 2019, a survey of essentially all US businesses found that only about 1.5 per cent of them had any AI investments. Even if such investments have picked up over the past year and a half, we have a long, long way to go before AI becomes widespread.
Bigger impact on women
Of course, AI could have larger effects than my analysis allows if it revolutionises the process of scientific discovery or creates many new tasks and products. The recent AI-enabled discoveries of new crystal structures and advances in protein folding do suggest such possibilities. But these breakthroughs are unlikely to be a major source of economic growth within 10 years.
Even if new discoveries could be tested and turned into actual products much faster, the tech industry is currently focused excessively on automation and monetising data, rather than on introducing new production tasks for workers.
Moreover, my own estimates could be too high. Early adoption of generative AI has naturally occurred where it performs reasonably well, meaning tasks for which there are objective measures of success, such as writing simple programming subroutines or verifying information. Here, the model can learn on the basis of outside information and readily available historical data.
But many of the 4.6 per cent of tasks that could feasibly be automated within 10 years – evaluating applications, diagnosing health problems, providing financial advice – do not have such clearly defined objective measures of success, and often involve complex context-dependent variables (what is good for one patient will not be right for another). In these cases, learning from outside observation is much harder, and generative AI models must rely instead on the behaviour of existing workers.
Under these circumstances, there will be less room for major improvements over human labour. Thus, I estimate that about one-quarter of the 4.6 per cent tasks are of the “harder-to-learn” category and will have lower productivity gains. Once this adjustment is made, the 0.66 per cent TFP growth figure declines to about 0.53 per cent.
What about the effects on workers, wages, and inequality? The good news is that, compared with earlier waves of automation – such as those based on robots or software systems – the effects of AI may be more broadly distributed across demographic groups.
If so, it will not have as extensive an impact on inequality as earlier automation technologies did (I estimated these effects in my previous work with Pascual Restrepo). However, I find no evidence that AI will reduce inequality or boost wage growth. Some groups – especially white, native-born women – are significantly more exposed and will be negatively affected, and capital will gain more than labour overall.
Economic theory and the available data justify a more modest, realistic outlook for AI. There is little to support the argument that we should not worry about regulation, because AI will be the proverbial rising tide that lifts all boats. AI is what economists call a general-purpose technology. We can do many things with it, and there are certainly better things to do than automate work and boost the profitability of digital advertising. But if we embrace techno-optimism uncritically or let the tech industry set the agenda, much of the potential could be squandered.
Daron Acemoglu is Institute Professor of Economics at MIT, and a co-author (with Simon Johnson) of Power and Progress: Our Thousand-Year Struggle Over Technology and Prosperity.
Have your say
- We are always interested to hear your views on current topics.
- Guidelines for how to write an opinion article are here.
- Guidelines for how to write a letter to the editor are here. Please send your letter to edletters@afr.com.au